Region-Aware Multiscale Aggregation Networks for Crowd Counting
2023 8th International Conference on Image, Vision and Computing (ICIVC)(2023)
摘要
Scene complexity and scale variation have long been recognized as common problems in crowd counting. Although deep learning has a good performance in crowd counting, it is still challenging to accurately perform the crowd counting task in scenes with large-scale variations or sheer complexity, and a few objects are often mistaken for people. To address these difficulties, we propose a Region-Aware Multiscale Aggregation Network (RMANet) for crowd counting. On the whole, we design a Fine-Grained Module (FGM). Specifically, FGM consists of an adaptive scale pyramid module (ASPM) and a weight recalibration fusion module (WRFM). ASPM is used to extract contextual features within different regions. WRFM adopts region-aware attention (RAA) to further calibrate the feature weights for different regions. Furthermore, the Multi-scale Aggregation Regression Head Module (MARHM) is introduced to make full use of multi-scale and contextual features extracted from images to generate high-quality crowd density maps and perform accurate count estimation. We implemented extensive experiments on three different challenging datasets, i.e., Shanghai Tech, UCF_CC_50, and UCF-QNRF, and the results demonstrate the effectiveness of the method.
更多查看译文
关键词
Image processing,Crowd counting,Deep learning
AI 理解论文
溯源树
样例
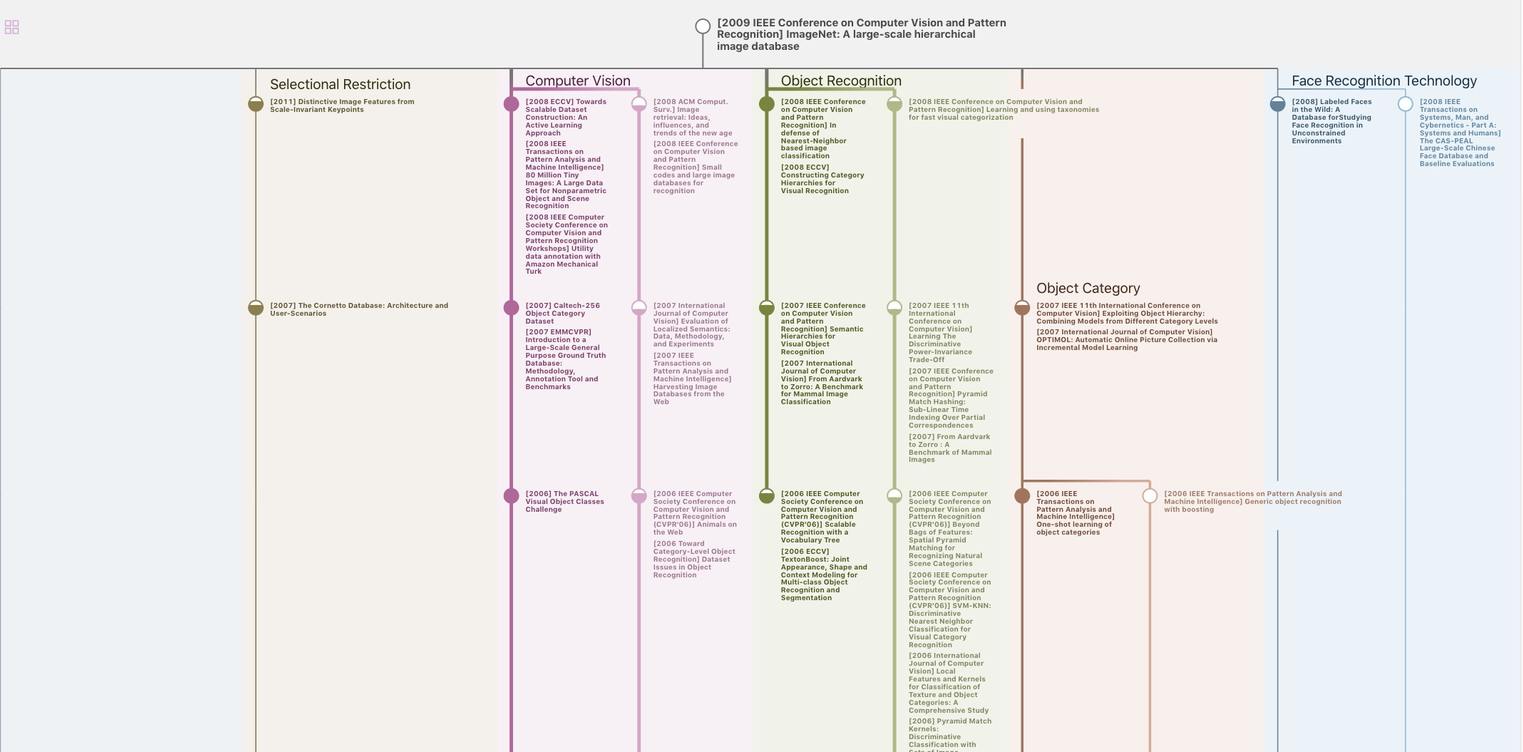
生成溯源树,研究论文发展脉络
Chat Paper
正在生成论文摘要