Ihas: Instance-wise Hierarchical Architecture Search for Deep Learning Recommendation Models
PROCEEDINGS OF THE 32ND ACM INTERNATIONAL CONFERENCE ON INFORMATION AND KNOWLEDGE MANAGEMENT, CIKM 2023(2023)
Abstract
Current recommender systems employ large-sized embedding tables with uniform dimensions for all features, leading to overfitting, high computational cost, and suboptimal generalizing performance. Many techniques aim to solve this issue by feature selection or embedding dimension search. However, these techniques typically select a fixed subset of features or embedding dimensions for all instances and feed all instances into one recommender model without considering heterogeneity between items or users. This paper proposes a novel instance-wise Hierarchical Architecture Search framework, iHAS, which automates neural architecture search at the instance level. Specifically, iHAS incorporates three stages: searching, clustering, and retraining. The searching stage identifies optimal instance-wise embedding dimensions across different field features via carefully designed Bernoulli gates with stochastic selection and regularizers. After obtaining these dimensions, the clustering stage divides samples into distinct groups via a deterministic selection approach of Bernoulli gates. The retraining stage then constructs different recommender models, each one designed with optimal dimensions for the corresponding group. We conduct extensive experiments to evaluate the proposed iHAS on two public benchmark datasets from a real-world recommender system. The experimental results demonstrate the effectiveness of iHAS and its outstanding transferability to widely-used deep recommendation models.
MoreTranslated text
Key words
recommender system,instance-wise,embedding dimension search
PDF
View via Publisher
AI Read Science
AI Summary
AI Summary is the key point extracted automatically understanding the full text of the paper, including the background, methods, results, conclusions, icons and other key content, so that you can get the outline of the paper at a glance.
Example
Background
Key content
Introduction
Methods
Results
Related work
Fund
Key content
- Pretraining has recently greatly promoted the development of natural language processing (NLP)
- We show that M6 outperforms the baselines in multimodal downstream tasks, and the large M6 with 10 parameters can reach a better performance
- We propose a method called M6 that is able to process information of multiple modalities and perform both single-modal and cross-modal understanding and generation
- The model is scaled to large model with 10 billion parameters with sophisticated deployment, and the 10 -parameter M6-large is the largest pretrained model in Chinese
- Experimental results show that our proposed M6 outperforms the baseline in a number of downstream tasks concerning both single modality and multiple modalities We will continue the pretraining of extremely large models by increasing data to explore the limit of its performance
Try using models to generate summary,it takes about 60s
Must-Reading Tree
Example
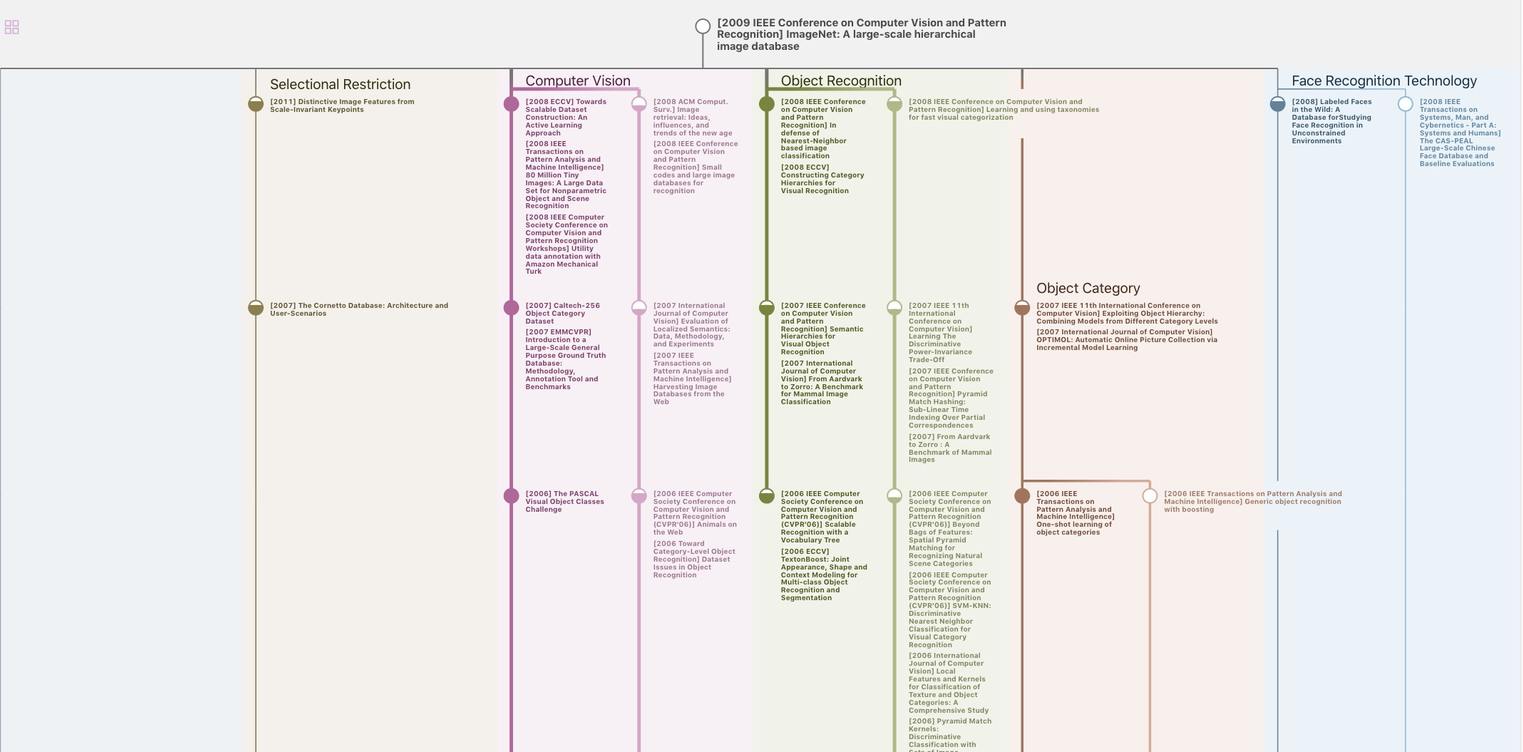
Generate MRT to find the research sequence of this paper
Related Papers
1954
被引用267 | 浏览
International World Wide Web Conference 2018
被引用899 | 浏览
2022
被引用27 | 浏览
Data Disclaimer
The page data are from open Internet sources, cooperative publishers and automatic analysis results through AI technology. We do not make any commitments and guarantees for the validity, accuracy, correctness, reliability, completeness and timeliness of the page data. If you have any questions, please contact us by email: report@aminer.cn
Chat Paper
去 AI 文献库 对话