LiteVSR: Efficient Visual Speech Recognition by Learning from Speech Representations of Unlabeled Data
CoRR(2023)
摘要
This paper proposes a novel, resource-efficient approach to Visual Speech
Recognition (VSR) leveraging speech representations produced by any trained
Automatic Speech Recognition (ASR) model. Moving away from the
resource-intensive trends prevalent in recent literature, our method distills
knowledge from a trained Conformer-based ASR model, achieving competitive
performance on standard VSR benchmarks with significantly less resource
utilization. Using unlabeled audio-visual data only, our baseline model
achieves a word error rate (WER) of 47.4% and 54.7% on the LRS2 and LRS3 test
benchmarks, respectively. After fine-tuning the model with limited labeled
data, the word error rate reduces to 35% (LRS2) and 45.7% (LRS3). Our model can
be trained on a single consumer-grade GPU within a few days and is capable of
performing real-time end-to-end VSR on dated hardware, suggesting a path
towards more accessible and resource-efficient VSR methodologies.
更多查看译文
关键词
Visual Speech Recognition,Knowledge Distillation,Conformer,Resource Efficiency,Data Efficiency
AI 理解论文
溯源树
样例
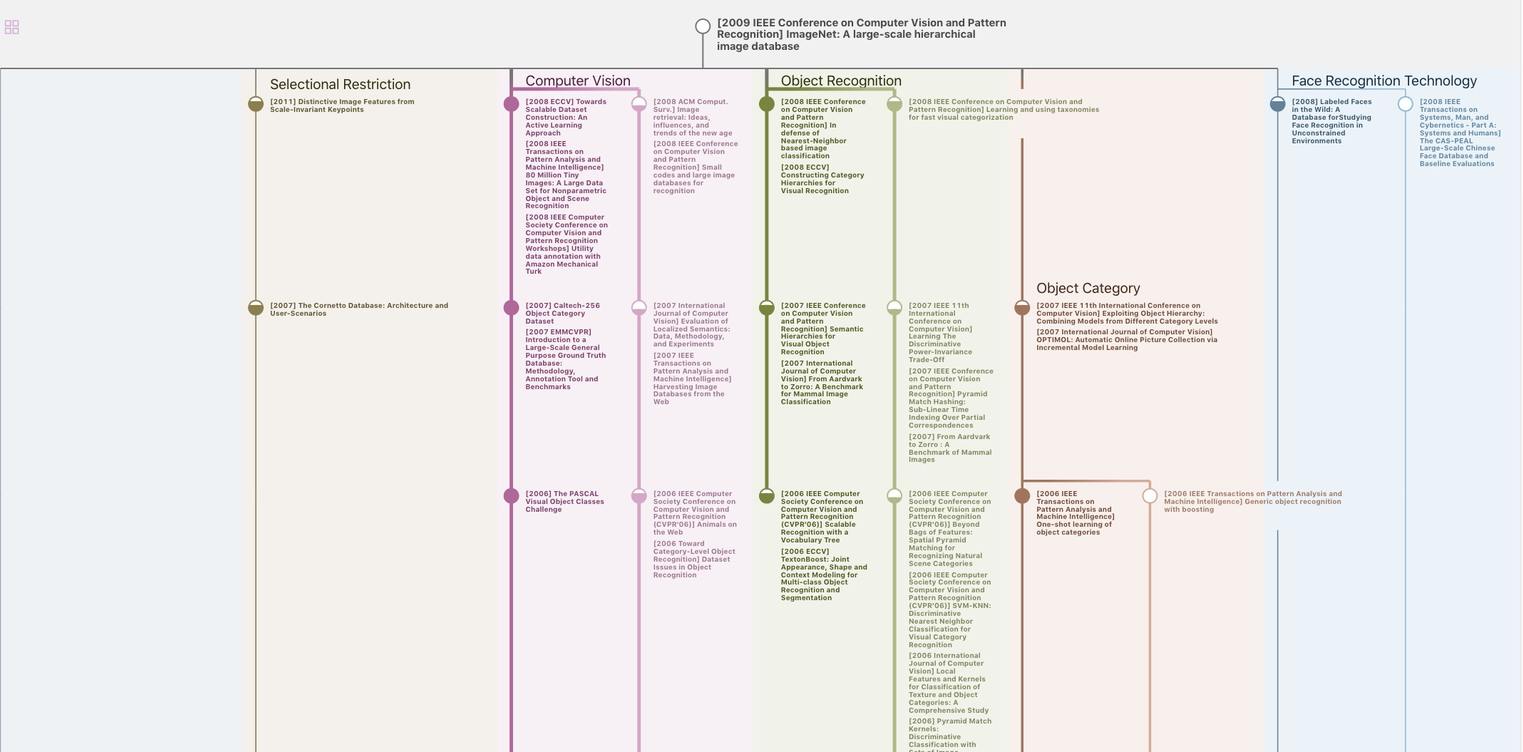
生成溯源树,研究论文发展脉络
Chat Paper
正在生成论文摘要