Space-Optimal Profile Estimation in Data Streams with Applications to Symmetric Functions
CoRR(2023)
摘要
We revisit the problem of estimating the profile (also known as the rarity) in the data stream model. Given a sequence of $m$ elements from a universe of size $n$, its profile is a vector $\phi$ whose $i$-th entry $\phi_i$ represents the number of distinct elements that appear in the stream exactly $i$ times. A classic paper by Datar and Muthukrishan from 2002 gave an algorithm which estimates any entry $\phi_i$ up to an additive error of $\pm \epsilon D$ using $O(1/\epsilon^2 (\log n + \log m))$ bits of space, where $D$ is the number of distinct elements in the stream. In this paper, we considerably improve on this result by designing an algorithm which simultaneously estimates many coordinates of the profile vector $\phi$ up to small overall error. We give an algorithm which, with constant probability, produces an estimated profile $\hat\phi$ with the following guarantees in terms of space and estimation error: - For any constant $\tau$, with $O(1 / \epsilon^2 + \log n)$ bits of space, $\sum_{i=1}^\tau |\phi_i - \hat\phi_i| \leq \epsilon D$. - With $O(1/ \epsilon^2\log (1/\epsilon) + \log n + \log \log m)$ bits of space, $\sum_{i=1}^m |\phi_i - \hat\phi_i| \leq \epsilon m$. In addition to bounding the error across multiple coordinates, our space bounds separate the terms that depend on $1/\epsilon$ and those that depend on $n$ and $m$. We prove matching lower bounds on space in both regimes. Application of our profile estimation algorithm gives estimates within error $\pm \epsilon D$ of several symmetric functions of frequencies in $O(1/\epsilon^2 + \log n)$ bits. This generalizes space-optimal algorithms for the distinct elements problems to other problems including estimating the Huber and Tukey losses as well as frequency cap statistics.
更多查看译文
AI 理解论文
溯源树
样例
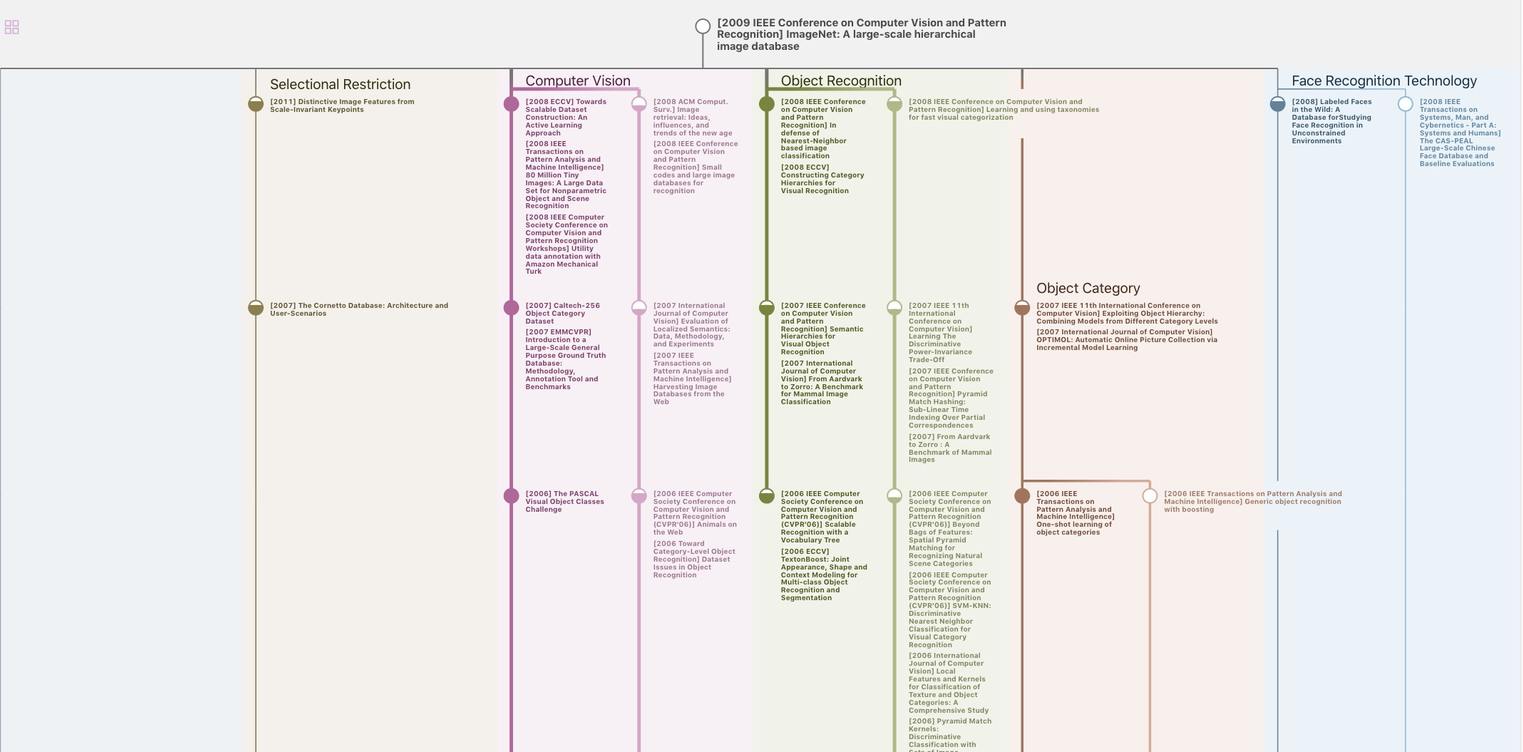
生成溯源树,研究论文发展脉络
Chat Paper
正在生成论文摘要