Semantic-Aware Contrastive Learning With Proposal Suppression for Video Semantic Role Grounding
IEEE TRANSACTIONS ON CIRCUITS AND SYSTEMS FOR VIDEO TECHNOLOGY(2024)
摘要
Video semantic role grounding has gained substantial interest from both the academic and industrial communities. While existing methods have demonstrated considerable performance improvements, the influence of noisy and intra-object proposals, referring to proposals with the same object label, has yet to be explored in video semantic role grounding. In this study, we propose a semantic-aware contrastive learning network with proposal suppression to enhance the accuracy of grounding referenced objects. To fully exploit the semantic information in each semantic role, we introduce a novel semantic role encoding module that allows for precise representations of each semantic role. We also design a semantic-aware proposal suppression network to reduce the impact of noisy proposals on object representation learning. Additionally, we propose a proposal contrastive loss to improve cross-modal alignment and reduce the effect of irrelevant intra-object proposals. Extensive experiments on four datasets demonstrate that our model achieves significant improvements over state-of-the-art methods.
更多查看译文
关键词
Video semantic role grounding,cross-modal retrieval,proposal contrastive learning
AI 理解论文
溯源树
样例
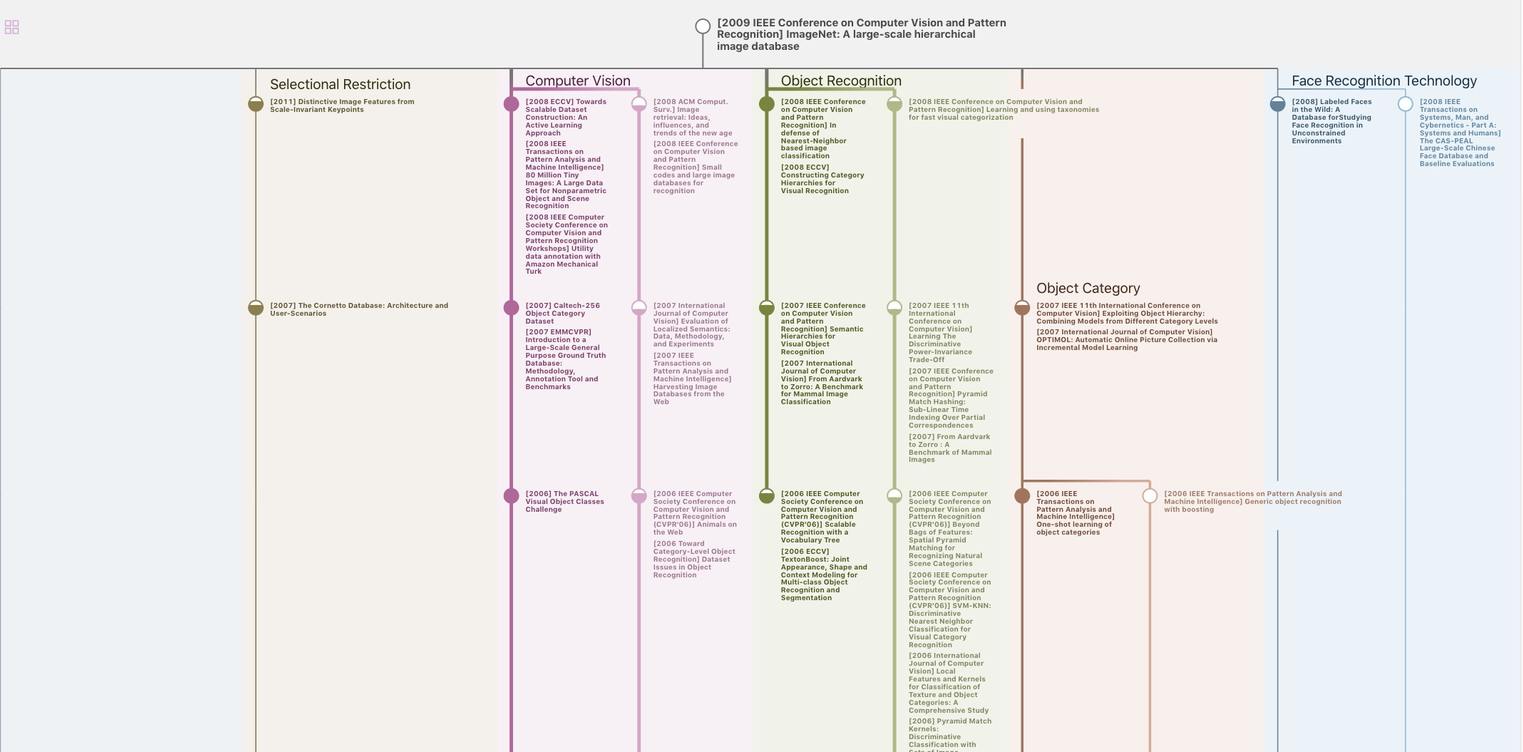
生成溯源树,研究论文发展脉络
Chat Paper
正在生成论文摘要