Deep Learning Enhanced Tandem Repeat Variation Identification via Multi-Modal Conversion of Nanopore Reads Alignment
bioRxiv (Cold Spring Harbor Laboratory)(2023)
摘要
Identification of tandem repeat (TR) variations plays a crucial role in advancing our understanding of genetic diseases, forensic analysis, evolutionary studies, and crop improvement, thereby contributing to various fields of research and practical applications. However, traditional TR identification methods are often limited to processing genomes obtained through sequence assembly and cannot directly start detection from sequencing reads. Furthermore, the inflexibility of detection mode and parameters hinders the accuracy and completeness of the identification, rendering the results unsatisfactory. These shortcomings result in existing TR variation identification methods being associated with high computational cost, limited detection sensitivity, precision and comprehensiveness. Here, we propose DeepTRs, a novel method for identifying TR variations, which enables direct TR variation identification from raw Nanopore sequencing reads and achieves high sensitivity, accuracy, and completeness results through the multi-modal conversion of Nanopore reads alignment and deep learning. Comprehensive evaluations demonstrate that DeepTRs outperform existing methods.
### Competing Interest Statement
The authors have declared no competing interest.
更多查看译文
关键词
deep learning,variation,multi-modal
AI 理解论文
溯源树
样例
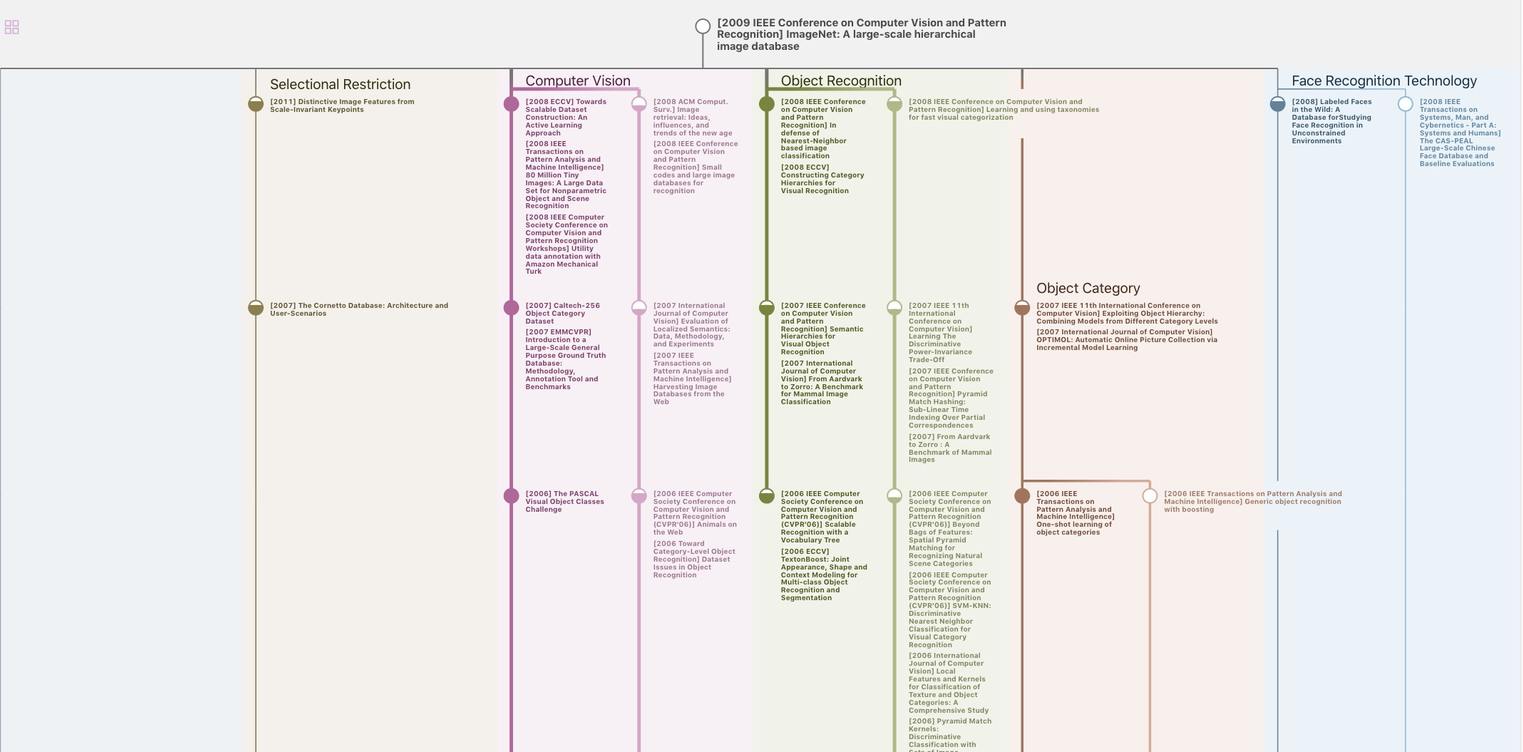
生成溯源树,研究论文发展脉络
Chat Paper
正在生成论文摘要