A Spatio-Temporal Graph Convolutional Network for Gesture Recognition from High-Density Electromyography
arXiv (Cornell University)(2023)
摘要
Accurate hand gesture prediction is crucial for effective upper-limb prosthetic limbs control. As the high flexibility and multiple degrees of freedom exhibited by human hands, there has been a growing interest in integrating deep networks with high-density surface electromyography (HD-sEMG) grids to enhance gesture recognition capabilities. However, many existing methods fall short in fully exploit the specific spatial topology and temporal dependencies present in HD-sEMG data. Additionally, these studies are often limited number of gestures and lack generality. Hence, this study introduces a novel gesture recognition method, named STGCN-GR, which leverages spatio-temporal graph convolution networks for HD-sEMG-based human-machine interfaces. Firstly, we construct muscle networks based on functional connectivity between channels, creating a graph representation of HD-sEMG recordings. Subsequently, a temporal convolution module is applied to capture the temporal dependences in the HD-sEMG series and a spatial graph convolution module is employed to effectively learn the intrinsic spatial topology information among distinct HD-sEMG channels. We evaluate our proposed model on a public HD-sEMG dataset comprising a substantial number of gestures (i.e., 65). Our results demonstrate the remarkable capability of the STGCN-GR method, achieving an impressive accuracy of 91.07% in predicting gestures, which surpasses state-of-the-art deep learning methods applied to the same dataset.
更多查看译文
关键词
Graph convolution networks,gesture recognition,Human-machine interface,high density sEMG,Muscle network
AI 理解论文
溯源树
样例
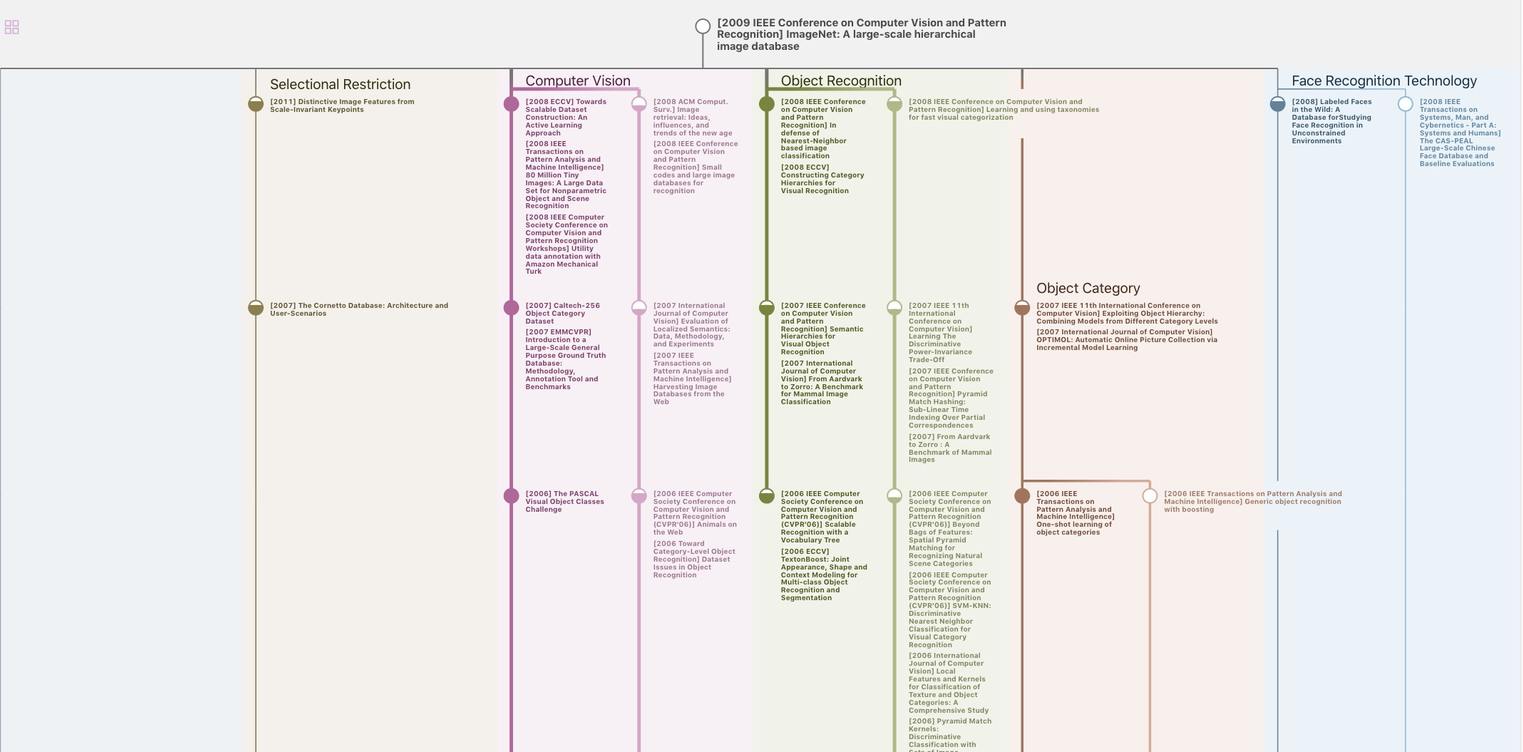
生成溯源树,研究论文发展脉络
Chat Paper
正在生成论文摘要