Integrating Multi-Demonstration Knowledge and Bounded Workspaces for Efficient Deep Reinforcement Learning
2023 IEEE-RAS 22ND INTERNATIONAL CONFERENCE ON HUMANOID ROBOTS, HUMANOIDS(2023)
摘要
We propose a novel approach for boosting deep Reinforcement Learning (RL) using human demonstrations and offline workspace bounding. Our approach involves collecting data from human demonstrations on random surfaces with varying friction and stiffness properties. We then compute a 3D convex hull that encompasses all the paths taken by the demonstrators. By defining the task and the desired parameters as reward functions, we enable the reinforcement learning agent to learn an optimal solution within the bounded space, significantly reducing the search space required for the agent. We compare the training progress and the behavior of the trained policy of our approach with a baseline approach. The results demonstrate that our approach not only expedites learning but also improves the policy's performance and resilience to local minima. Combining our approach with RL also enables the use of imperfect demonstrators as their behavior can be improved during the learning. Our approach has the potential to significantly boost the development of deep RL applications in various domains, including robotics, gaming, and autonomous systems.
更多查看译文
关键词
Deep reinforcement learning,Offline workspace bounding,Contact-rich path following,Human demonstrations
AI 理解论文
溯源树
样例
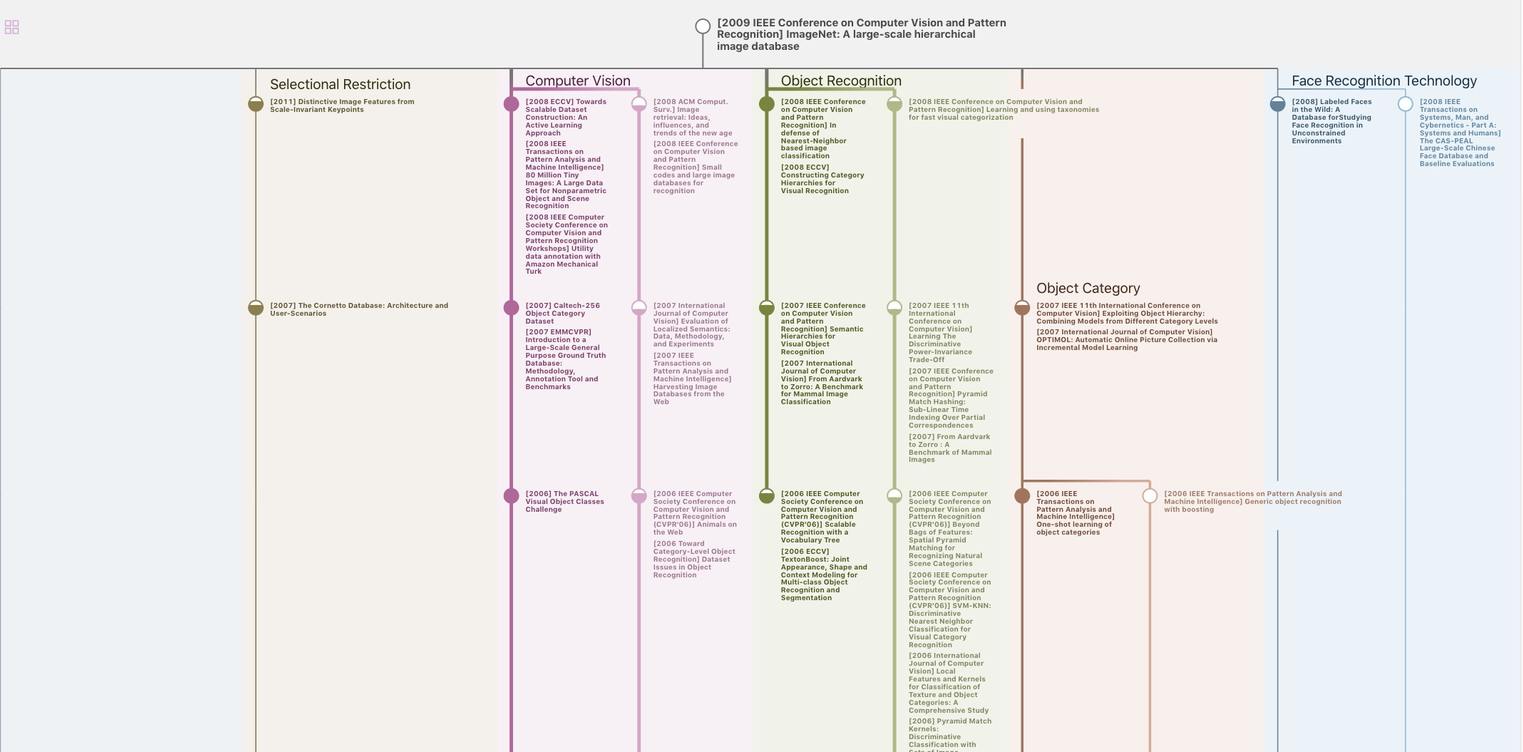
生成溯源树,研究论文发展脉络
Chat Paper
正在生成论文摘要