Fatigue Assessment from Facial Videos using Deep Neural Networks and Engineered Features Informed by Domain Knowledge
2023 45TH ANNUAL INTERNATIONAL CONFERENCE OF THE IEEE ENGINEERING IN MEDICINE & BIOLOGY SOCIETY, EMBC(2023)
摘要
Fatigue impairs cognitive and motor function, potentially leading to mishaps in high-pressure occupations such as aviation and emergency medical services. The current approach is primarily based on self-assessment, which is subjective and error-prone. An objective method is needed to detect severe and likely dangerous levels of fatigue quickly and accurately. Here, we present a quantitative evaluation tool that uses less than two minutes of facial video, captured using an iPad, to assess fatigue vs. alertness. The tool is fast, easy to use, and scalable since it uses cameras readily available on consumer-electronic devices. We compared the classification performance between a Long Short-Term Memory (LSTM) deep neural network and a Random Forest (RF) classifier applied to engineered features informed by domain knowledge. The preliminary results on an 11-subject dataset show that RF outperforms LSTM, with added interpretability on the features used. For the RF classifiers, the average areas under the receiver operating characteristic curve, based on the 11-fold and individualized 11-fold cross validations, are 0.72 +/- 0.16 and 0.8 +/- 0.12, respectively. Equal error rates are 0.34 and 0.26, respectively. This study presents a promising approach for rapid fatigue detection. Additional data will be collected to assess the generalizability across populations.
更多查看译文
AI 理解论文
溯源树
样例
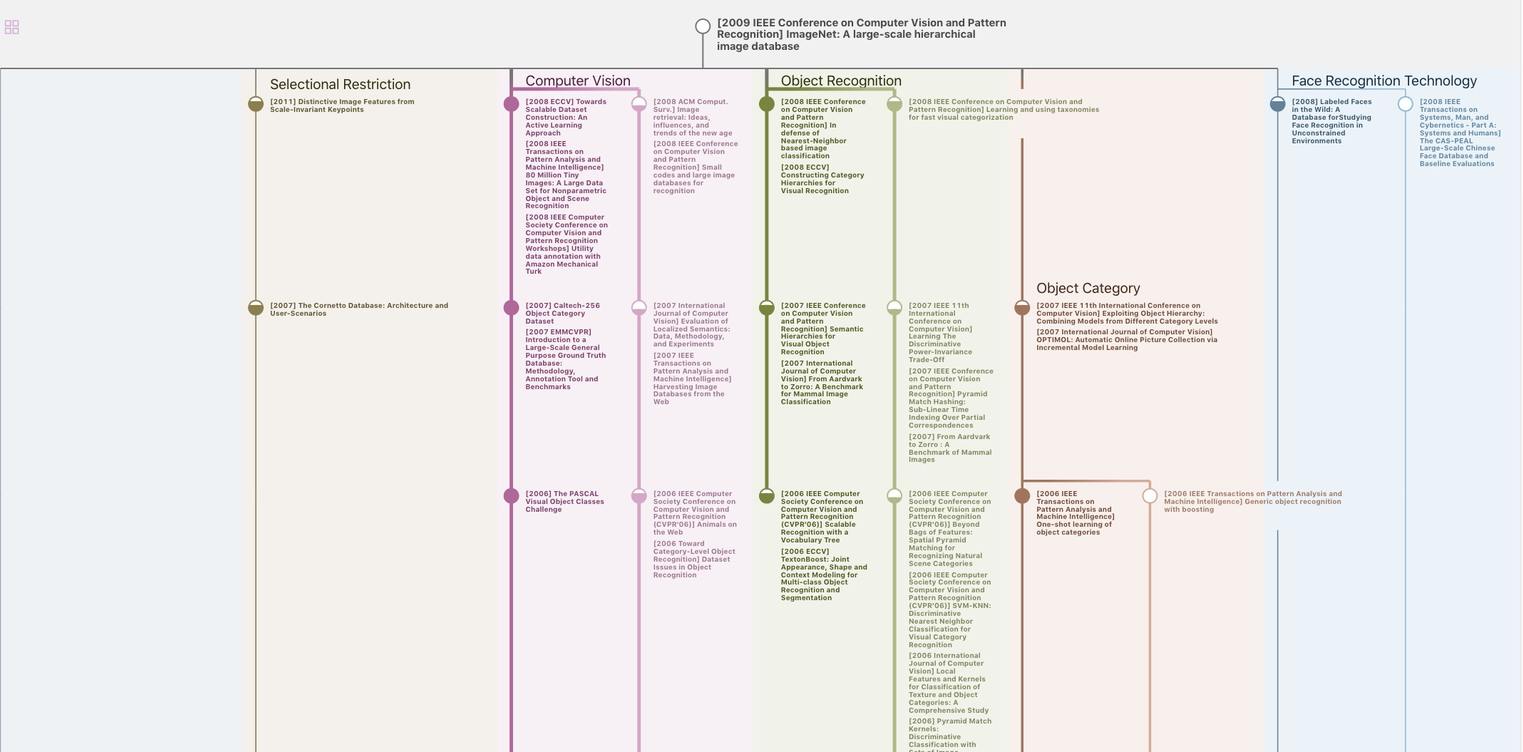
生成溯源树,研究论文发展脉络
Chat Paper
正在生成论文摘要