Shape description losses for medical image segmentation
Mach. Vis. Appl.(2023)
摘要
Despite the success of deep learning in medical image segmentation, most deep learning-based segmentation methods utilize pixel-wise losses as their training objectives, which focus on the local information around each pixel but lack global context. In this paper, we propose a set of shape description losses to supervise the training of the segmentation networks by extracting and quantifying geometric features of the targets, including volume, surface area, center of mass, and bounding box. We believe that these loss functions can introduce global geometrical and statistical constraints into the training procedure. The proposed shape losses are differentiable and can be computed efficiently. We demonstrate that using the proposed shape losses alone for weakly supervised learning can obtain promising performance. The results of our study illustrate the effectiveness of the shape descriptions with physical meanings on segmenting different organs from various modalities, including liver from computed tomography, left atrium from magnetic resonance imaging, and prostate from ultrasound volume.
更多查看译文
关键词
Deep learning, Medical image segmentation, Loss function, Weakly supervised segmentation, Shape analysis, Radiomics features
AI 理解论文
溯源树
样例
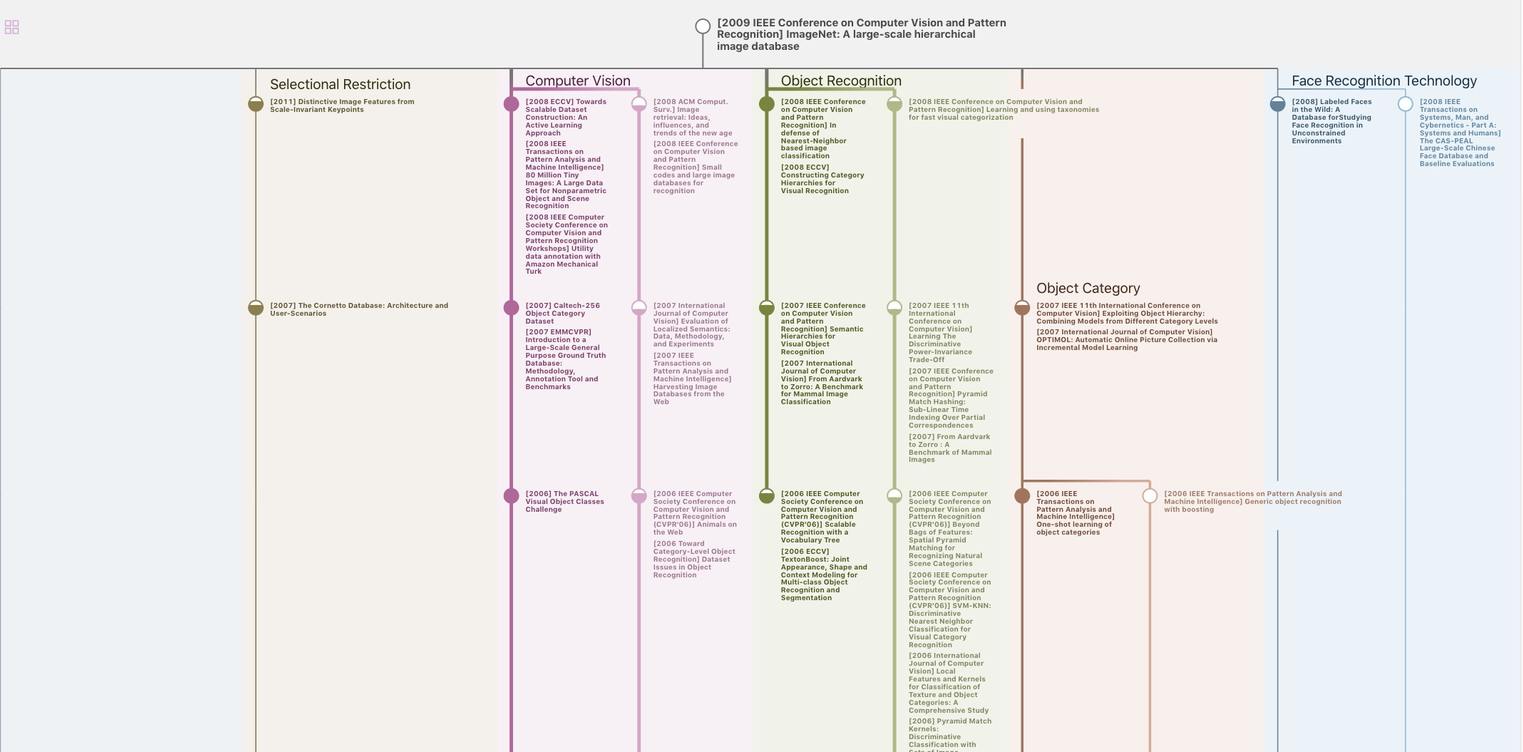
生成溯源树,研究论文发展脉络
Chat Paper
正在生成论文摘要