Adaptive Gradient Methods For Over-the-Air Federated Learning
2023 IEEE 24th International Workshop on Signal Processing Advances in Wireless Communications (SPAWC)(2023)
摘要
Federated learning (FL) provides a privacy-preserving approach to realizing networked intelligence. However, the performance of FL is often constrained by the limited communication resources, especially in the context of a wireless system. To tackle this communication bottleneck, recent studies propose an analog over-the-air (A-OTA) FL paradigm which employs A-OTA computations in the model aggregation step that significantly enhances scalability. The existing architectures mainly conduct model training via (stochastic) gradient descent, while adaptive optimization methods, which have achieved notable success in deep learning, remain unexplored. In this paper, we establish a distributed training paradigm that incorporates adaptive gradient methods into the A-OTA FL framework, aiming to enhance the system’s convergence performance. We derive an analytical expression for the convergence rate, capturing the effects of various system parameters on the convergence performance of the proposed method. We also perform several experiments to validate the efficacy of the proposed method.
更多查看译文
AI 理解论文
溯源树
样例
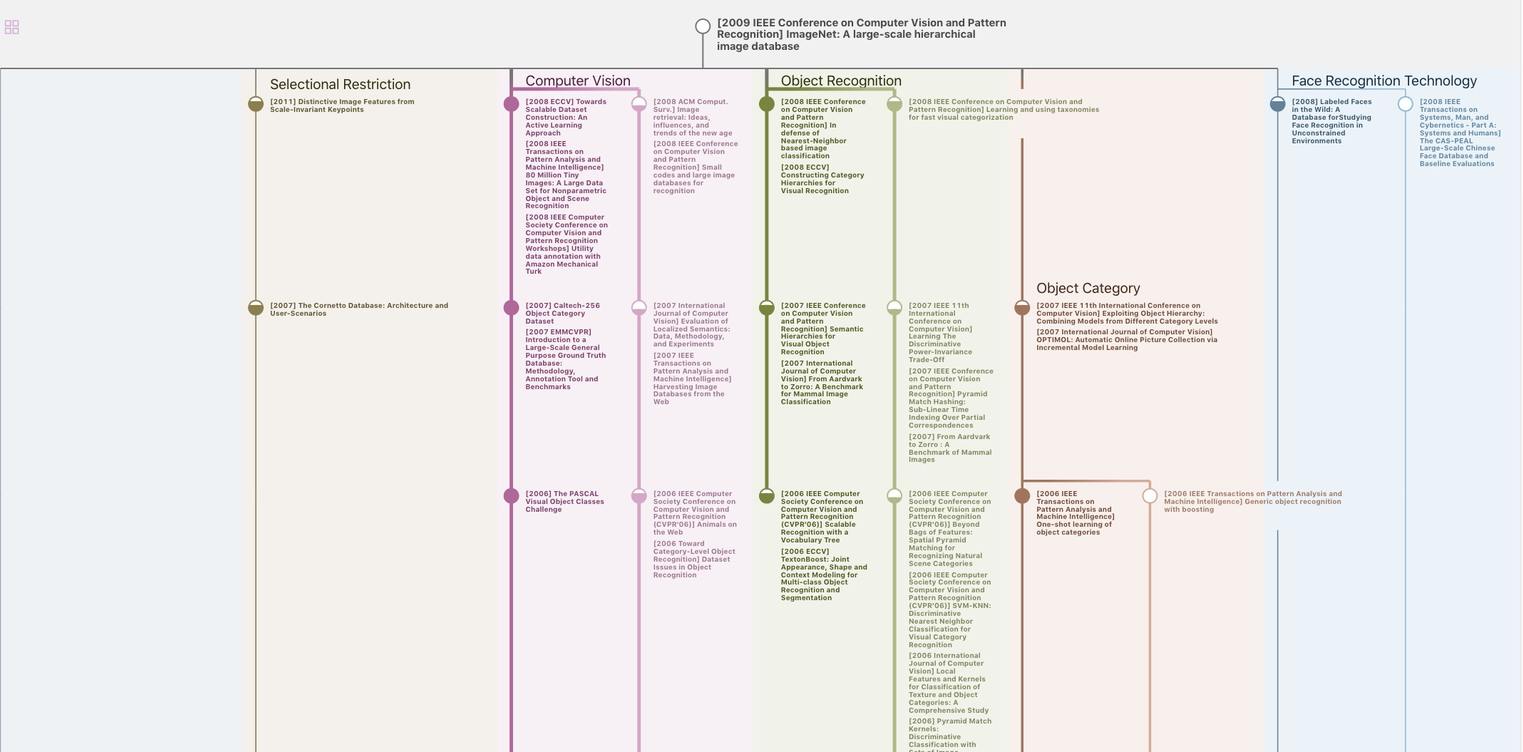
生成溯源树,研究论文发展脉络
Chat Paper
正在生成论文摘要