Exploring the Knowledge Transferred by Response-Based Teacher-Student Distillation
MM '23: Proceedings of the 31st ACM International Conference on Multimedia(2023)
摘要
Response-based Knowledge Distillation refers to the technique of supervising the student network with the teacher networks' predictions. The method is motivated by observing that the predicted probabilities reflect the relation among labels, which is the knowledge to be transferred. This paper explores the transferred knowledge from a novel perspective: comparing the knowledge transferred through different teachers. Two intriguing properties are observed. First, higher confidence scores of teachers' predictions lead to better distillation results, and second, teachers' incorrectly predicted training samples should be kept for distillation. We then analyze the phenomenon by studying teachers' decision boundaries, of which some can help the student generalize while some may not. Based on the observations, we further propose an embarrassingly simple distillation framework named Efficient Distillation, which is effective on ImageNet with different teacher-student pairs: When using ResNet34 as the teacher, the student ResNet18 trained from scratch reaches 74.07% Top-1 accuracy within 98 GPU hours (RTX 3090), outperforming current state-of-the-art result (73.19%) by a large margin. Our code is available at https://github.com/lsongx/EffDstl.
更多查看译文
AI 理解论文
溯源树
样例
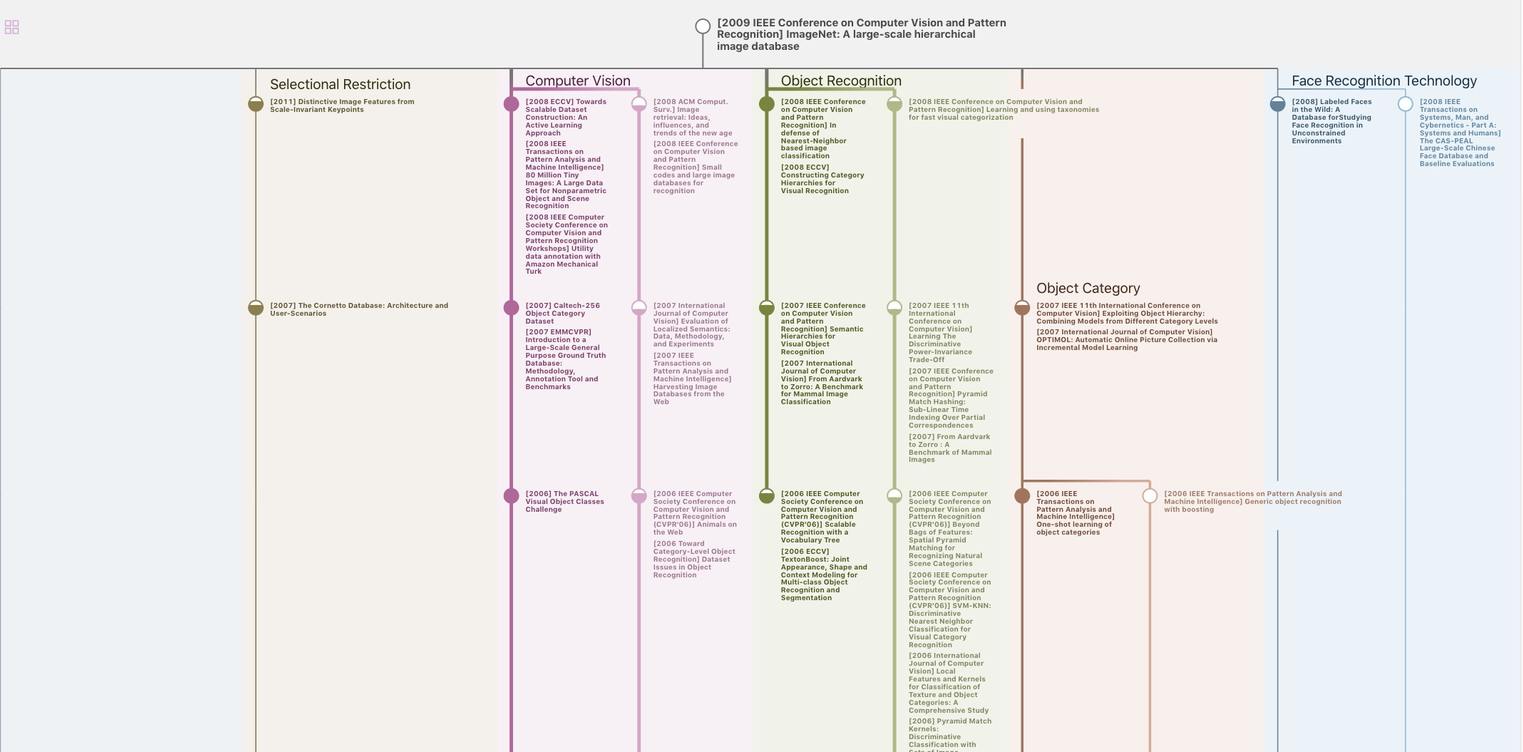
生成溯源树,研究论文发展脉络
Chat Paper
正在生成论文摘要