Multi-level Subgraph Representation Learning for Drug-Disease Association Prediction Over Heterogeneous Biological Information Network.
ICIC (3)(2023)
Abstract
Identifying new indications for existing drugs is a crucial role in drug research and development. Computational-based methods are normally regarded as an effective way to infer drugs with new indications. They, though effective, normally fall short of capturing semantic higher-order connectivity patterns presented in heterogeneous biological information networks (HBINs) when learning the respective embeddings of drugs and diseases. To overcome this problem, we propose a novel Multi-level Subgraph Representation Learning model, namely MSRLDDA, for drug-disease association (DDA) prediction. In particular, MSRLDDA first defines different meta-paths to construct semantic subgraphs such that the mechanisms of how drugs act on diseases can be revealed. For each subgraph, a particular graph neural network model is adopted to conduct the representation learning process from different perspectives. By doing so, more expressive representations of drugs and diseases are obtained at multi-level. Experimental results on two benchmark datasets demonstrate that MSRLDDA performs better than several state-of-the-art drug repositioning models. This is a strong indicator that the consideration of higher-order connectivity patterns gains new insight into DDA prediction with improved accuracy.
MoreTranslated text
Key words
heterogeneous biological information network,information network,association,multi-level,drug-disease
AI Read Science
Must-Reading Tree
Example
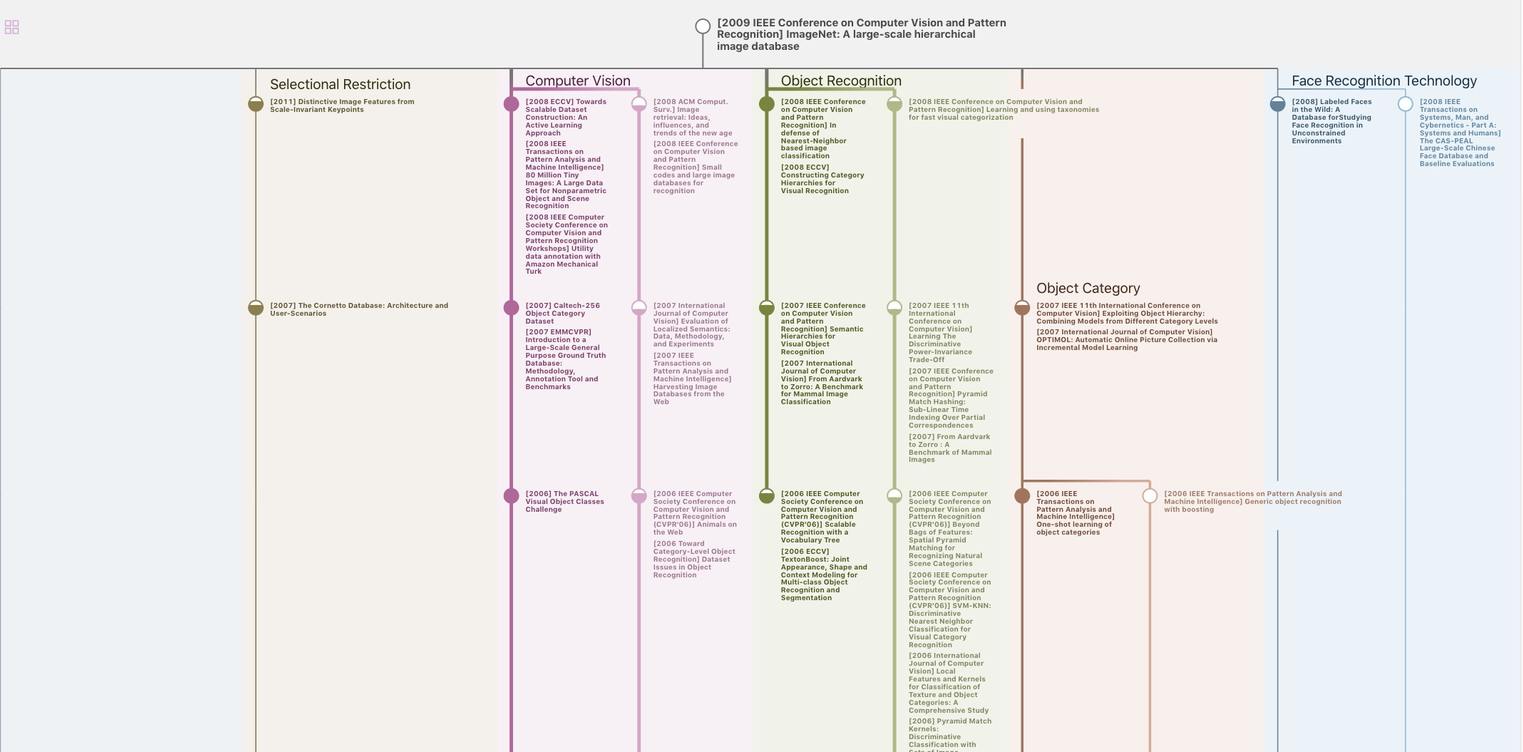
Generate MRT to find the research sequence of this paper
Chat Paper
Summary is being generated by the instructions you defined