Deep Learning-based Sequence Labeling Tools for Nepali
ACM Transactions on Asian and Low-Resource Language Information Processing(2023)
摘要
A Part-of-Speech (POS) tagger and Chunker (or shallow parser) are sequence labeling tools, crucial for improving the accuracy of Natural Language Processing (NLP) tasks like parsing, named entity recognition, sentiment analysis, information extraction, and so on. Developing such tools for a low-resource language is an arduous task. Nepali is a relatively resource-poor Indian language and has not been able to evolve from a computational perspective. Therefore, we present effective part-of-speech tagging and chunking tools for the Nepali text using sequential deep learning models-Bidirectional Long Short-Term Memory Network with a Conditional Random Field Layer (BI-LSTM-CRF) and other LSTM-based models exploring both character and word embeddings of the Nepali texts. Word Embedding has been used to capture syntactic as well as semantic information whereas character embedding has been applied to capture the morphological as well as shape information of words and also to handle the out-of-vocabulary problem. The developed chunker is the first statistical chunker for the Nepali language. A baseline model with a Conditional Random Field has also been developed to identify the optimum feature set for the aforementioned tasks. The BI-LSTM-CRF model produced an accuracy of 99.20% and 98.40%, for Nepali POS tagging and chunking, respectively. This is the highest-ever accuracy for Nepali. Thorough error analysis and observations have also been reported with examples. The developed tools can help advance research in Nepali language processing, improve the accuracy of language technology applications, and contribute to the preservation and promotion of the Nepali language.
更多查看译文
关键词
Deep learning-based Nepali tools,Nepali sequence labeling tools,Nepali chunker,BI-LSTM-CRF neural network,Nepali text feature selection,Nepali optimum feature set
AI 理解论文
溯源树
样例
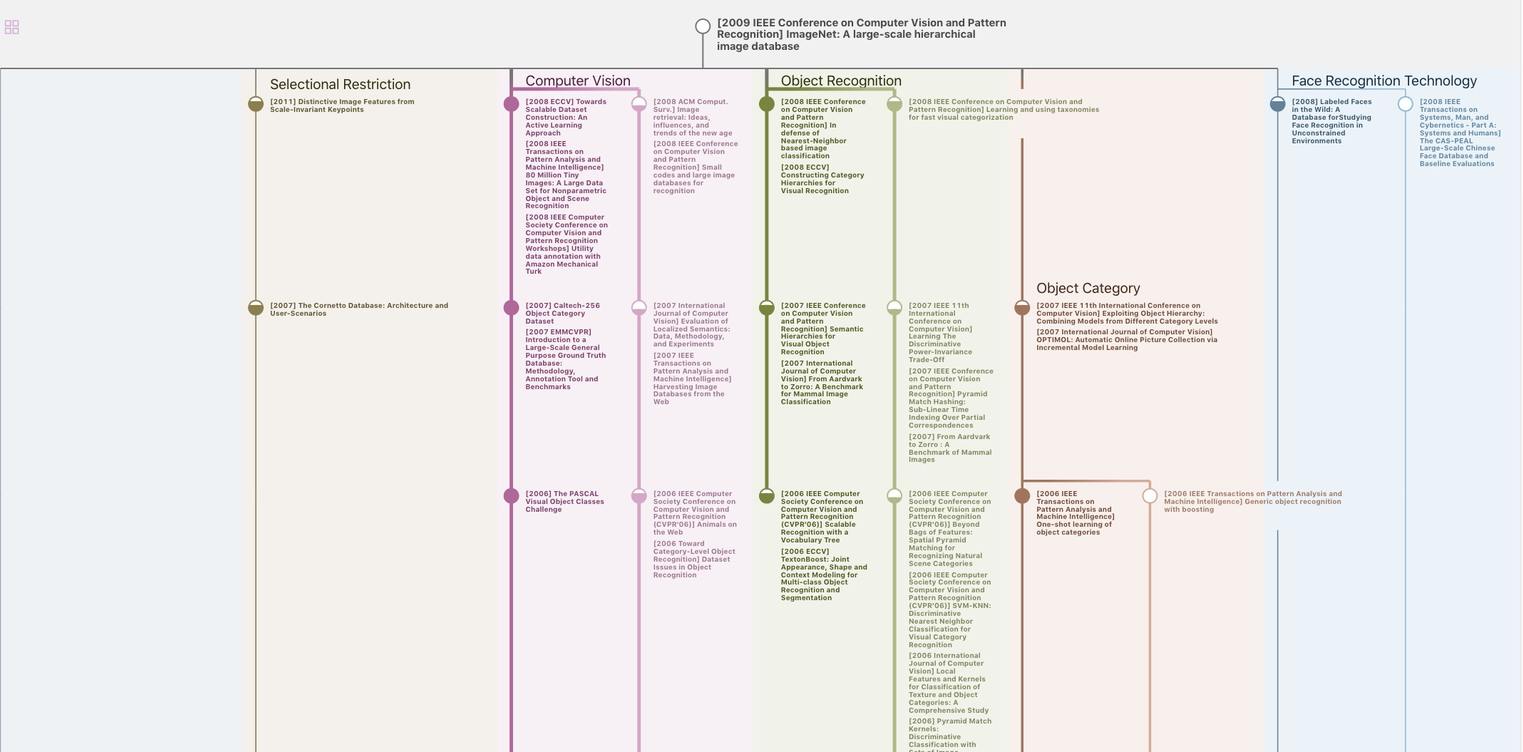
生成溯源树,研究论文发展脉络
Chat Paper
正在生成论文摘要