On Learning Gaussian Multi-index Models with Gradient Flow
CoRR(2023)
摘要
We study gradient flow on the multi-index regression problem for
high-dimensional Gaussian data. Multi-index functions consist of a composition
of an unknown low-rank linear projection and an arbitrary unknown,
low-dimensional link function. As such, they constitute a natural template for
feature learning in neural networks.
We consider a two-timescale algorithm, whereby the low-dimensional link
function is learnt with a non-parametric model infinitely faster than the
subspace parametrizing the low-rank projection. By appropriately exploiting the
matrix semigroup structure arising over the subspace correlation matrices, we
establish global convergence of the resulting Grassmannian population gradient
flow dynamics, and provide a quantitative description of its associated
`saddle-to-saddle' dynamics. Notably, the timescales associated with each
saddle can be explicitly characterized in terms of an appropriate Hermite
decomposition of the target link function. In contrast with these positive
results, we also show that the related \emph{planted} problem, where the link
function is known and fixed, in fact has a rough optimization landscape, in
which gradient flow dynamics might get trapped with high probability.
更多查看译文
关键词
gradient flow,models,multi-index
AI 理解论文
溯源树
样例
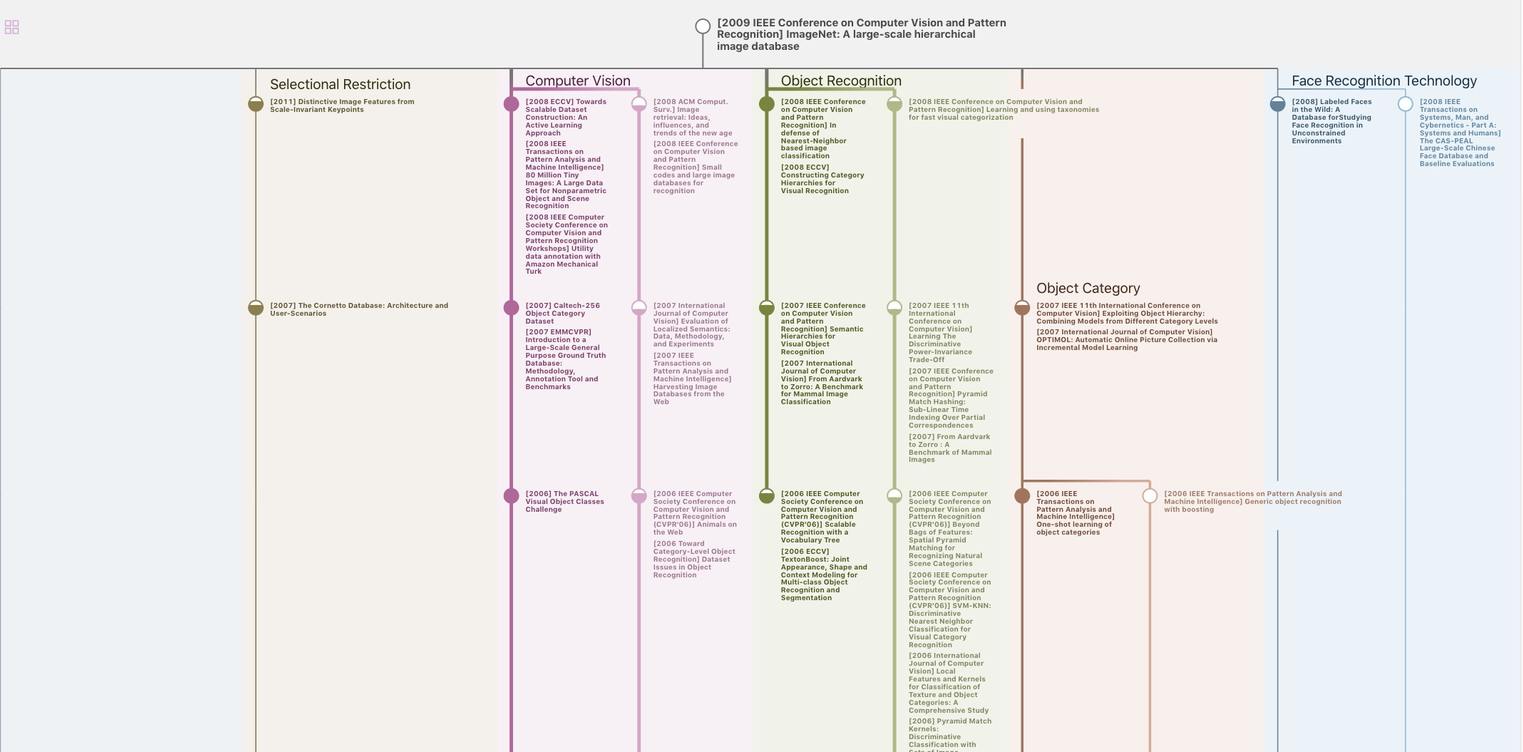
生成溯源树,研究论文发展脉络
Chat Paper
正在生成论文摘要