Resilient Legged Local Navigation: Learning to Traverse with Compromised Perception End-to-End
CoRR(2023)
摘要
Autonomous robots must navigate reliably in unknown environments even under compromised exteroceptive perception, or perception failures. Such failures often occur when harsh environments lead to degraded sensing, or when the perception algorithm misinterprets the scene due to limited generalization. In this paper, we model perception failures as invisible obstacles and pits, and train a reinforcement learning (RL) based local navigation policy to guide our legged robot. Unlike previous works relying on heuristics and anomaly detection to update navigational information, we train our navigation policy to reconstruct the environment information in the latent space from corrupted perception and react to perception failures end-to-end. To this end, we incorporate both proprioception and exteroception into our policy inputs, thereby enabling the policy to sense collisions on different body parts and pits, prompting corresponding reactions. We validate our approach in simulation and on the real quadruped robot ANYmal running in real-time (<10 ms CPU inference). In a quantitative comparison with existing heuristic-based locally reactive planners, our policy increases the success rate over 30% when facing perception failures. Project Page: https://bit.ly/45NBTuh.
更多查看译文
关键词
traverse,learning,perception,end-to-end
AI 理解论文
溯源树
样例
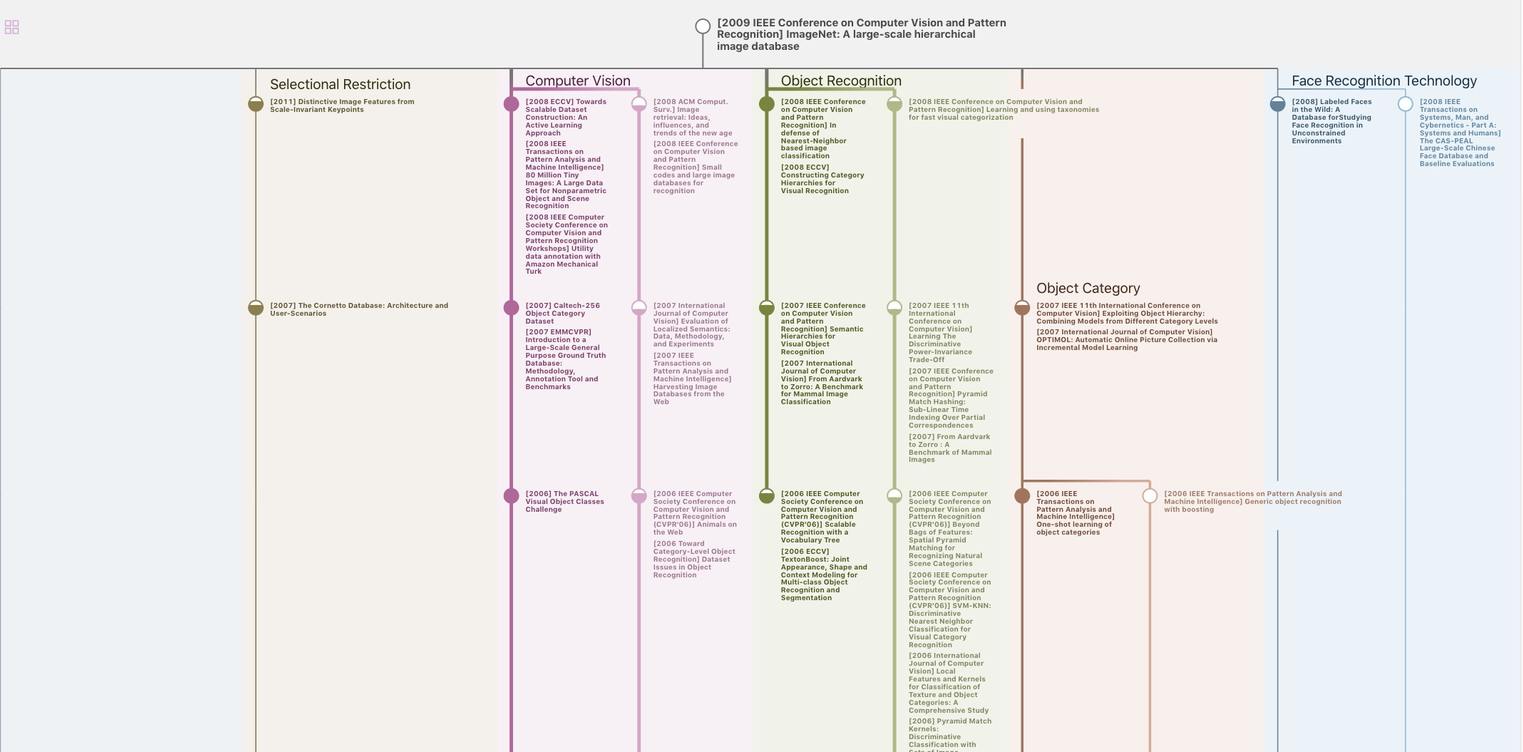
生成溯源树,研究论文发展脉络
Chat Paper
正在生成论文摘要