3DSN-Net: A 3-D Scale-Aware convNet With Nonlocal Context Guidance for Kidney and Tumor Segmentation From CT Volumes.
IEEE transactions on cybernetics(2023)
摘要
Automatic kidney and tumor segmentation from CT volumes is a critical prerequisite/tool for diagnosis and surgical treatment (such as partial nephrectomy). However, it remains a particularly challenging issue as kidneys and tumors often exhibit large-scale variations, irregular shapes, and blurring boundaries. We propose a novel 3-D network to comprehensively tackle these problems; we call it 3DSN-Net. Compared with existing solutions, it has two compelling characteristics. First, with a new scale-aware feature extraction (SAFE) module, the proposed 3DSN-Net is capable of adaptively selecting appropriate receptive fields according to the sizes of targets instead of indiscriminately enlarging them, which is particularly essential for improving the segmentation accuracy of the tumor with large scale variation. Second, we propose a novel yet efficient nonlocal context guidance (NCG) mechanism to capture global dependencies to tackle irregular shapes and blurring boundaries of kidneys and tumors. Instead of directly harnessing a 3-D NCG mechanism, which makes the number of parameters exponentially increase and hence the network difficult to be trained under limited training data, we develop a 2.5D NCG mechanism based on projections of feature cubes, which achieves a tradeoff between segmentation accuracy and network complexity. We extensively evaluate the proposed 3DSN-Net on the famous KiTS dataset with many challenging kidney and tumor cases. Experimental results demonstrate our solution consistently outperforms state-of-the-art 3-D networks after being equipped with scale aware and NCG mechanisms, particularly for tumor segmentation.
更多查看译文
关键词
3-D convolutional neural networks (CNNs),kidney and tumor segmentation,nonlocal context guidance (NCG),scale-aware feature extraction (SAFE)
AI 理解论文
溯源树
样例
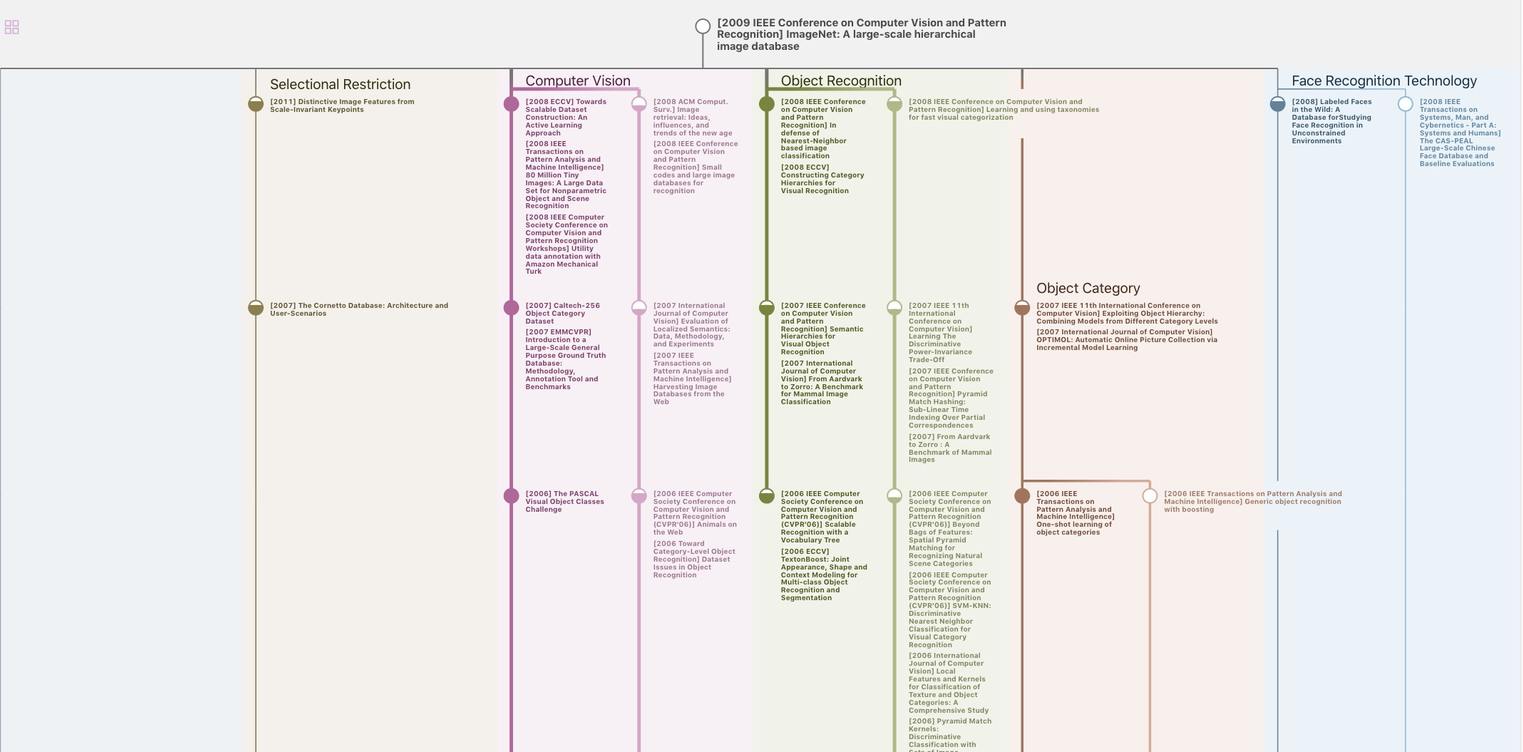
生成溯源树,研究论文发展脉络
Chat Paper
正在生成论文摘要