RuleDRL: Reliability-Aware SFC Provisioning With Bounded Approximations in Dynamic Environments
IEEE Transactions on Services Computing(2023)
摘要
As a key enabling technology for 5G, network function virtualization abstracts services into software-based service function chains (SFCs), facilitating mission-critical services with high-reliability requirements. However, it is challenging to cost-effectively provide reliable SFCs in dynamic environments due to delayed rewards caused by future SFC requests, limited infrastructure resources, and heterogeneity in hardware and software reliability. Although deep reinforcement learning (DRL) can effectively capture delayed rewards in dynamic environments, its trial-and-error exploration in a vast solution space with massive infeasible solutions may lead to frequent constraint violations and traps in poor local optima. To address these challenges, we propose a RuleDRL algorithm that combines the capability of DRL to capture delayed rewards and the strength of rule-based schemes to explore high-quality solutions without violating constraints. Specifically, we first formulate the reliable SFC provision problem as an integer nonlinear programming problem, which is proven to be NP-hard. Then, we jointly design DRL and rule-based schemes that are coupled to make the final decision and establish a bounded approximation ratio in general cases. Extensive trace-driven simulations show that RuleDRL can save the total cost by up to 65.67% and improve the SFC acceptance ratio by up to 82%, compared to the state-of-the-art solution.
更多查看译文
关键词
Network function virtualization, edge computing, 5G, deep reinforcement learning
AI 理解论文
溯源树
样例
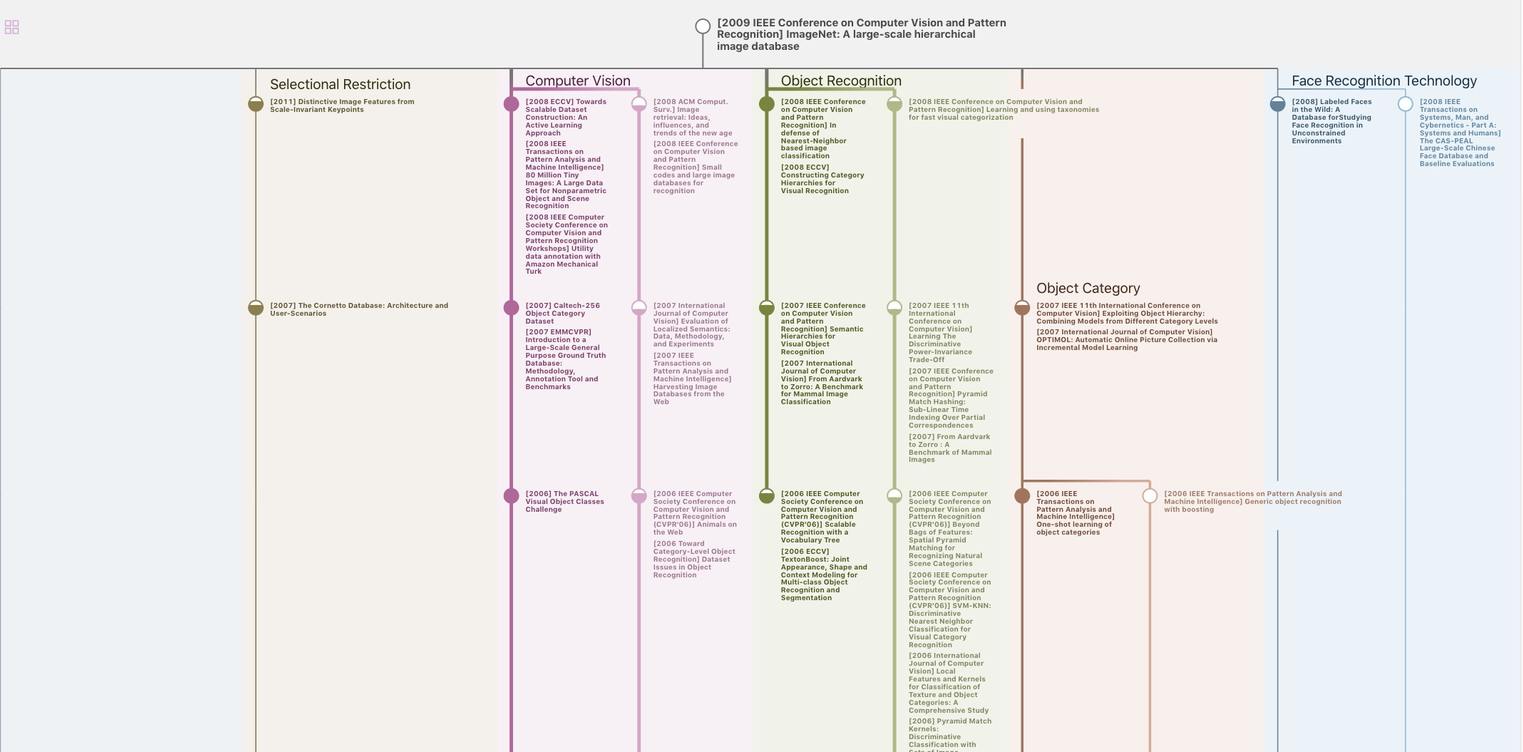
生成溯源树,研究论文发展脉络
Chat Paper
正在生成论文摘要