Learning Generalized Representations for Open-Set Temporal Action Localization
MM '23: Proceedings of the 31st ACM International Conference on Multimedia(2023)
摘要
Open-set Temporal Action Localization (OSTAL) is a critical and challenging task that aims to recognize and temporally localize human actions in untrimmed videos in open word scenarios. The main challenge in this task is the knowledge transfer from known actions to unknown actions. However, existing methods utilize limited training data and overparameterized deep neural network, which have poor generalization. This paper proposes a novel Generalized OSTAL model (namely GOTAL) to learn generalized representations of actions. GOTAL utilizes a Transformer network to model actions and a open-set detection head to perform action localization and recognition. Benefitting from Transformer's temporal modeling capabilities, GOTAL facilitates the extraction of human motion information from videos to mitigate the effects of irrelevant background data. Furthermore, a sharpness minimization algorithm is used to learn the network parameters of GOTAL, which facilitates the convergence of network parameters towards flatter minima by simultaneously minimizing the training loss value and sharpness of the loss plane. The collaboration of the above components significantly enhances the generalization of the representation. Experimental results demonstrate that GOTAL achieves the state-of-the-art performance on THUMOS14 and ActivityNet1.3 benchmarks, confirming the effectiveness of our proposed method.
更多查看译文
AI 理解论文
溯源树
样例
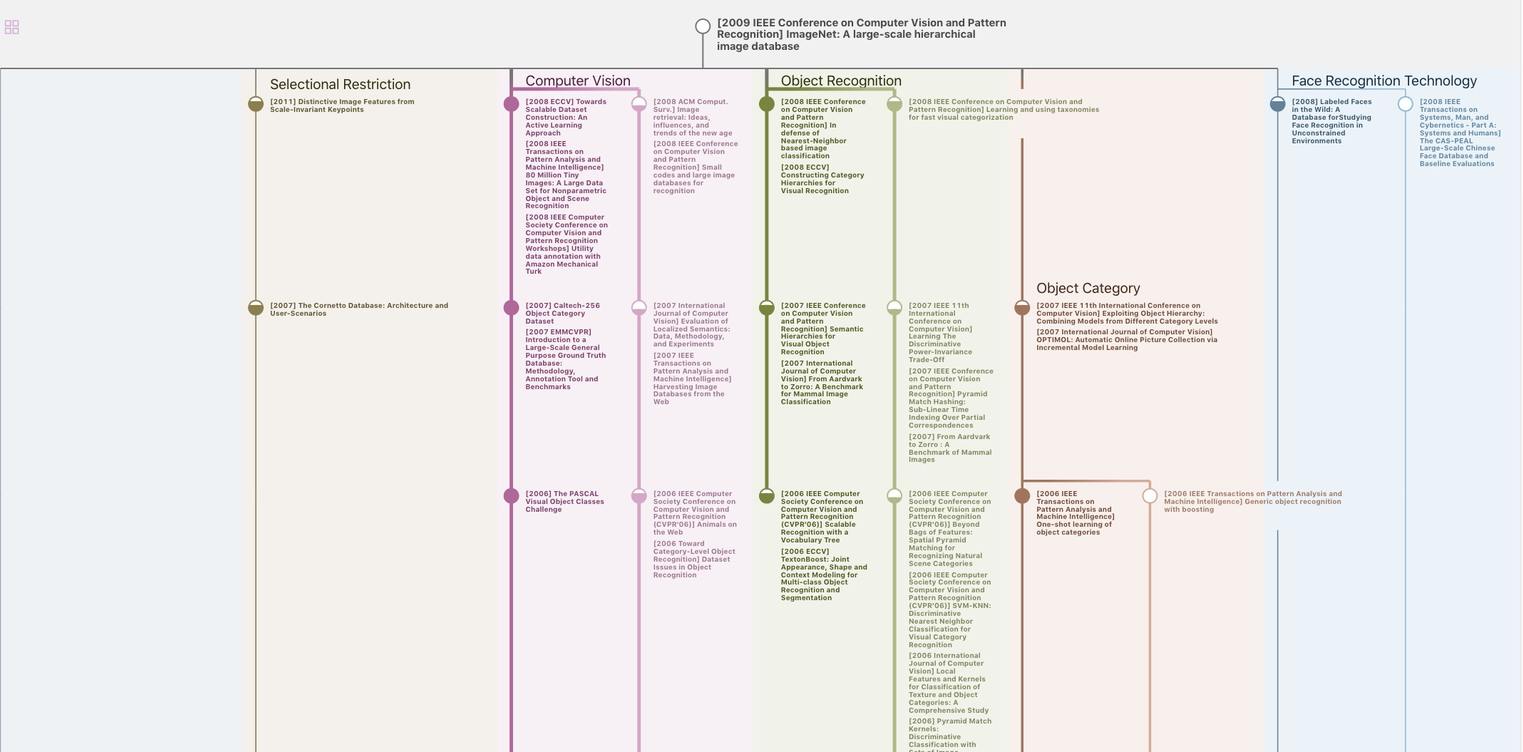
生成溯源树,研究论文发展脉络
Chat Paper
正在生成论文摘要