TRCDNet: A Transformer Network for Video Cloud Detection.
IEEE Trans. Geosci. Remote. Sens.(2023)
摘要
In remote-sensing image (RSI) preprocessing steps, detecting and removing cloudy areas is a critical task. Recently, cloud detection methods based on deep neural networks achieve outstanding performance over traditional methods. Current approaches mostly focus on cloud detection on a single image captured by polar-orbiting satellites. However, there is another type of meteorological satellite-geostationary satellite, which can capture temporal consecutive frames of a particular location. Therefore, the cloud detection task targeting a geostationary satellite can be treated as a video cloud detection task. And in addition to extracting features on a single image, extracting and making full use of the relations between sequential frames is also important. To tackle this problem, we design a deep-learning video cloud detection model: transformer network for video cloud detection (TRCDNet). The proposed network is based on the encoder-decoder structure. In the encoder, the module ContextGhostLayer is proposed to encode more semantic information to tackle challenging problems like thin clouds in RSIs. Besides, we design a transformer-based video sequence transformer (VSTR) block. Based on the attention mechanism, VSTR can fully extract the across-frame relations. In the proposed decoder, the cloud masks are recovered gradually to the same scale as the input image. To evaluate the methods, we create a Video Cloud Detection dataset based on the captured videos from Fengyun 4 (FY-4) satellite: Fengyun4aCloud. Extensive experiments of current cloud detection methods, semantic segmentation methods, and video semantic segmentation (VSS) methods indicate that the designed TRCDNet achieves state-of-art performance in video cloud detection.
更多查看译文
关键词
trcdnet,transformer network,cloud,detection
AI 理解论文
溯源树
样例
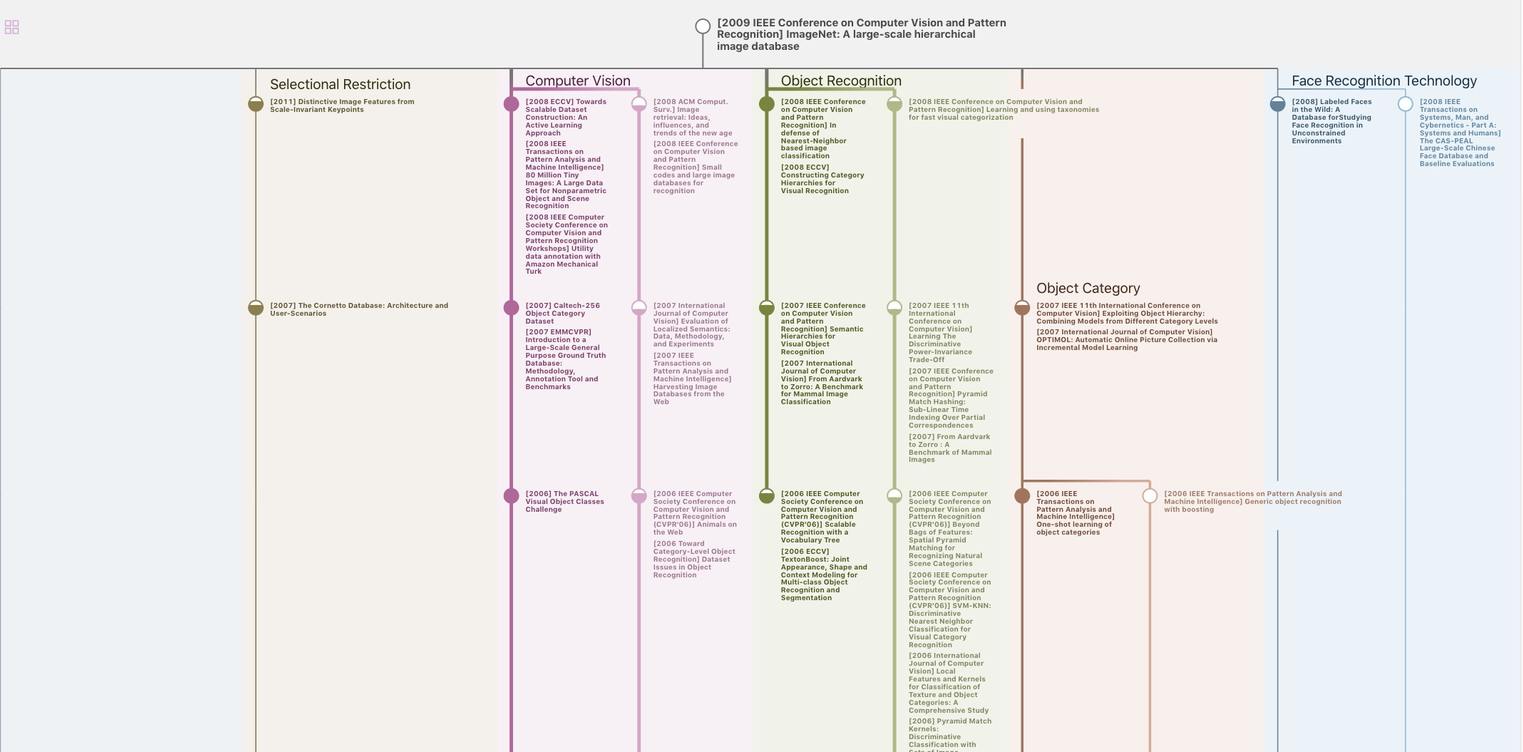
生成溯源树,研究论文发展脉络
Chat Paper
正在生成论文摘要