Contextual classification of polarimetric sar data through a complex-valued kernel and global energy minimization
IGARSS 2023 - 2023 IEEE INTERNATIONAL GEOSCIENCE AND REMOTE SENSING SYMPOSIUM(2023)
摘要
This paper addresses the challenges of supervised semantic segmentation using Polarimetric Synthetic Aperture Radar (PolSAR) data for land cover mapping. We extend previous approaches relying on spatial-contextual classifier based on Support Vector Machines (SVMs) and Markov Random Field (MRF) models. The kernel used in this work extends a previously presented complex formulation based on reproducing kernel Hilbert spaces (RKHS). In this paper, we present a symmetrized form of this complex kernel, integrating it with global energy minimization techniques, and show that it provides more accurate predictions. The proposed approach achieves competitive accuracy on benchmark datasets, comparable to those of deep learning algorithms. The method's advantage lies in its lower resource requirements, making it a promising alternative for PolSAR semantic segmentation.
更多查看译文
关键词
PolSAR,SVM,MRF,complex-valued-kernel,reproducing kernel Hilbert space (RKHS)
AI 理解论文
溯源树
样例
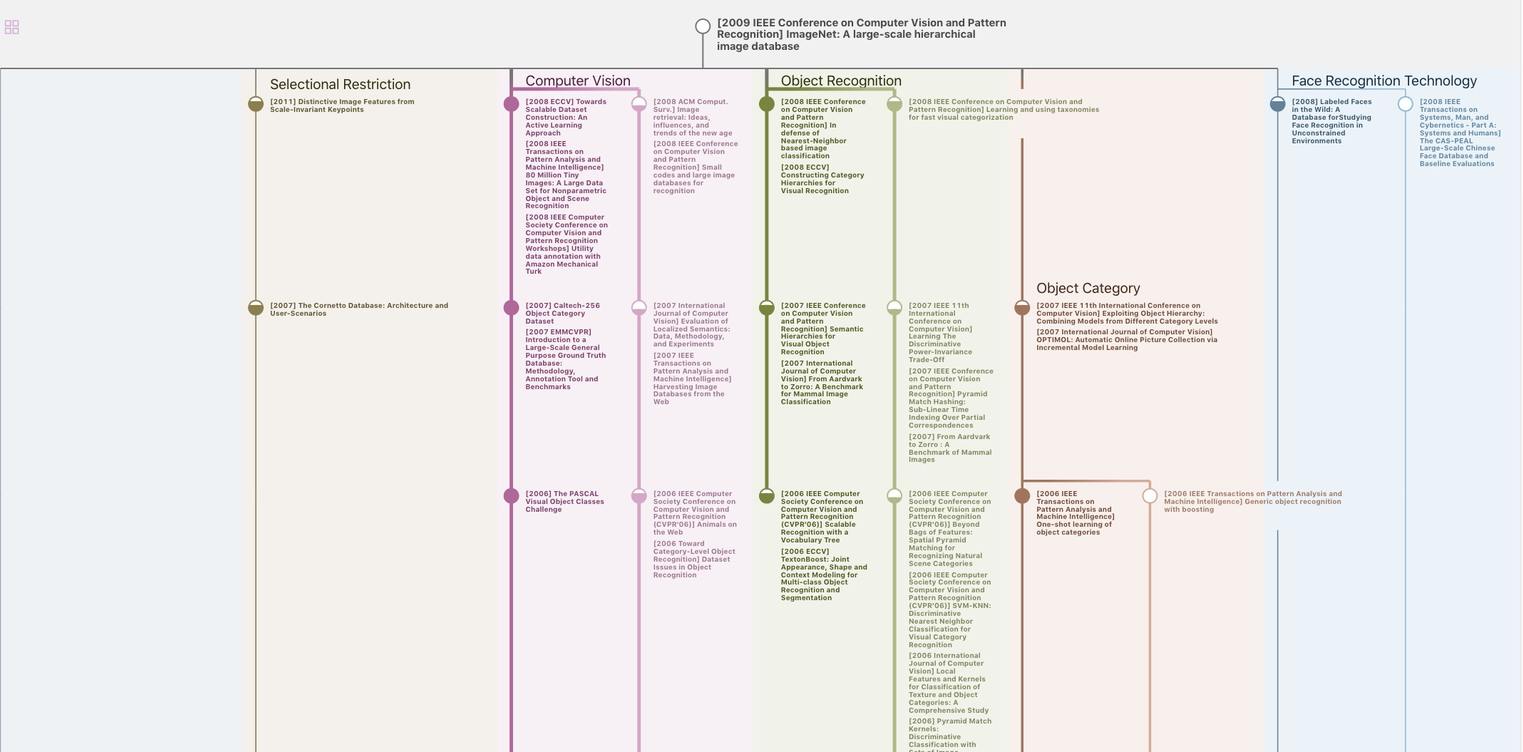
生成溯源树,研究论文发展脉络
Chat Paper
正在生成论文摘要