Physics informed neural networks
Journal of Computational Physics(2023)
摘要
Physics informed neural networks have been recently proposed and offer a new promising method to solve differential equations. They have been adapted to many more scenarios and different variations of the original method have been proposed. In this case study we review many of these variations. We focus on variants that can compensate for imbalances in the loss function and perform a comprehensive numerical comparison of these variants with application to gas transport problems. Our case study includes different formulations of the loss function, different algorithmic loss balancing methods, different optimization schemes and different numbers of parameters and sampling points. We conclude that the original PINN approach with specifically chosen constant weights in the loss function gives the best results in our tests. These weights have been obtained by a computationally expensive random-search scheme. We further conclude for our test case that loss balancing methods which were developed for other differential equations have no benefit for gas transport problems, that the control volume physics informed formulation has no benefit against the initial formulation and that the best optimization strategy is the L-BFGS method. • Physics informed neural networks emerged as a new method to approximate solutions of partial differential equations. • There are many extensions to the original method that are mostly proposed in conjunction with certain differential equations. • There is a lack of comparisons between these extensions and a lack of understanding how they generalize to other problems. • We review many extensions and perform comprehensive numerical tests for a specific application-oriented use case. • We conclude that most extensions provide no benefit and that a random search improves the original method substantially.
更多查看译文
关键词
Physics informed neural network,Multi-criteria optimization,Gas flow,Euler equations,Conservation laws
AI 理解论文
溯源树
样例
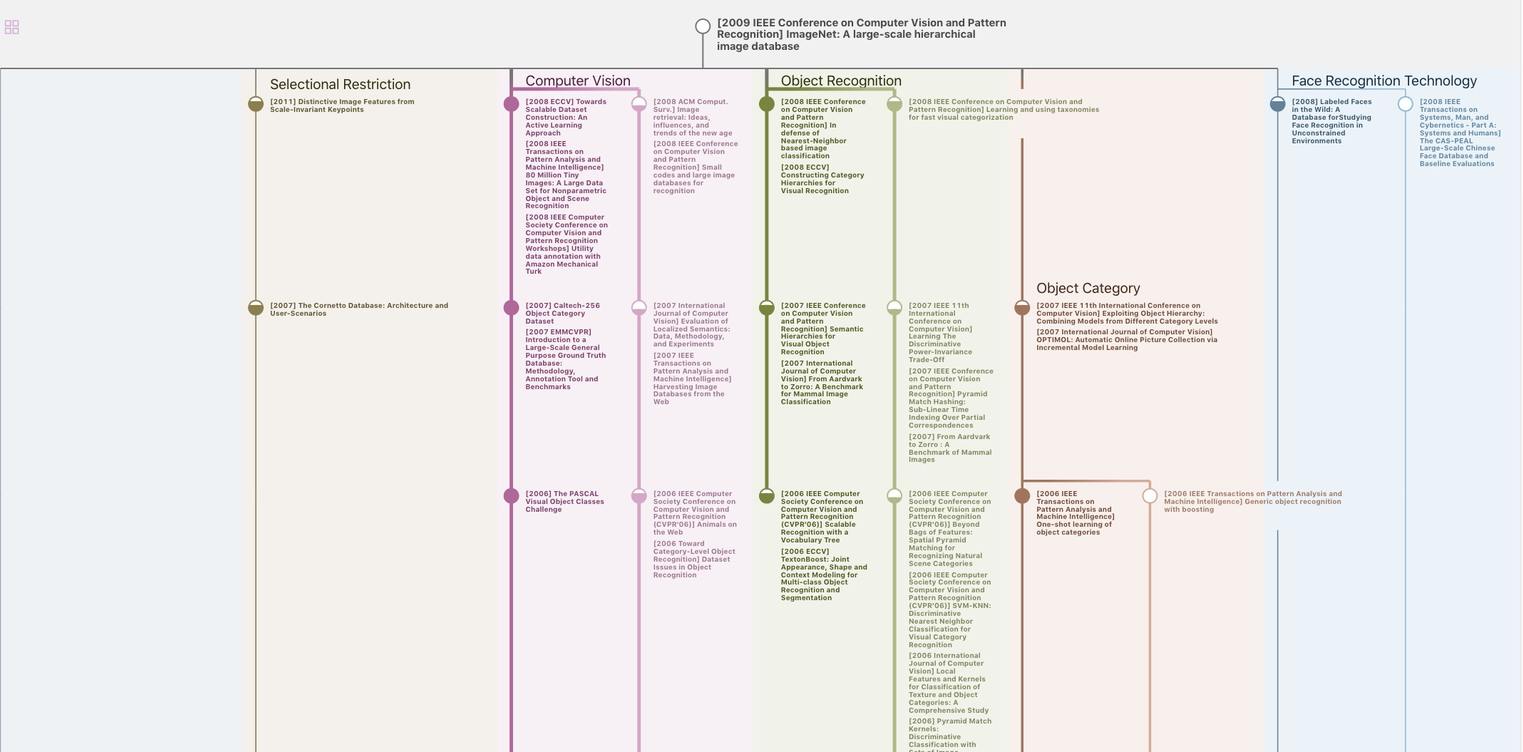
生成溯源树,研究论文发展脉络
Chat Paper
正在生成论文摘要