Extreme value modeling with errors-in-variables in detection and attribution of changes in climate extremes
Stat. Comput.(2023)
摘要
The generalized extreme value (GEV) regression provides a framework for modeling extreme events across various fields by incorporating covariates into the location parameter of GEV distributions. When the covariates are subject to errors-in-variables (EIV) or measurement error, ignoring the EIVs leads to biased estimation and degraded inferences. This problem arises in detection and attribution analyses of changes in climate extremes because the covariates are estimated with uncertainty. It has not been studied even for the case of independent EIVs, let alone the case of dependent EIVs, due to the complex structure of GEV. Here we propose a general Monte Carlo corrected score method and extend it to address temporally correlated EIVs in GEV modeling with application to the detection and attribution analyses for climate extremes. Through extensive simulation studies, the proposed method provides an unbiased estimator and valid inference. In the application to the detection and attribution analyses of temperature extremes in central regions of China, with the proposed method, the combined anthropogenic and natural signal is detected in the change in the annual minimum of daily maximum and the annual minimum of daily minimum.
更多查看译文
关键词
Corrected score,Estimating equation,Measurement error,Monte Carlo
AI 理解论文
溯源树
样例
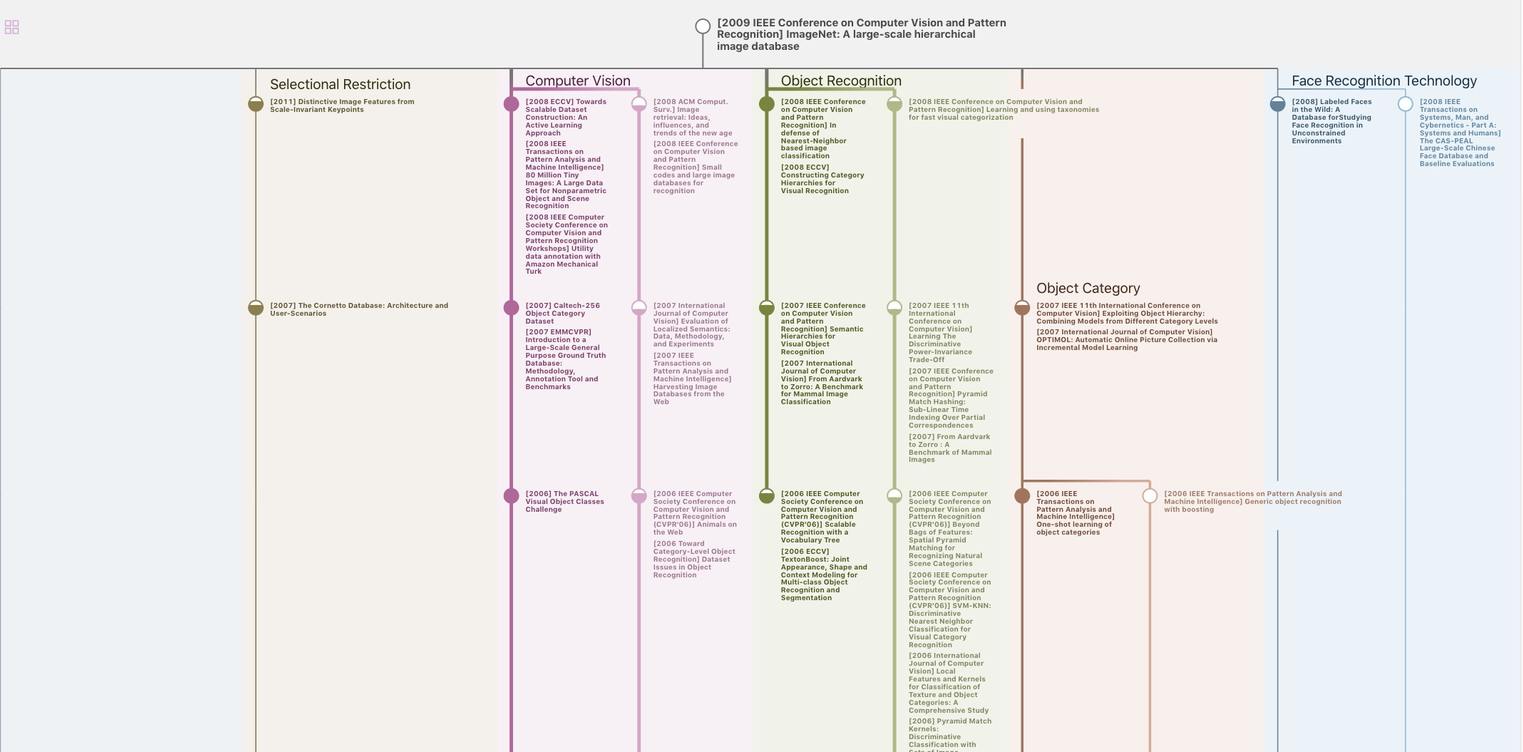
生成溯源树,研究论文发展脉络
Chat Paper
正在生成论文摘要