HiMoDepth: Efficient Training-Free High-Resolution On-Device Depth Perception
IEEE TRANSACTIONS ON MOBILE COMPUTING(2024)
关键词
Efficient,high-resolution,heterogeneous cameras,mobile device
AI 理解论文
溯源树
样例
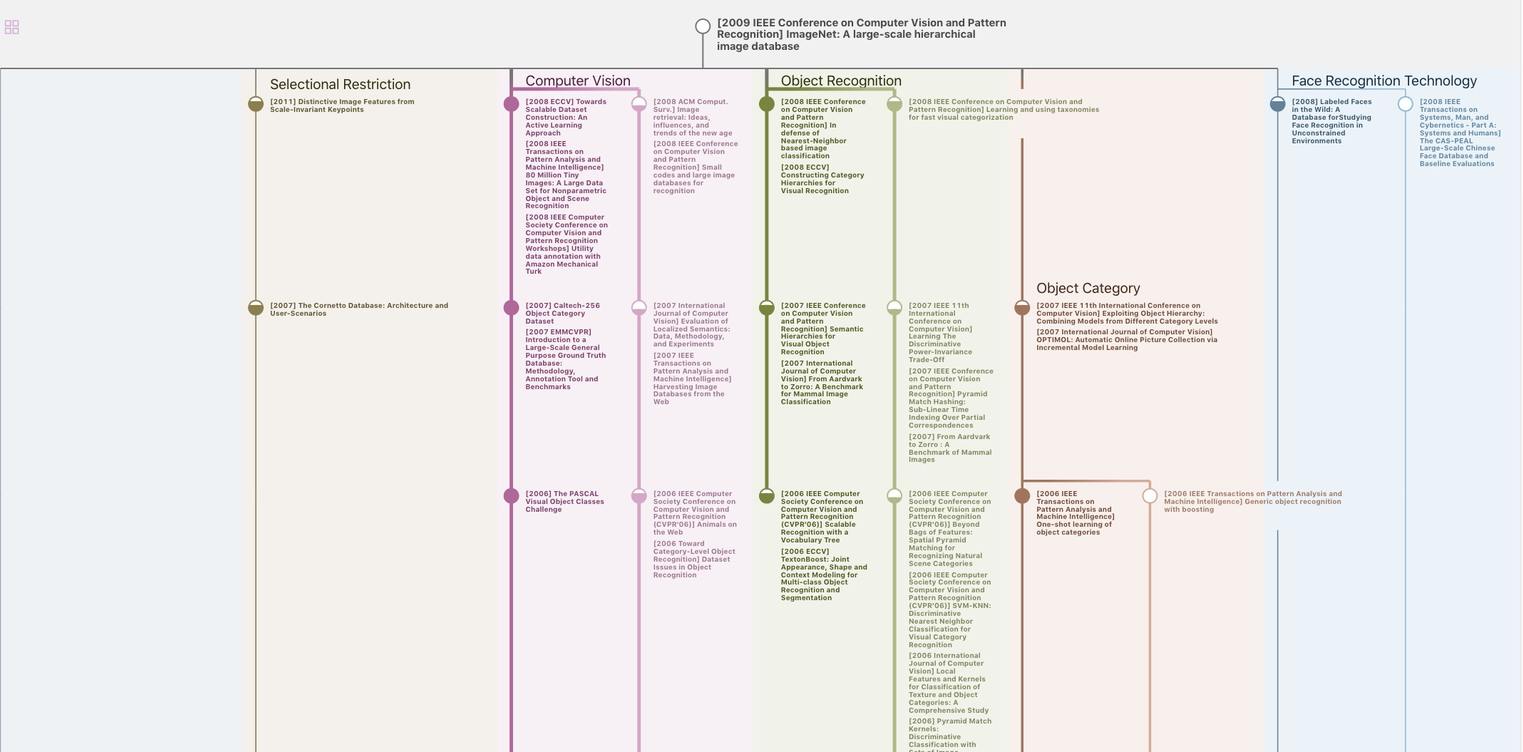
生成溯源树,研究论文发展脉络
Chat Paper
正在生成论文摘要
IEEE TRANSACTIONS ON MOBILE COMPUTING(2024)