Erasure Correcting Blind Detection in Unsourced Random Access for Grant-Free Massive Connections
IEEE TRANSACTIONS ON WIRELESS COMMUNICATIONS(2024)
Abstract
The requirement for massive connectivity in 5G and beyond 5G systems pose new challenges to random access protocol design. As a promising technique to afford massive short packet connections, unsourced random access (URA) gains traction. However, the existing URA schemes were developed for static channels and not implementable in fast fading channels. In this paper, we propose a novel URA strategy tailored to time-varying Rayleigh fading channels when the system loads and instantaneous channels are unknown to the receiver. With a time slotted transmission framework, we devise a low-complexity error correcting code and a decoding algorithm leveraging successive interference cancellation (SIC). The receiver corrects errors across slots by using SIC to decode the transmitted messages from highly interfering superposed signals. The asymptotic error rate is derived and a trade-off between the minimum required energy required for reliable communication and the user density is analyzed. Our simulation results verify that the proposed scheme achieves a near-optimal energy efficiency performance when the system load is in the moderate system load regime and a significant performance gain over the existing URA schemes in time varying fading channels.
MoreTranslated text
Key words
Unsourced random access,blind receiver,forward error correction,successive interference cancellation,approximate message passing
求助PDF
上传PDF
View via Publisher
AI Read Science
AI Summary
AI Summary is the key point extracted automatically understanding the full text of the paper, including the background, methods, results, conclusions, icons and other key content, so that you can get the outline of the paper at a glance.
Example
Background
Key content
Introduction
Methods
Results
Related work
Fund
Key content
- Pretraining has recently greatly promoted the development of natural language processing (NLP)
- We show that M6 outperforms the baselines in multimodal downstream tasks, and the large M6 with 10 parameters can reach a better performance
- We propose a method called M6 that is able to process information of multiple modalities and perform both single-modal and cross-modal understanding and generation
- The model is scaled to large model with 10 billion parameters with sophisticated deployment, and the 10 -parameter M6-large is the largest pretrained model in Chinese
- Experimental results show that our proposed M6 outperforms the baseline in a number of downstream tasks concerning both single modality and multiple modalities We will continue the pretraining of extremely large models by increasing data to explore the limit of its performance
Upload PDF to Generate Summary
Must-Reading Tree
Example
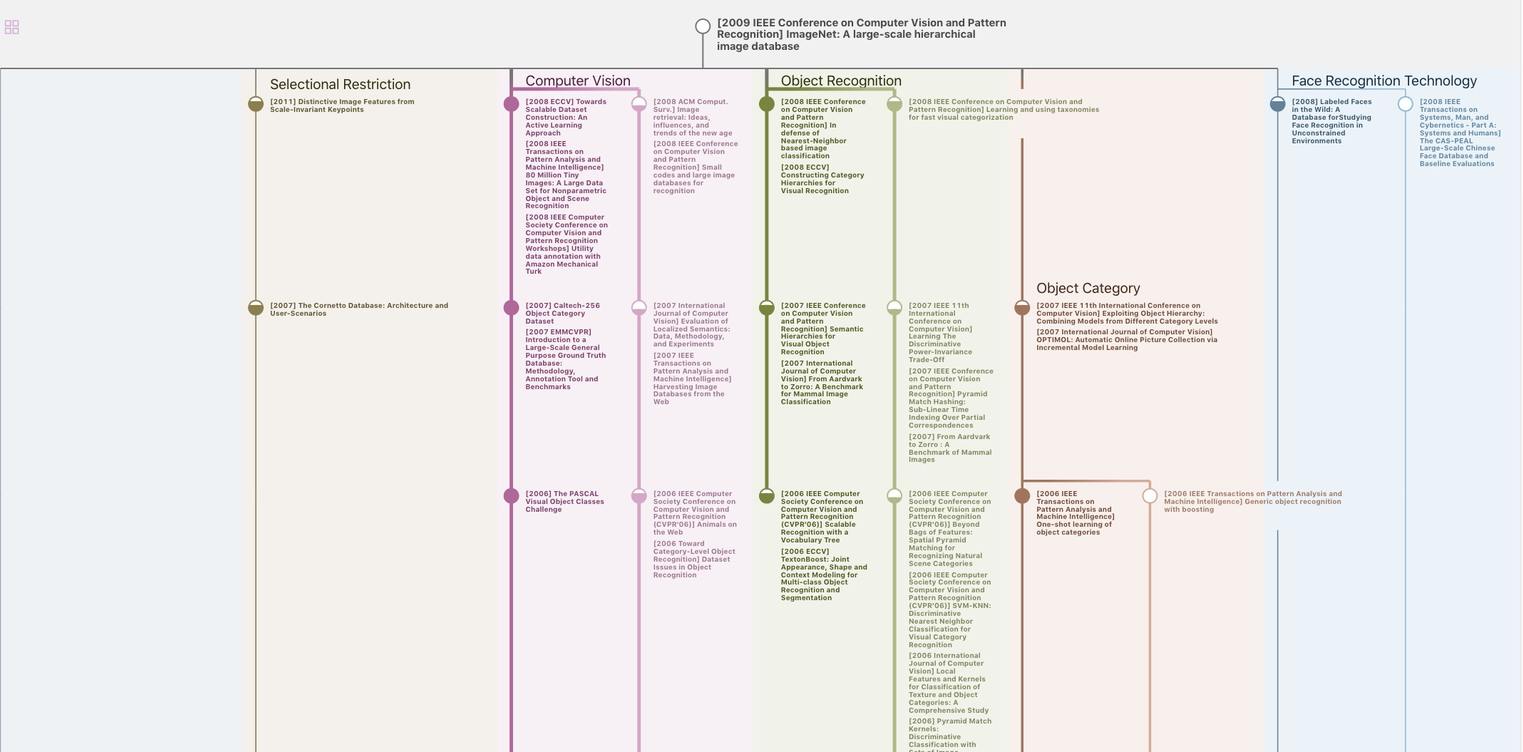
Generate MRT to find the research sequence of this paper
Related Papers
Data Disclaimer
The page data are from open Internet sources, cooperative publishers and automatic analysis results through AI technology. We do not make any commitments and guarantees for the validity, accuracy, correctness, reliability, completeness and timeliness of the page data. If you have any questions, please contact us by email: report@aminer.cn
Chat Paper