A heterogeneous graph neural network model for list recommendation.
Knowl. Based Syst.(2023)
摘要
List recommendation, an approach for recommending a set of items closely related to user preferences, plays a significant role in improving the user experience and has received growing attention in recent years. However, most existing models ignore list-level attributes and the heterogeneity of the interactions between the users and lists when predicting user preferences. In this paper, we propose a heterogeneous graph neural network list recommendation (HGLR) model to bridge these gaps. First, a representation learning module based on pre-training techniques is developed to learn the embeddings of the lists, by encoding textual information and aggregating the features of the constituent items. We then transform the user-list interaction into a bipartite graph and incorporate the list attributes into a heterogeneous information network (HIN). Propagation-based embedding learning is subsequently performed to generate user and list representation based on the message-passing mechanism of the graph neural network. Finally, an attentive fusion layer is designed to obtain the global representations for the recommendation. We also develop a new large-scale dataset containing multiple types of side information to evaluate the list recommendation model. Extensive experiments demonstrate that our model significantly outperforms state-of-the-art list recommendation methods and achieves performance gains over 8.96% and 14.59% in Recall and NDCG, respectively, against the strongest baselines.
更多查看译文
关键词
List recommendation,Bundle recommendation,Graph neural network,Heterogeneous information network
AI 理解论文
溯源树
样例
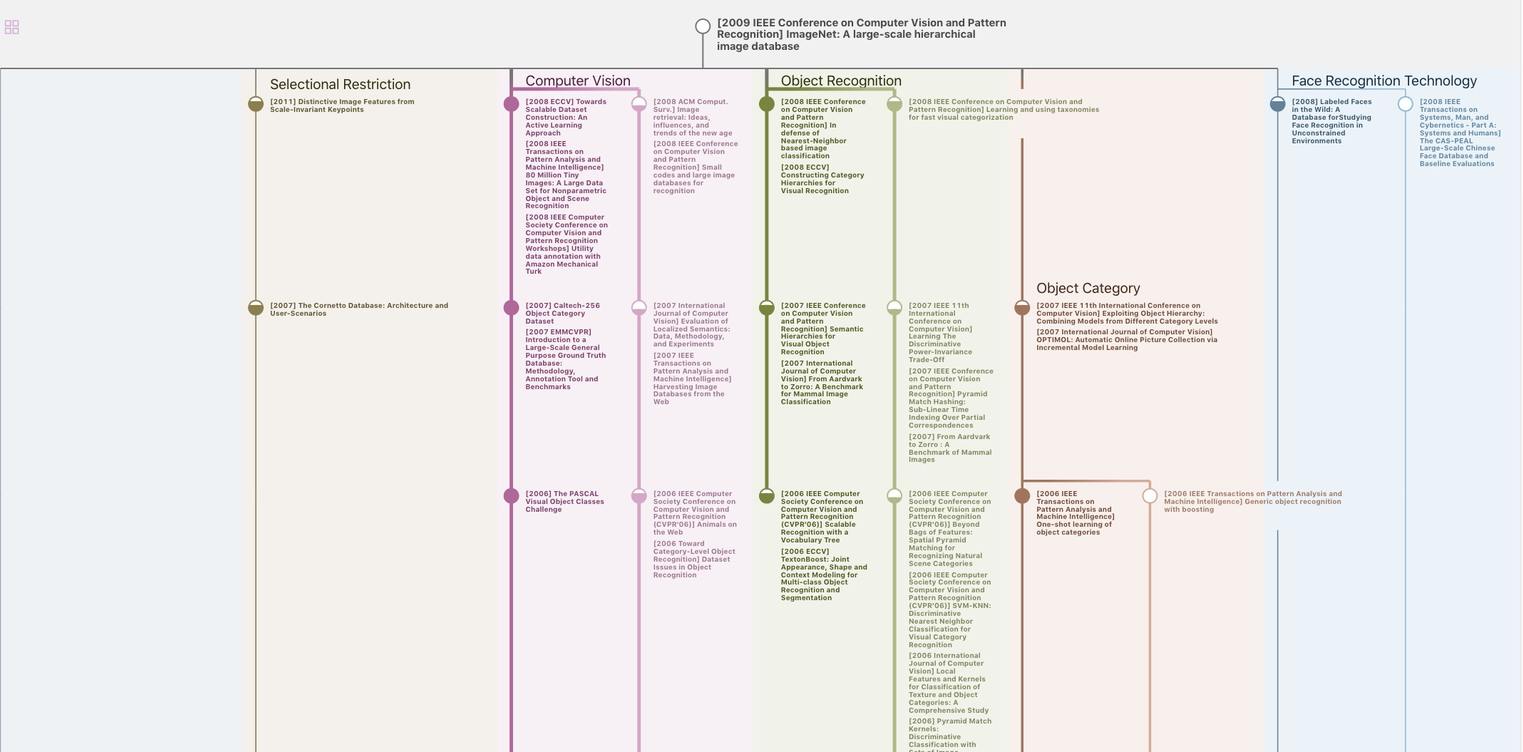
生成溯源树,研究论文发展脉络
Chat Paper
正在生成论文摘要