On the Feasibility of Cross-Task Transfer with Model-Based Reinforcement Learning
arXiv (Cornell University)(2022)
摘要
Reinforcement Learning (RL) algorithms can solve challenging control problems directly from image observations, but they often require millions of environment interactions to do so. Recently, model-based RL algorithms have greatly improved sample-efficiency by concurrently learning an internal model of the world, and supplementing real environment interactions with imagined rollouts for policy improvement. However, learning an effective model of the world from scratch is challenging, and in stark contrast to humans that rely heavily on world understanding and visual cues for learning new skills. In this work, we investigate whether internal models learned by modern model-based RL algorithms can be leveraged to solve new, distinctly different tasks faster. We propose Model-Based Cross-Task Transfer (XTRA), a framework for sample-efficient online RL with scalable pretraining and finetuning of learned world models. By offline multi-task pretraining and online cross-task finetuning, we achieve substantial improvements over a baseline trained from scratch; we improve mean performance of model-based algorithm EfficientZero by 23%, and by as much as 71% in some instances.
更多查看译文
关键词
reinforcement
AI 理解论文
溯源树
样例
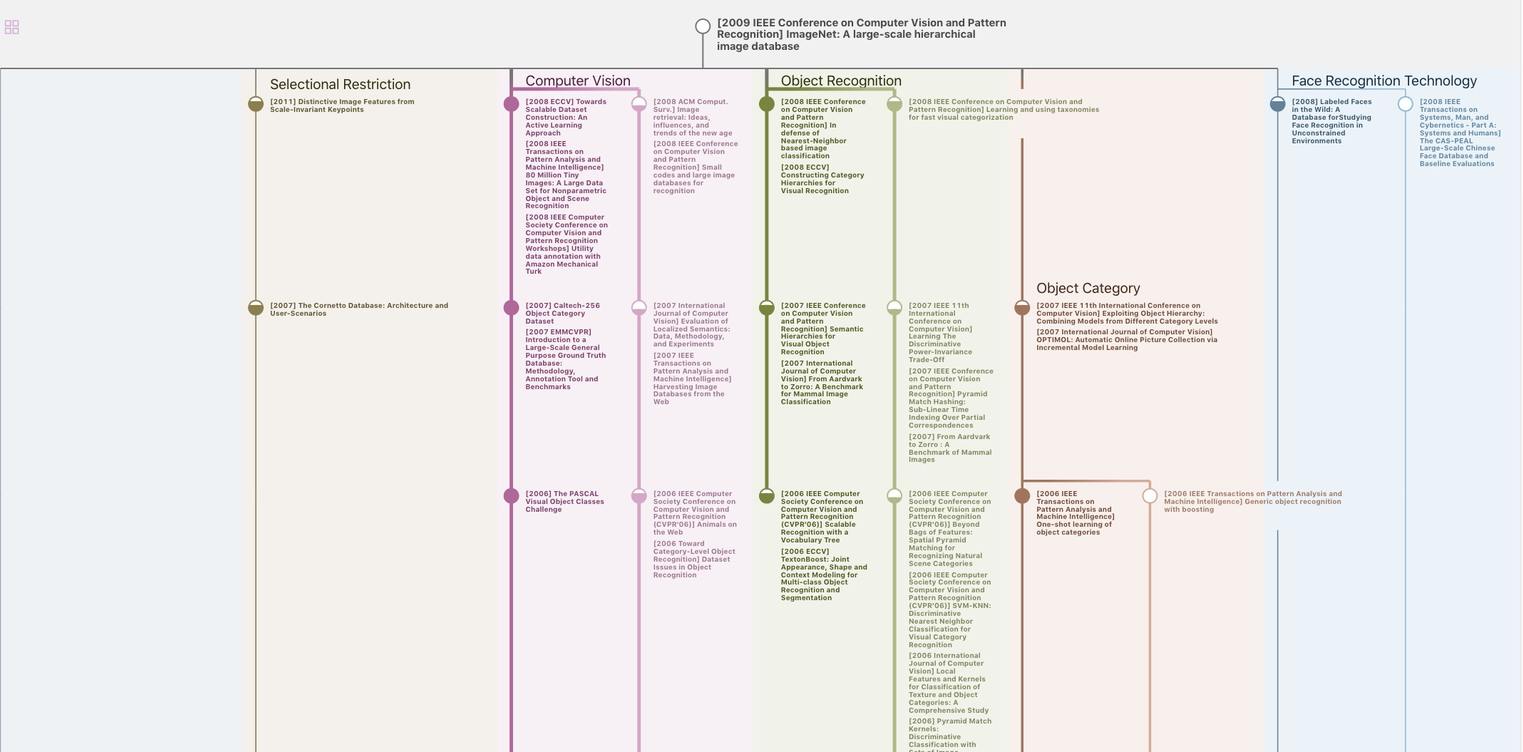
生成溯源树,研究论文发展脉络
Chat Paper
正在生成论文摘要