SHIFT3D: Synthesizing Hard Inputs for Tricking 3D Detectors.
Proceedings of the IEEE/CVF International Conference on Computer Vision (ICCV)(2023)
摘要
We present SHIFT3D, a differentiable pipeline for generating 3D shapes that are structurally plausible yet challenging to 3D object detectors. In safety-critical applications like autonomous driving, discovering such novel challenging objects can offer insight into unknown vulnerabilities of 3D detectors. By representing objects with a signed distanced function (SDF), we show that gradient error signals allow us to smoothly deform the shape or pose of a 3D object in order to confuse a downstream 3D detector. Importantly, the objects generated by SHIFT3D physically differ from the baseline object yet retain a semantically recognizable shape. Our approach provides interpretable failure modes for modern 3D object detectors, and can aid in preemptive discovery of potential safety risks within 3D perception systems before these risks become critical failures.
更多查看译文
关键词
Surface Defect Detection,Object Detection,Fabric Defect Detection,Virtual Metrology
AI 理解论文
溯源树
样例
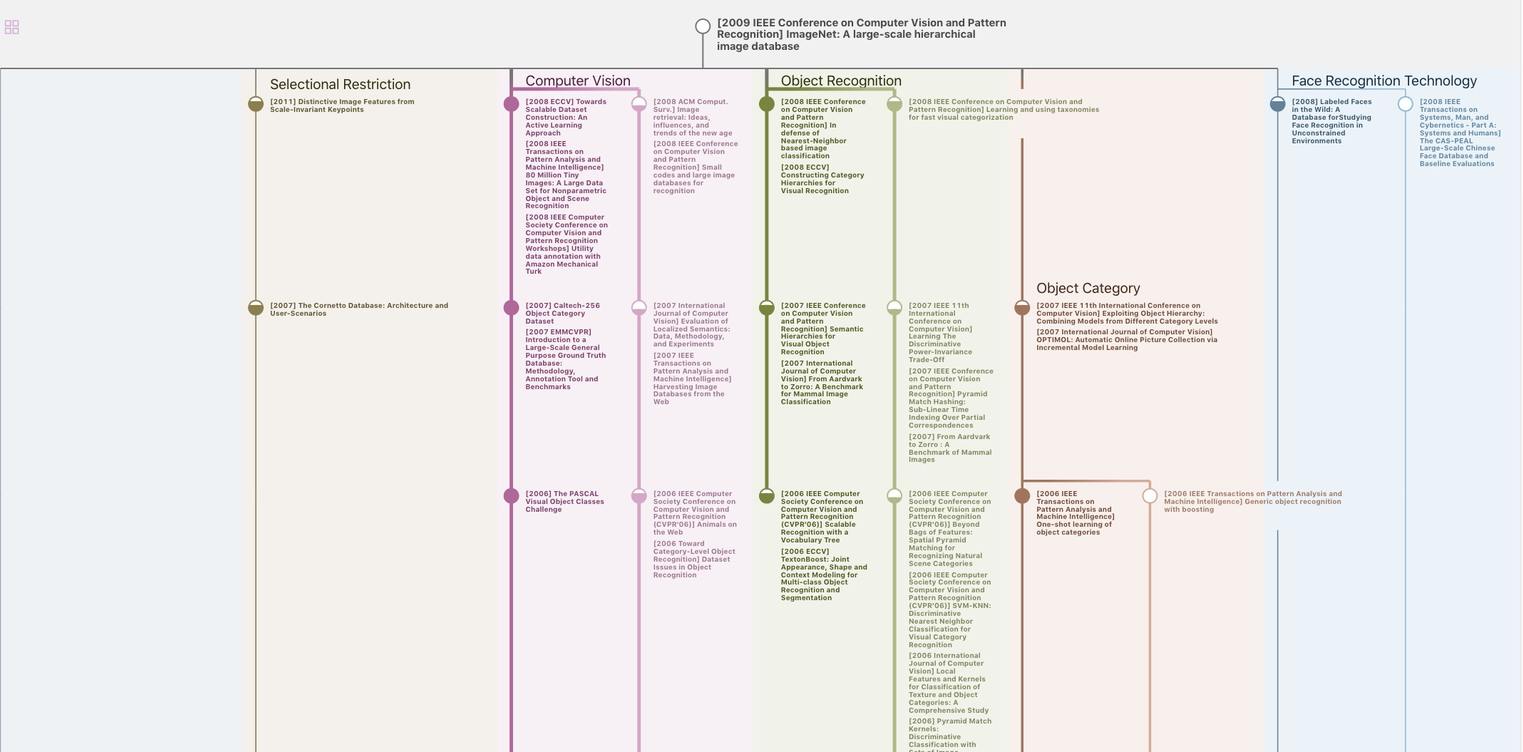
生成溯源树,研究论文发展脉络
Chat Paper
正在生成论文摘要