A Survey of the Impact of Self-Supervised Pretraining for Diagnostic Tasks with Radiological Images
CoRR(2023)
摘要
Self-supervised pretraining has been observed to be effective at improving feature representations for transfer learning, leveraging large amounts of unlabelled data. This review summarizes recent research into its usage in X-ray, computed tomography, magnetic resonance, and ultrasound imaging, concentrating on studies that compare self-supervised pretraining to fully supervised learning for diagnostic tasks such as classification and segmentation. The most pertinent finding is that self-supervised pretraining generally improves downstream task performance compared to full supervision, most prominently when unlabelled examples greatly outnumber labelled examples. Based on the aggregate evidence, recommendations are provided for practitioners considering using self-supervised learning. Motivated by limitations identified in current research, directions and practices for future study are suggested, such as integrating clinical knowledge with theoretically justified self-supervised learning methods, evaluating on public datasets, growing the modest body of evidence for ultrasound, and characterizing the impact of self-supervised pretraining on generalization.
更多查看译文
关键词
pretraining,diagnostic
AI 理解论文
溯源树
样例
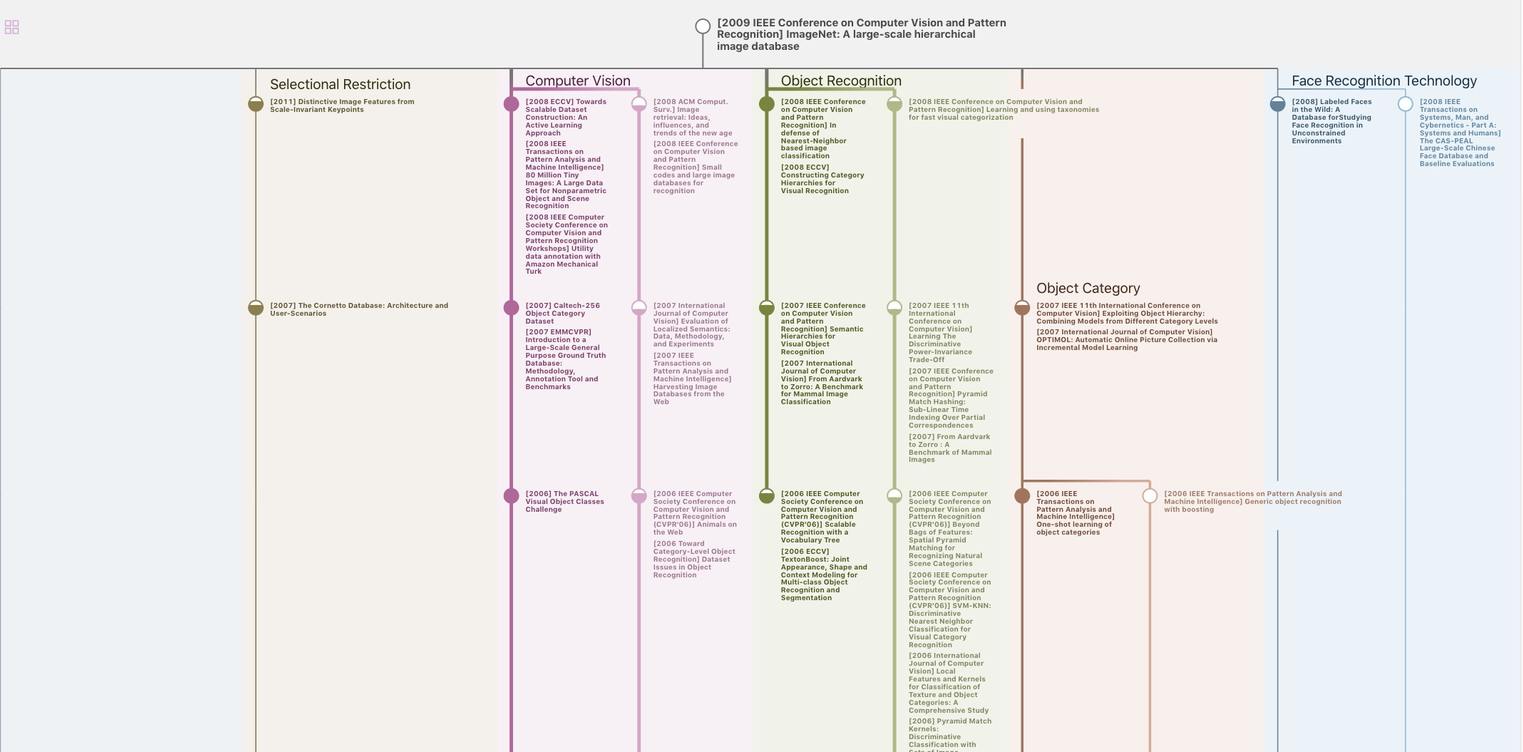
生成溯源树,研究论文发展脉络
Chat Paper
正在生成论文摘要