DeepComboSAD: Spectro-Temporal Correlation Based Speech Activity Detection for Naturalistic Audio Streams.
IEEE Signal Process. Lett.(2023)
摘要
Speech activity detection (SAD) serves as a crucial front-end system to several downstream Speech and Language Technology (SLT) tasks such as speaker diarization, speaker identification, and speech recognition. Recent years have seen deep learning (DL)-based SAD systems designed to improve robustness against static background noise and interfering speakers. However, SAD performance can be severely limited for conversations recorded in naturalistic environments due to dynamic acoustic scenarios and previously unseen non-speech artifacts. In this letter, we propose an end-to-end deep learning framework designed to be robust to time-varying noise profiles observed in naturalistic audio. We develop a novel SAD solution for the UTDallas Fearless Steps Apollo corpus based on NASA's Apollo missions. The proposed system leverages spectro-temporal correlations with a threshold optimization mechanism to adjust to acoustic variabilities across multiple channels and missions. This system is trained and evaluated on the Fearless Steps Challenge (FSC) corpus (a subset of the Apollo corpus). Experimental results indicate a high degree of adaptability to out-of-domain data, achieving a relative Detection Cost Function (DCF) performance improvement of over 50% compared to the previous FSC baselines and state-of-the-art (SOTA) SAD systems. The proposed model also outperforms the most recent DL-based SOTA systems from FSC Phase-4. Ablation analysis is conducted to confirm the efficacy of the proposed spectro-temporal features.
更多查看译文
关键词
speech activity detection,naturalistic audio streams,spectro-temporal
AI 理解论文
溯源树
样例
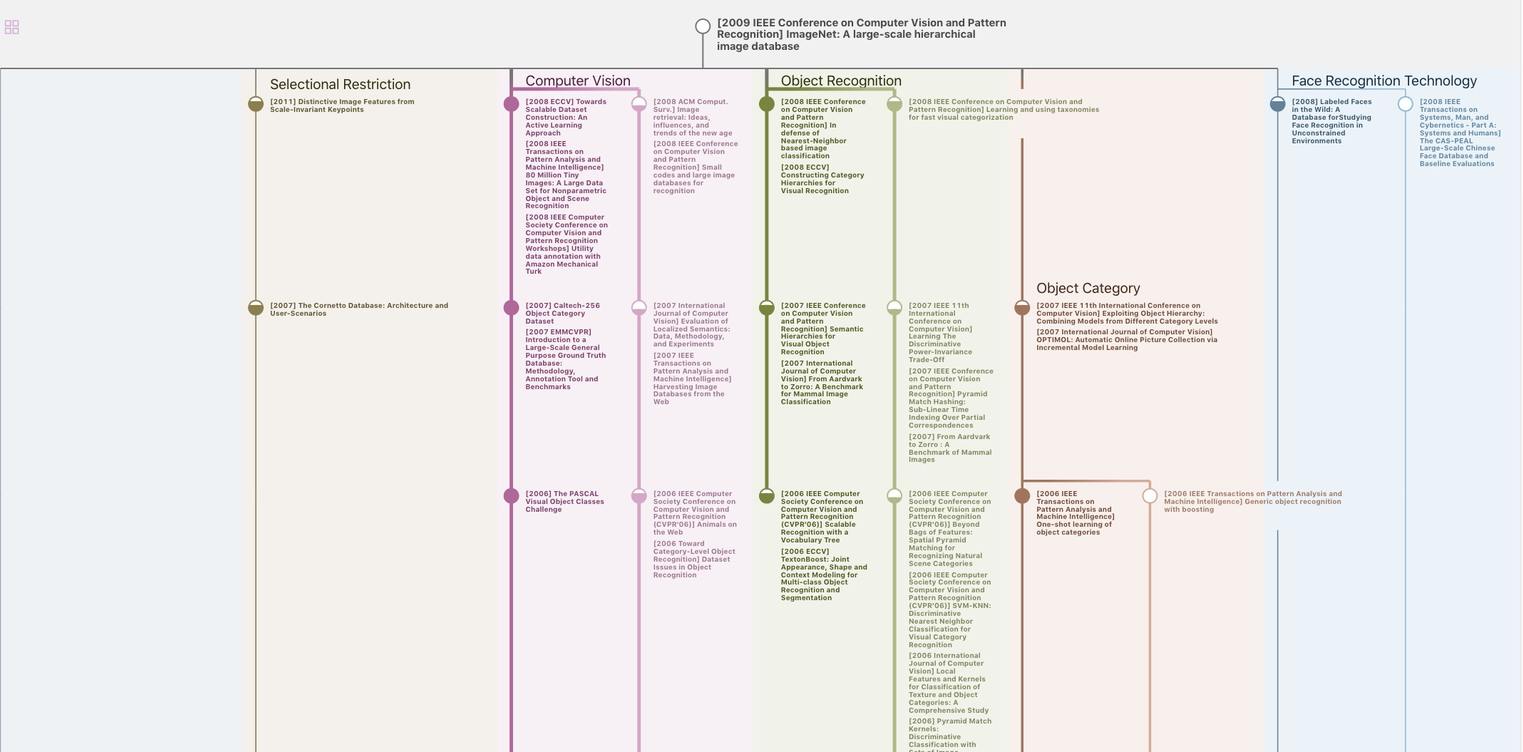
生成溯源树,研究论文发展脉络
Chat Paper
正在生成论文摘要