Visual Embedding Augmentation in Fourier Domain for Deep Metric Learning
IEEE Transactions on Circuits and Systems for Video Technology(2023)
摘要
Deep Metric Learning (DML) is very effective for many computer vision applications such as image retrieval or cross-modal matching. The common paradigm for DML is to seek metric spaces that can encode semantically similar objects close while locating the dissimilar ones far away from each other. To make features more discriminative, the mainstream methods usually design various specific loss functions to seek the help of hard negatives through complex hard mining strategies or hard synthesizing with additional networks. In spite of their fruitfulness, these approaches ignore the impact of low-level information in images on the performance, which may degrade the discerning ability of learned embedding. To alleviate these problems, we introduce a simple yet effective augmentation method to generate more hard negatives by swapping the low-frequency spectra of negative instances with anchors in the Fourier domain. Specifically, unlike previous methods, our proposed approach does not involve any complex design strategies but enriches hard negatives by manipulating the low-level variability of images only with simple Fourier transforms. In addition, our method is treated as a universal plug-in, which can be incorporated into different models for performance improvement. In the end, we conduct extensive experiments to evaluate our method on the widely-used datasets including CUB-200–2011, CARS-196, and Stanford Online Products. Our quantitative results demonstrate that the proposed plug-in outperforms previous approaches consistently and significantly across different datasets and evaluation metrics.
更多查看译文
关键词
augmentation,fourier domain,deep,learning
AI 理解论文
溯源树
样例
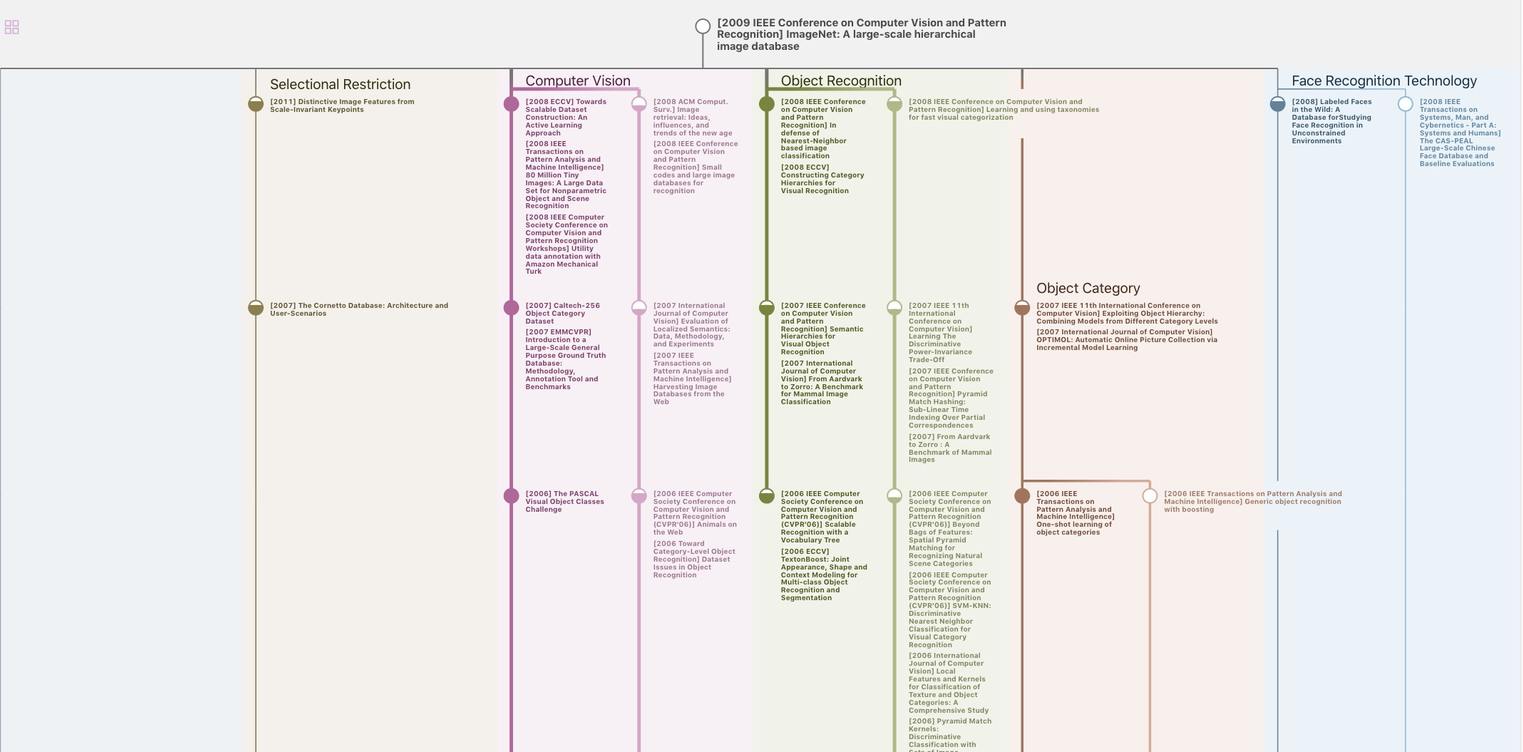
生成溯源树,研究论文发展脉络
Chat Paper
正在生成论文摘要