Efficient Deep Learning on Wearable Physiological Sensor Data for Pilot Flight Performance Analysis
2023 IEEE 19th International Conference on Body Sensor Networks (BSN)(2023)
摘要
With the proliferation of wearable sensors for physiological and cognitive monitoring, a large amount of time series data needs to be processed and analyzed in a timely fashion. While deep learning has shown to be useful for the analysis, the majority of the deep learning methods are computing resource intensive. This paper demonstrates an efficient deep learning approach by adapting MINIROCKET to eye tracking and electrodermal activity data for flight performance assessment. The model was trained on 35 subjects using leave-one-subject-out cross validation and further evaluated on an independent data set of 8 subjects. We performed dimensionality reduction on each time series observation, reducing the size by 99.7% while still achieving averaged Area Under the Curve of 0.912 and average equal error rate of 0.181, thus enabling fast and accurate inference on edge devices. The approach presented here can be implemented in real-world cockpits for near instantaneous performance monitoring and could also be extended beyond this domain to other resource constrained time series applications.
更多查看译文
关键词
deep learning,dimensionality reduction,physiological sensing,performance analysis,wearable sensor
AI 理解论文
溯源树
样例
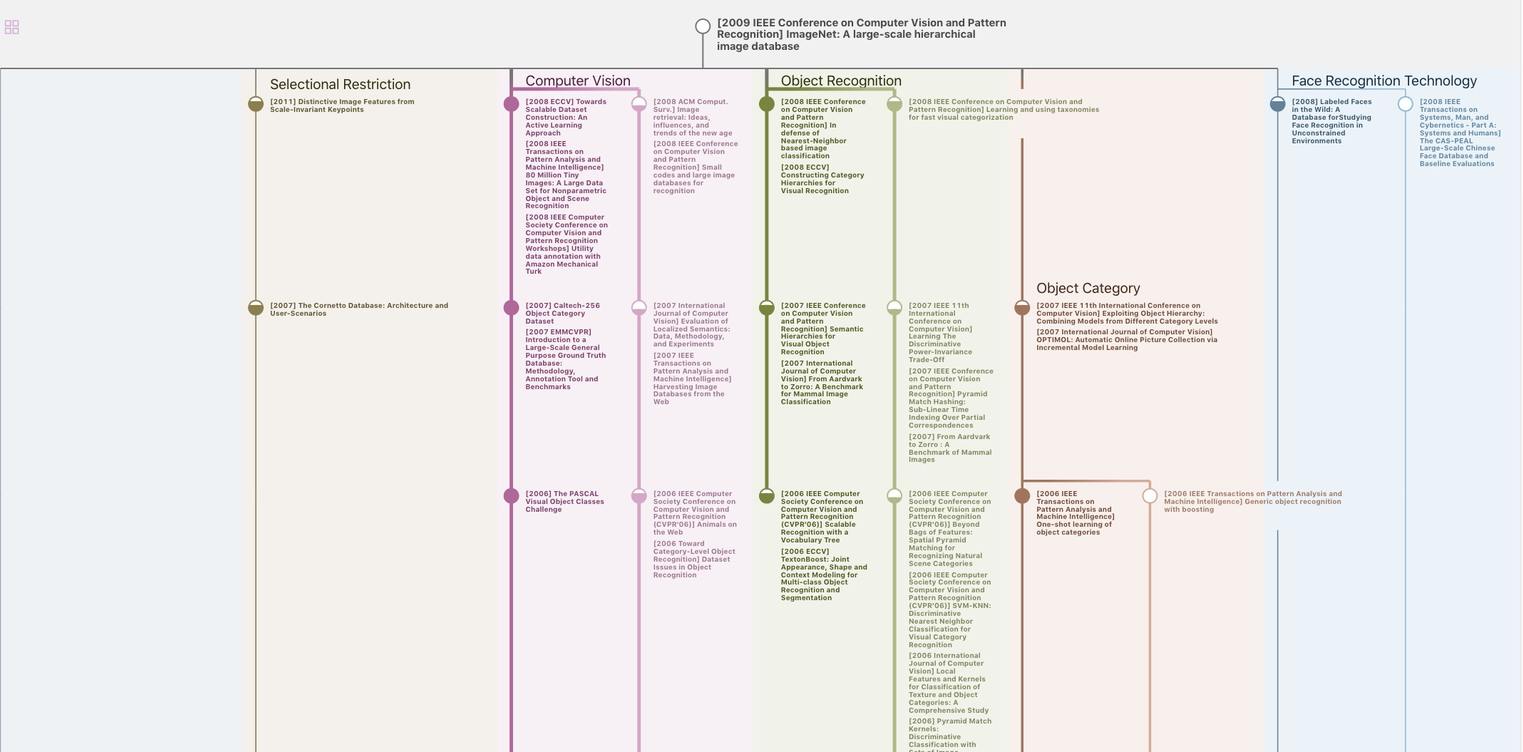
生成溯源树,研究论文发展脉络
Chat Paper
正在生成论文摘要