Interpretable Prediction Rules for Congestion Risk in Intensive Care Units
Stochastic systems(2023)
摘要
We study the problem of predicting congestion risk in intensive care units (ICUs). Congestion is associated with poor service experience, high costs, and poor health outcomes. By predicting future congestion, decision makers can initiate preventive measures, such as rescheduling activities or increasing short-term capacity, to mitigate the effects of congestion. To this end, we consider well-established queueing models of ICUs and define “high-risk states” as system states that are likely to lead to congestion in the near future. We strive to formulate rules for determining whether a given system state is high risk. We design the rules to be interpretable (informally, easy to understand) for their practical appeal to stakeholders. We show that for simple Markovian queueing systems, such as the [Formula: see text] queue with multiple patient classes, our rules take the form of linear and quadratic functions on the state space. For more general queueing systems, we employ methods from queueing theory, simulation, and machine learning (ML) to devise interpretable prediction rules, and we demonstrate their effectiveness through an extensive computational study, which includes a large-scale ICU model validated using data. Our study shows that congestion risk can be effectively and transparently predicted using linear ML models and interpretable features engineered from the queueing model representation of the system. History: This paper has been accepted for the Service Science/Stochastic Systems Joint Special Issue. Supplemental Material: The online appendix is available at https://doi.org/10.1287/stsy.2022.0018 .
更多查看译文
关键词
congestion risk,intensive care units,prediction
AI 理解论文
溯源树
样例
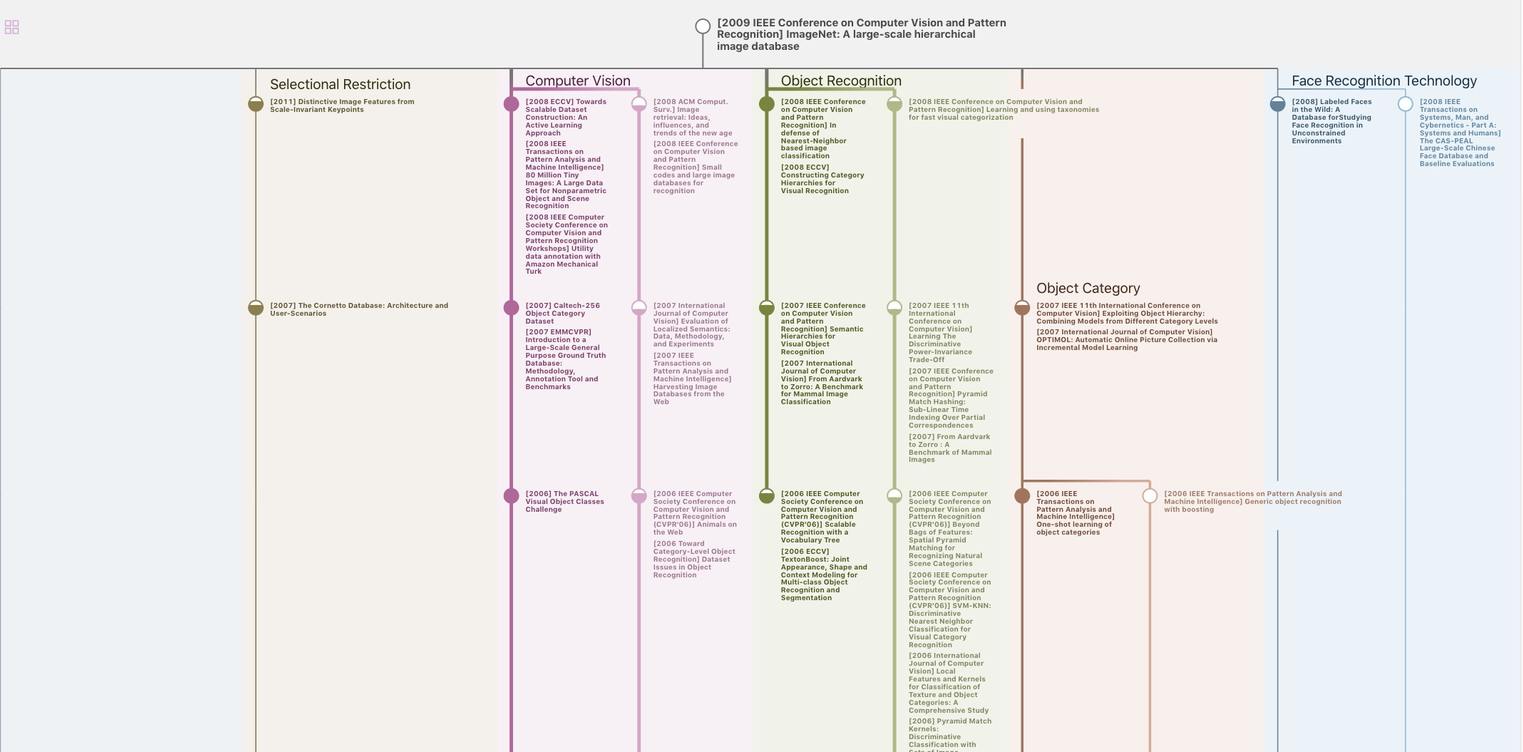
生成溯源树,研究论文发展脉络
Chat Paper
正在生成论文摘要