Learning Shape-Biased Representations for Infrared Small Target Detection
IEEE TRANSACTIONS ON MULTIMEDIA(2024)
摘要
Typically, infrared small target detection aims to accurately localize objects from complex backgrounds where the object textures are often dim and the object shapes are varying. A feasible solution is learning discriminative representations with deep convolutional neural networks (CNNs). However, the representations learned by traditional deep CNNs often suffer from low shape bias. In this work, we propose a unified framework to learn shape-biased representations for facilitating infrared small target detection by explicitly incorporating shape information into model learning. The framework cascades a large-kernel encoder and a shape-guided decoder to learn discriminative shape-biased representations in an end-to-end manner. The large-kernel encoder describes infrared images into shape-preserving representations by using a few convolutions whose kernel size is as large as $9\times 9$, in contrast to commonly used $3\times 3$. The shape-guided decoder simultaneously addresses two tasks: decodes the encoder representations via upsampling reconstruction to reconstruct the segmentation, and hierarchically fuses the decoder representations and edge information via cascaded gated ResNet blocks to reconstruct the contour. In this way, the learned shape-biased representations are effective for identifying infrared small targets. Extensive experiments show our approach outperforms 18 state-of-the-arts.
更多查看译文
关键词
Shape,Object detection,Feature extraction,Decoding,Kernel,Image reconstruction,Task analysis,Infrared small target detection,shape-biased representation,object segmentation,deep learning
AI 理解论文
溯源树
样例
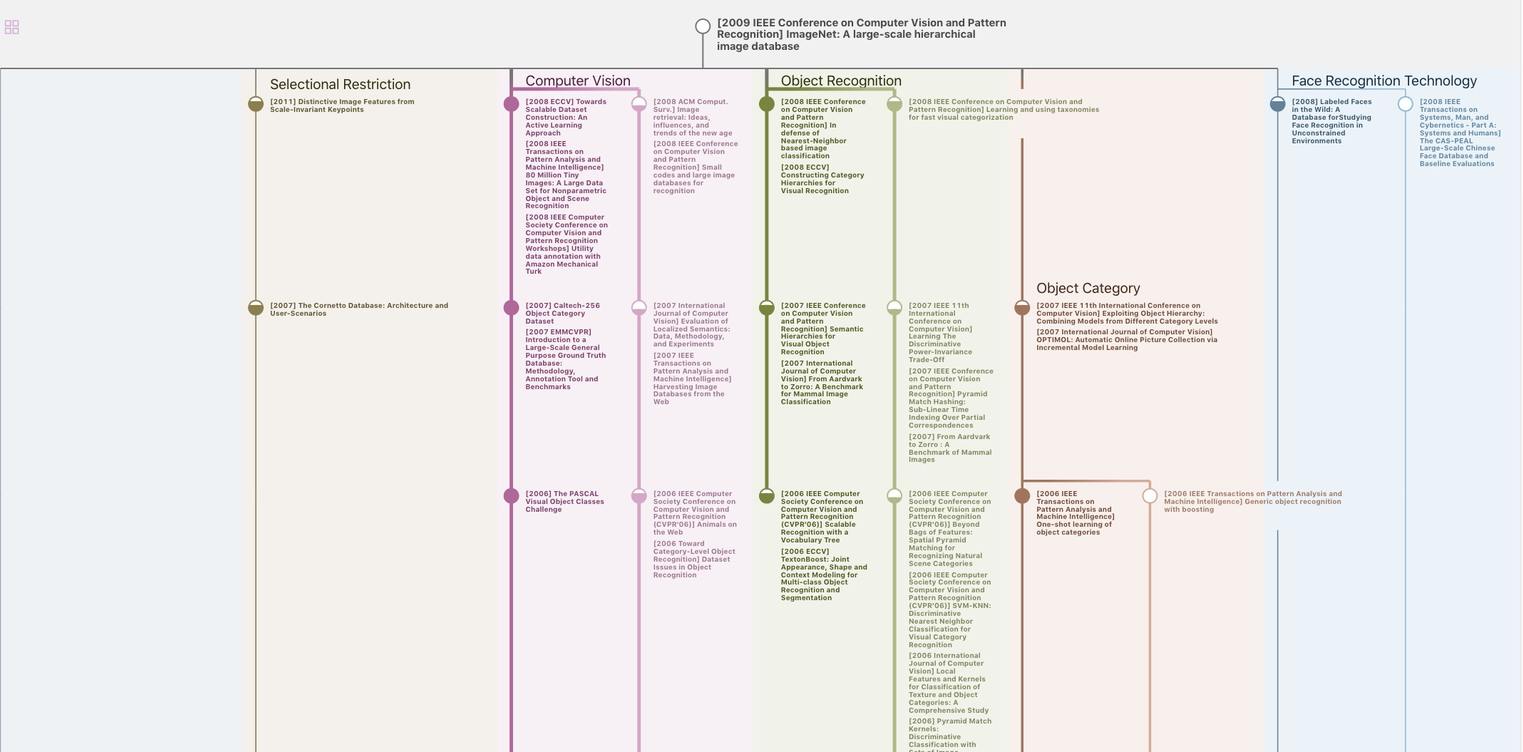
生成溯源树,研究论文发展脉络
Chat Paper
正在生成论文摘要