Towards automated dermatology triage: deep learning and knowledge-driven approaches
Research Square (Research Square)(2023)
摘要
Abstract Background The current triage process in the National Health Service (NHS) requires secondary care clinicians to manually read every General Practitioner’s (GP) referral letter, which makes the process time-consuming with associated high costs. Artificial Intelligence (AI) algorithms can be adopted to accelerate this process and reduce the required resources. Objectives To design AI models that can automatically stratify GP referrals to routine and non-routine categories, and to evaluate different AI algorithms against the current manual triage process. Methods We developed and evaluated multiple AI models to triage dermatology referrals into binary outcomes, i.e., routine or non-routine. The models ranged from a totally data-driven (deep learning) approach to different levels of knowledge-enriched approaches: 1) a transfer learning approach using a pre-trained large language model; 2) a deep learning model using Long Short-Term Memory architecture, enriched with key concepts from referral guidelines; and 3) a knowledge-driven model utilising the semantics of key concepts from clinical guidelines and customised clinicians’ dictionaries. Random oversampling and data augmentation were used for dealing with highly imbalanced triage classes. All referrals were individually triaged by two dermatologists and then compared against the results generated from AI-assisted triage models. Performances were evaluated using Precision-Recall Area Under Curve (PR-AUC) and Receiver Operating Characteristic Area Under Curve (ROC-AUC). Results 268 GP referrals to adult dermatology services were included. The knowledge-driven approach achieved the best performance (micro average PR-AUC of 0.907±0.006, ROC-AUC of 0.720 ± 0.010) compared to the baseline end-to-end deep learning model (micro average PR-AUC of 0.823±0.038, ROC-AUC of 0.616 ± 0.096) and the Long Short-Term Memory model (0.867±0.013, 0.600 ± 0.071). Imbalance preprocessing methods improved the model performance in some cases but not to a significant level. Combining all types of domain knowledge in AI models outperformed any subsets of these knowledge inputs. Conclusions The knowledge-enhanced AI approach showed promising results in achieving triage outcomes comparable to manual outcomes despite the limited data input from the referrals. AI-assisted triage has the potential to make the triaging process less time-consuming and more cost-effective, whilst retaining accuracy.
更多查看译文
关键词
dermatology triage,deep learning,knowledge-driven
AI 理解论文
溯源树
样例
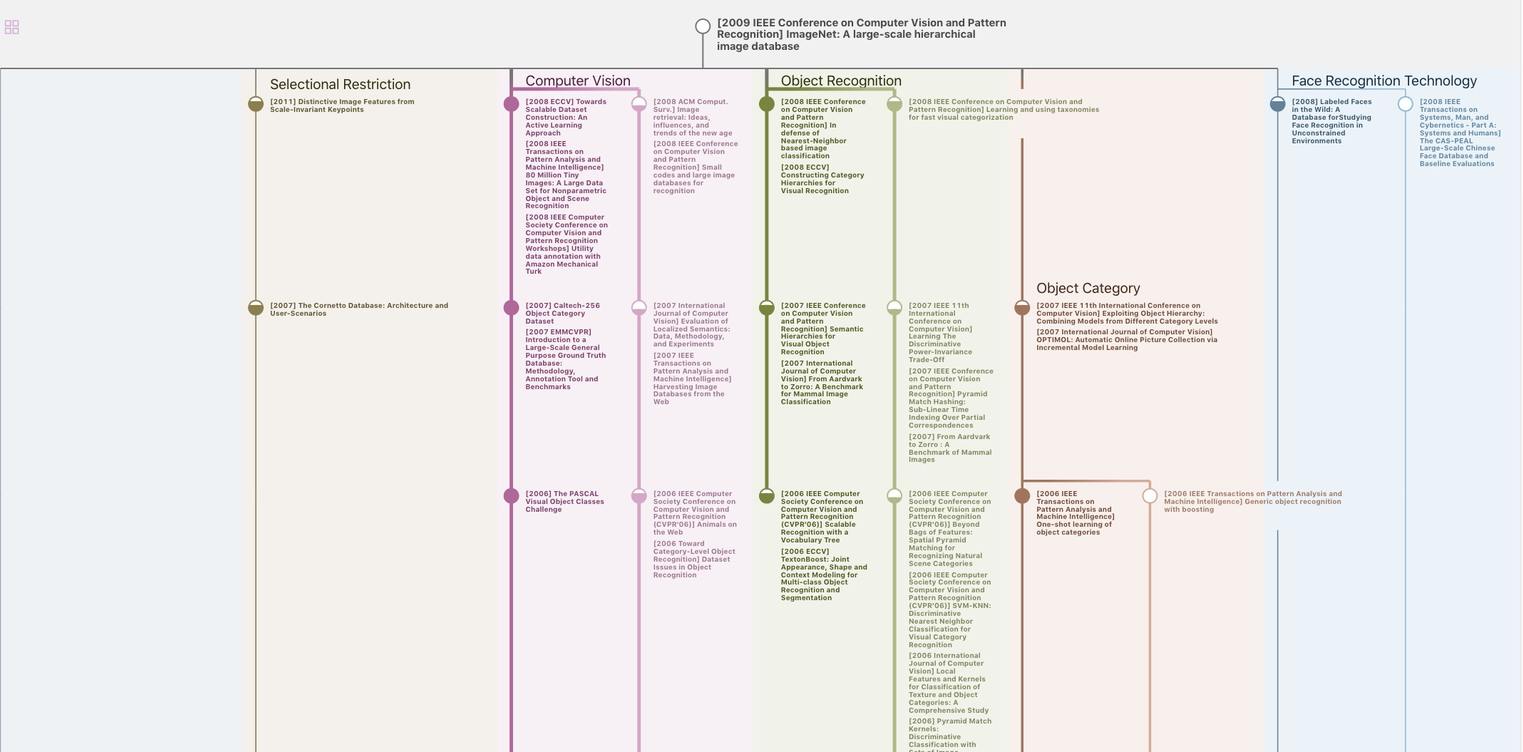
生成溯源树,研究论文发展脉络
Chat Paper
正在生成论文摘要