Evaluating Supervision Levels Trade-Offs for Infrared-Based People Counting
2024 IEEE/CVF Winter Conference on Applications of Computer Vision Workshops (WACVW)(2023)
摘要
Object detection models are commonly used for people counting (and
localization) in many applications but require a dataset with costly bounding
box annotations for training. Given the importance of privacy in people
counting, these models rely more and more on infrared images, making the task
even harder. In this paper, we explore how weaker levels of supervision can
affect the performance of deep person counting architectures for image
classification and point-level localization. Our experiments indicate that
counting people using a CNN Image-Level model achieves competitive results with
YOLO detectors and point-level models, yet provides a higher frame rate and a
similar amount of model parameters.
更多查看译文
关键词
Level Of Supervision,People Counting,Convolutional Network,Convolutional Neural Network,Object Detection,Bounding Box,Training Set,Number Of People,Convolutional Layers,Input Image,Feature Maps,Deep Learning Models,Image Object,Cost-effective Method,Counting Accuracy,Vision Transformer,Query Point,IR Images,Quadtree,Crowd Density,Different Amounts Of Data,Single Shot Detector,Strong Supervision,Pre-training Method,Parameter Count,Perspective Camera,Additional Details,Infrared Imaging,Pixel Coordinates,Benchmark
AI 理解论文
溯源树
样例
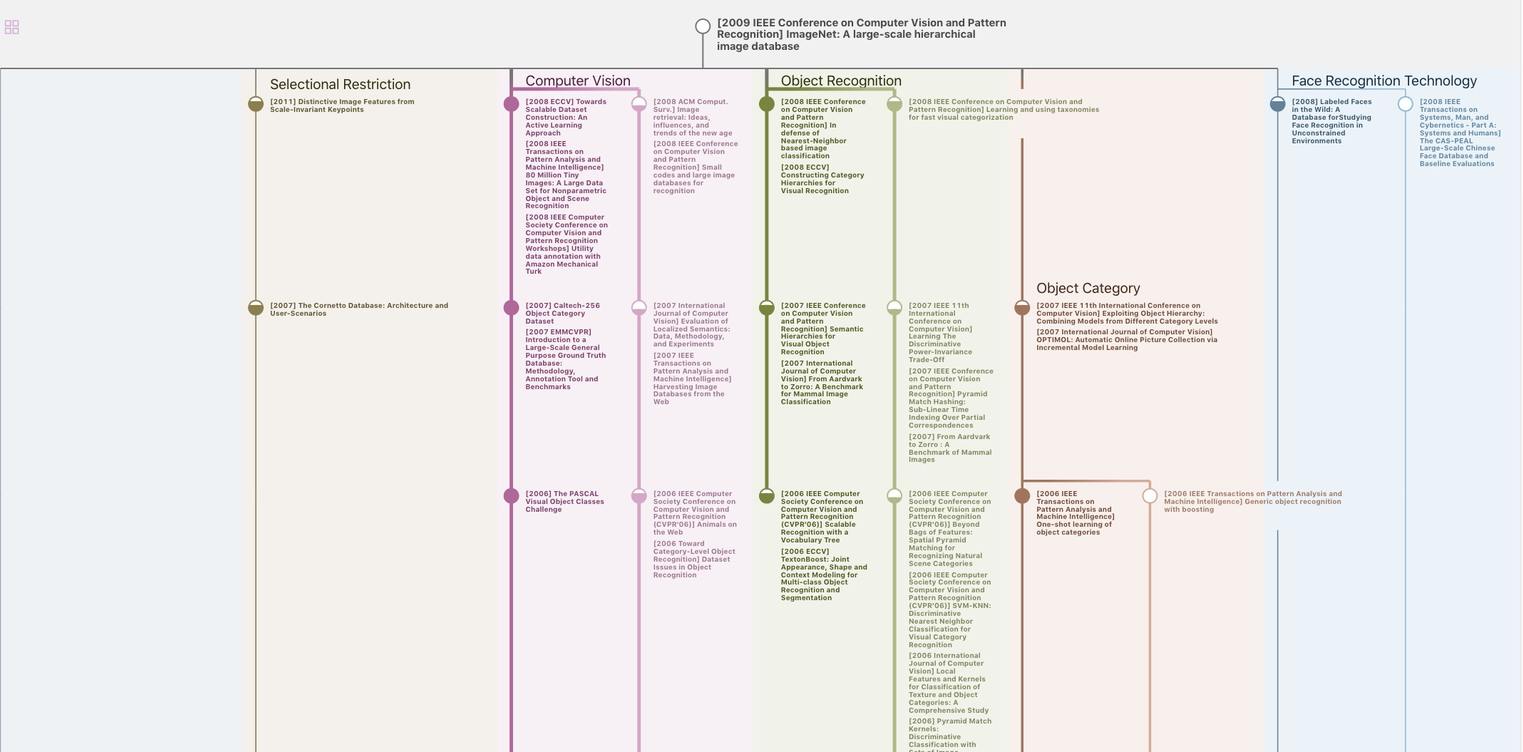
生成溯源树,研究论文发展脉络
Chat Paper
正在生成论文摘要