Quantifying the Effectiveness of Advertising: A Bootstrap Proportion Test for Brand Lift Testing
PROCEEDINGS OF THE 32ND ACM INTERNATIONAL CONFERENCE ON INFORMATION AND KNOWLEDGE MANAGEMENT, CIKM 2023(2023)
LinkedIn Corp | Univ Notre Dame
Abstract
Brand Lift test is a widely deployed statistical tool for measuring the effectiveness of online advertisements on brand perception such as ad recall, brand familiarity and favorability. By formulating the problem of interest into a two-sample test on the binomial proportions from the control group (p_0) and the treatment group (p_1), Brand Lift test evaluates ads impact based on the statistical significance of test results. Traditional approaches construct the test statistics based on the absolute difference between the two observed proportions, a.k.a, absolute lift. In this work, we propose a new bootstrap test based on the percentage difference between the two observed proportions, i.e., relative lift. We provide rigorous theoretical guarantees on the asymptotic validity of the proposed relative-lift-based test. Our numerical studies suggest that the relative-lift-based test requires less stringent conditions than the absolute-lift-based test for controlling the type-I error rate. Interestingly, we also prove that the relative-lift-based test is more powerful than the absolute-lift-based test when the alternative is positive (i.e., p1 - p0 > 0), but less powerful when the alternative is negative (i.e., p1 - p0 < 0). The empirical performance of the proposed test is demonstrated by extensive simulation studies, an application to a publicly available A/B testing dataset from advertising, and real datasets collected from the Brand Lift Testing platform at LinkedIn.
MoreTranslated text
Key words
biased test,binomial distribution,brand advertising,power enhancement,relative lift
求助PDF
上传PDF
View via Publisher
AI Read Science
AI Summary
AI Summary is the key point extracted automatically understanding the full text of the paper, including the background, methods, results, conclusions, icons and other key content, so that you can get the outline of the paper at a glance.
Example
Background
Key content
Introduction
Methods
Results
Related work
Fund
Key content
- Pretraining has recently greatly promoted the development of natural language processing (NLP)
- We show that M6 outperforms the baselines in multimodal downstream tasks, and the large M6 with 10 parameters can reach a better performance
- We propose a method called M6 that is able to process information of multiple modalities and perform both single-modal and cross-modal understanding and generation
- The model is scaled to large model with 10 billion parameters with sophisticated deployment, and the 10 -parameter M6-large is the largest pretrained model in Chinese
- Experimental results show that our proposed M6 outperforms the baseline in a number of downstream tasks concerning both single modality and multiple modalities We will continue the pretraining of extremely large models by increasing data to explore the limit of its performance
Upload PDF to Generate Summary
Must-Reading Tree
Example
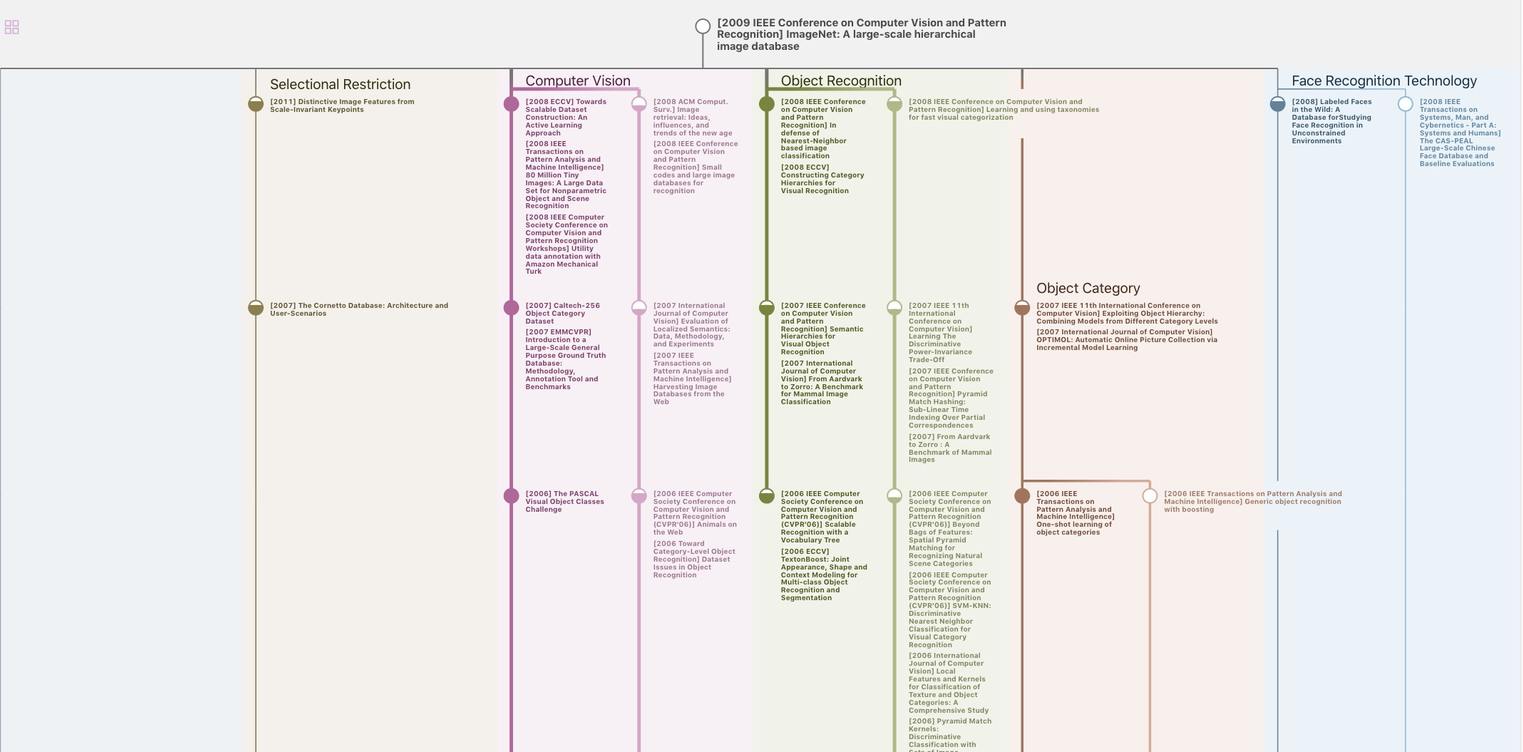
Generate MRT to find the research sequence of this paper
Related Papers
2013
被引用125 | 浏览
2000
被引用475 | 浏览
2015
被引用294 | 浏览
2013
被引用7 | 浏览
2023
被引用15 | 浏览
2022
被引用8 | 浏览
Data Disclaimer
The page data are from open Internet sources, cooperative publishers and automatic analysis results through AI technology. We do not make any commitments and guarantees for the validity, accuracy, correctness, reliability, completeness and timeliness of the page data. If you have any questions, please contact us by email: report@aminer.cn
Chat Paper
GPU is busy, summary generation fails
Rerequest