Extending and Improving Learned CountSketch
arXiv (Cornell University)(2020)
摘要
A sketching algorithm is a way to solve an optimization problem approximately and in a fraction of the usual time. We consider classical sketching algorithms which first compress data by multiplication with a random matrix. Our work improves and extends the paradigm, in which sketch matrices are optimized to yield better expected performance. This technique has only been used for a suboptimal variant of sketched low-rank decomposition (LRD). Our work extends the problem coverage to optimal sketched LRD, least-squares regression (LS), and $k$-means clustering. We improve sketch learning for all three problems and very significantly for LS and LRD: experimental performance increases by $12\%$ and $20\%$, respectively. (Interestingly, we can also prove that we get a strict improvement for LRD under certain conditions.) Finally, we design two sketching algorithm modifications that leverage the strong expected performance of learned sketches, provide worst-case performance guarantees, and have the same time complexity as classical sketching. We prove the worst-case property for each of the problems and their modified algorithms.
更多查看译文
关键词
learned countsketch,improving
AI 理解论文
溯源树
样例
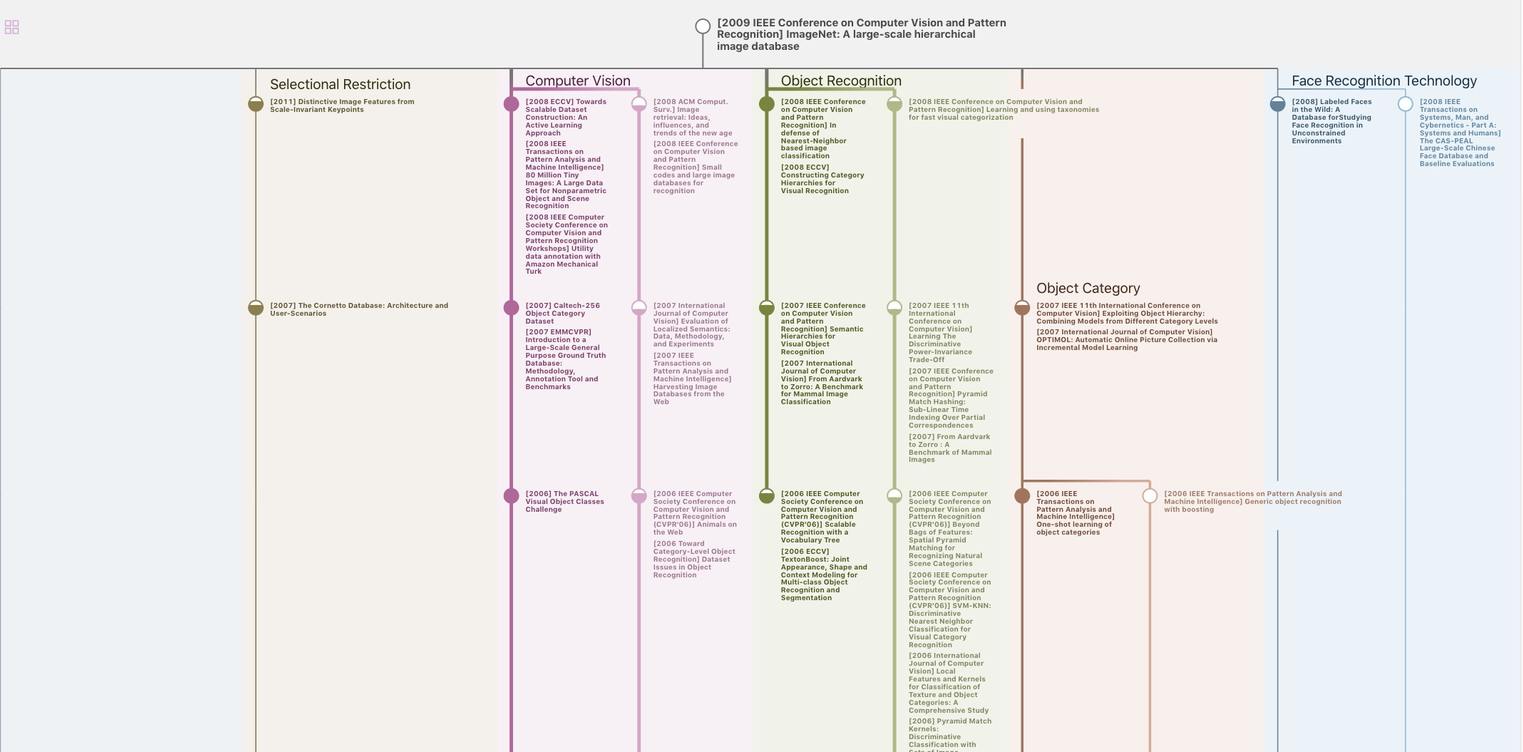
生成溯源树,研究论文发展脉络
Chat Paper
正在生成论文摘要