FedDRL: Trustworthy Federated Learning Model Fusion Method Based on Staged Reinforcement Learning
COMPUTING AND INFORMATICS(2024)
摘要
Traditional federated learning uses the number of samples to calculate theweights of each client model and uses this fixed weight value to fusion theglobal model. However, in practical scenarios, each client's device and dataheterogeneity leads to differences in the quality of each client's model. Thusthe contribution to the global model is not wholly determined by the samplesize. In addition, if clients intentionally upload low-quality or maliciousmodels, using these models for aggregation will lead to a severe decrease inglobal model accuracy. Traditional federated learning algorithms do not addressthese issues. To solve this probelm, we propose FedDRL, a model fusion approachusing reinforcement learning based on a two staged approach. In the firststage, Our method could filter out malicious models and selects trusted clientmodels to participate in the model fusion. In the second stage, the FedDRLalgorithm adaptively adjusts the weights of the trusted client models andaggregates the optimal global model. We also define five model fusion scenariosand compare our method with two baseline algorithms in those scenarios. Theexperimental results show that our algorithm has higher reliability than otheralgorithms while maintaining accuracy.
更多查看译文
关键词
Federated learning,model fusion,model attack,reinforcement learning
AI 理解论文
溯源树
样例
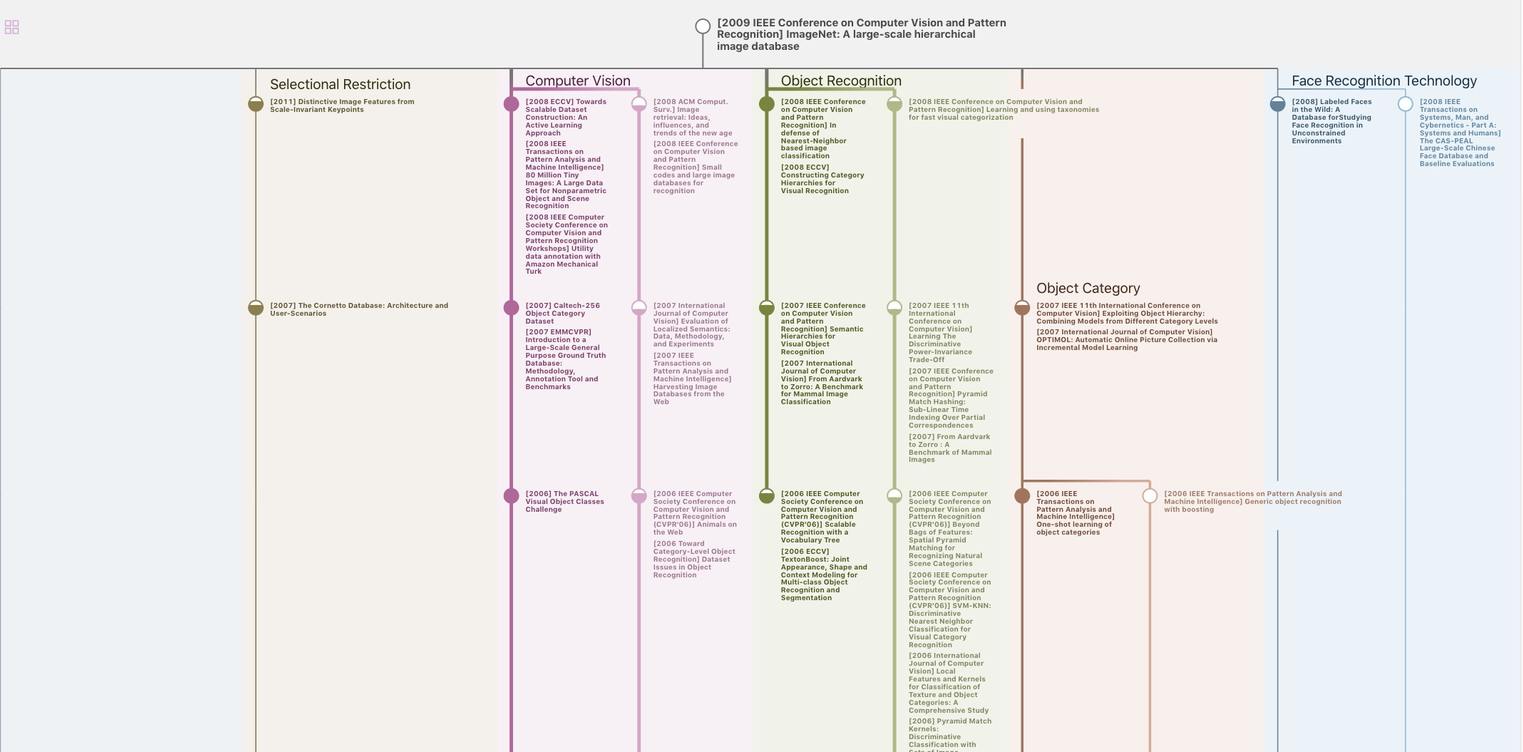
生成溯源树,研究论文发展脉络
Chat Paper
正在生成论文摘要