Attacking Memristor-Mapped Graph Neural Network by Inducing Slow-to-Write Errors.
ETS(2023)
摘要
Graph neural networks (GNNs) are becoming popular in various real-world applications. However, hardware-level security is a concern when GNN models are mapped to emerging neuromorphic technologies such as memristor-based crossbars. These security issues can lead to malfunction of memristor-mapped GNNs. We identify a vulnerability of memristor-mapped GNNs and propose an attack mechanism based on the identified vulnerability. The proposed attack tampers memristor-mapped graph-structured data of a GNN by injecting adversarial edges to the graph and inducing slow-to-write errors in crossbars. We show that 10% adversarial edge injection induces 1.11× longer write latency, eventually leading to a 44.33% error in node classification. Experimental results for the proposed attack also show that there is a 5.72× increase in the success rate compared to a software-based baseline.
更多查看译文
关键词
10% adversarial edge injection,attack mechanism,attack tampers memristor-mapped graph-structured data,attacking memristor-mapped graph neural network,GNN models,graph neural networks,hardware-level security,identified vulnerability,inducing slow-to-write errors,memristor-based crossbars,memristor-mapped GNNs,security issues
AI 理解论文
溯源树
样例
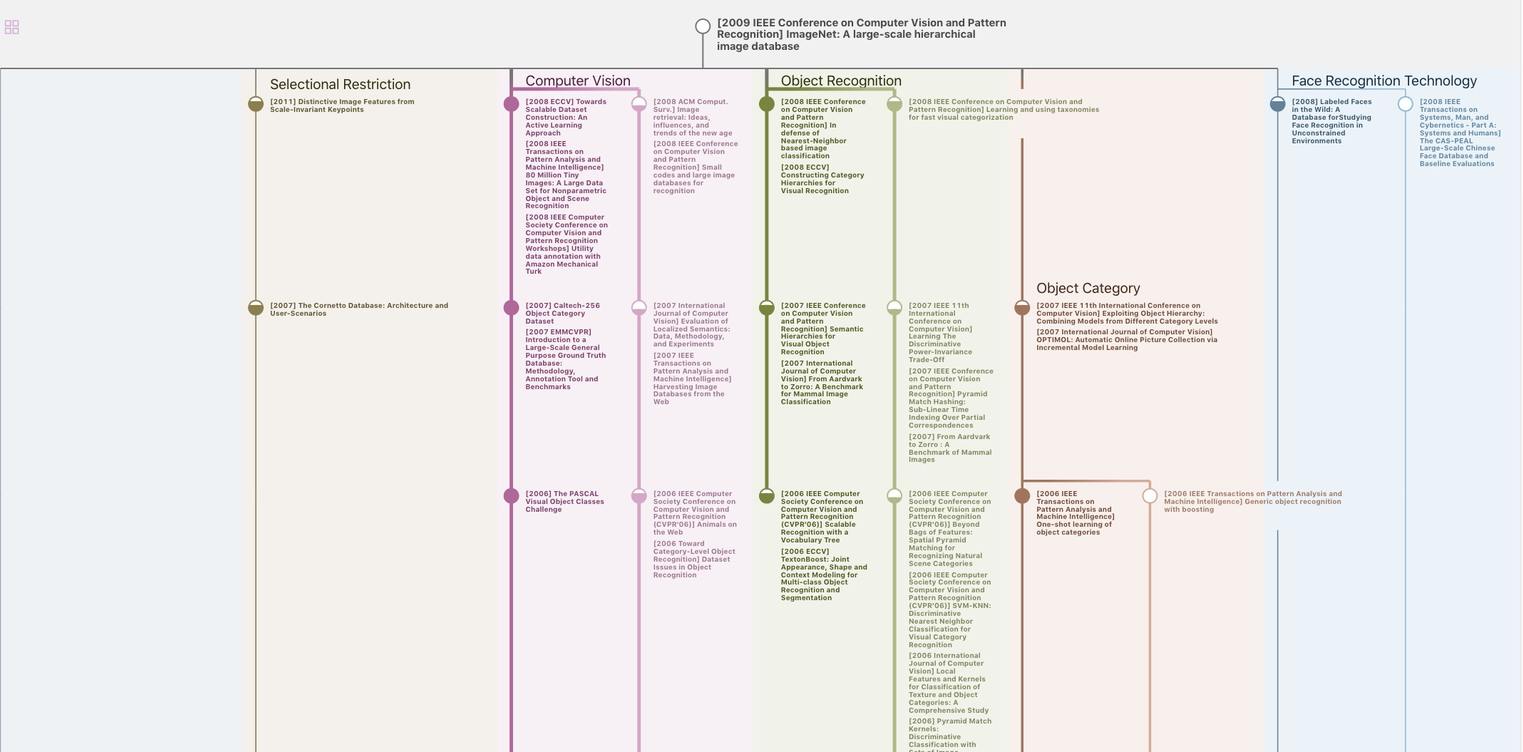
生成溯源树,研究论文发展脉络
Chat Paper
正在生成论文摘要