Navigating the Design Space of Equivariant Diffusion-Based Generative Models for De Novo 3D Molecule Generation
ICLR 2024(2023)
摘要
Deep generative diffusion models are a promising avenue for de novo 3D molecular design in material science and drug discovery. However, their utility is still constrained by suboptimal performance with large molecular structures and limited training data. Addressing this gap, we explore the design space of E(3) equivariant diffusion models, focusing on previously blank spots. Our extensive comparative analysis evaluates the interplay between continuous and discrete state spaces. Out of this investigation, we introduce the EQGAT-diff model, which consistently surpasses the performance of established models on the QM9 and GEOM-Drugs datasets by a large margin. Distinctively, EQGAT-diff takes continuous atomic positions while chemical elements and bond types are categorical and employ a time-dependent loss weighting that significantly increases training convergence and the quality of generated samples. To further strengthen the applicability of diffusion models to limited training data, we examine the transferability of EQGAT-diff trained on the large PubChem3D dataset with implicit hydrogens to target distributions with explicit hydrogens. Fine-tuning EQGAT-diff for a couple of iterations further pushes state-of-the-art performance across datasets. We envision that our findings will find applications in structure-based drug design, where the accuracy of generative models for small datasets of complex molecules is critical.
更多查看译文
关键词
Generative Modelling,Molecule Design,Denoising Diffusion Probabilistic Models,Ablation Study,Equivariant Graph Neural Network,3D Molecule Generation,Diffusion Model
AI 理解论文
溯源树
样例
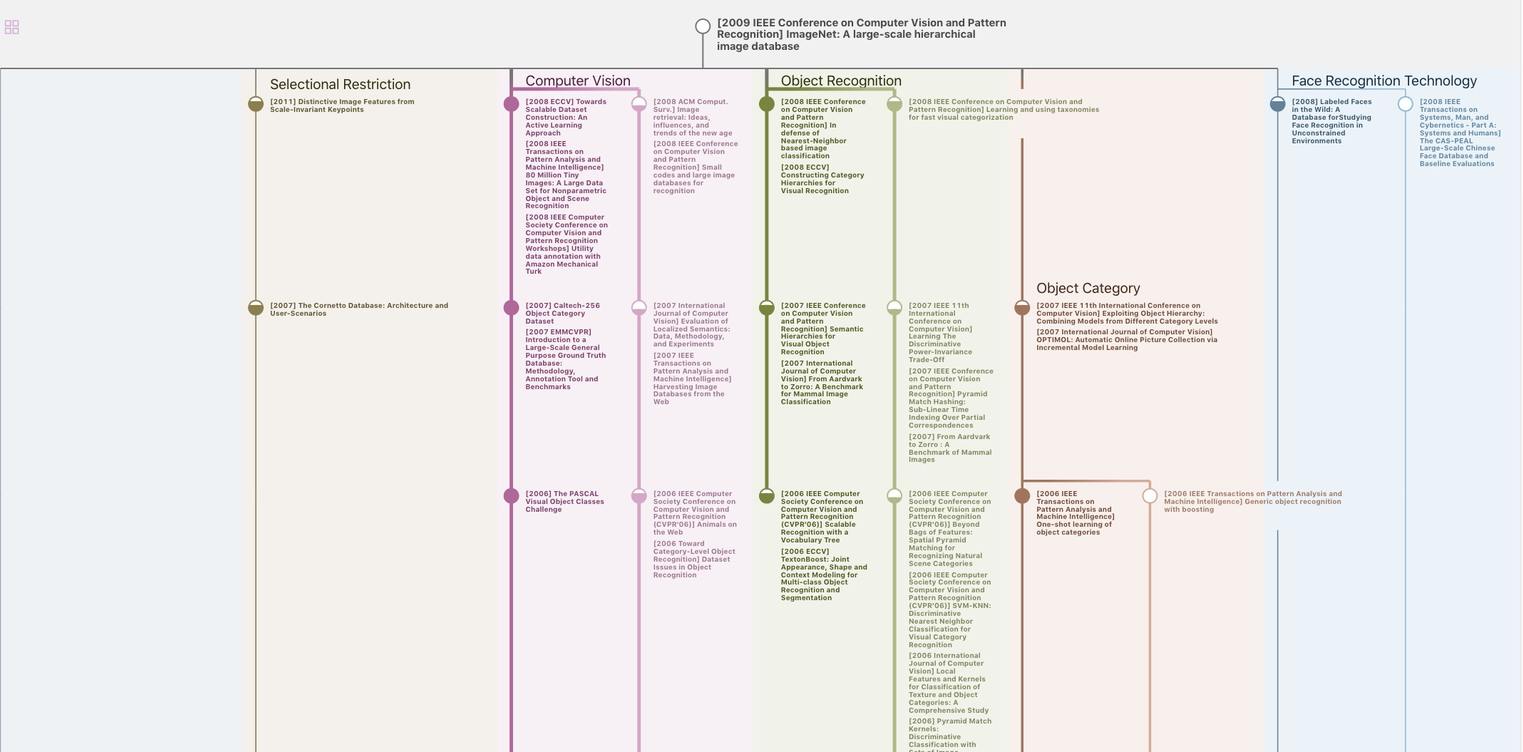
生成溯源树,研究论文发展脉络
Chat Paper
正在生成论文摘要