Knowing is Half the Battle: Enhancing Clean Data Accuracy of Adversarial Robust Deep Neural Networks via Dual-Model Bounded Divergence Gating
IEEE ACCESS(2024)
摘要
Significant advances have been made in recent years in improving the robustness of deep neural networks, particularly under adversarial machine learning scenarios where the data has been contaminated to fool networks into making undesirable predictions. However, such improvements in adversarial robustness has often come at a significant cost in model accuracy when dealing with uncontaminated data (i.e., clean data), making such defense mechanisms challenging to adapt for real-world practical scenarios where data is primarily clean and accuracy needs to be high. Motivated to find a better balance between adversarial robustness and clean data accuracy, we propose a new model-agnostic adversarial defense mechanism named Dual-model Bounded Divergence (DBD), driven by a theoretical and empirical analysis of the bias-variance trade-off within an adversarial machine learning context. More specifically, the proposed DBD mechanism is premised on the observation that the variance in deep neural networks tends to increase in the presence of adversarial perturbations in the input data. As such, DBD employs a gating mechanism to decide on the final model prediction output based on a novel dual-model variance measure (coined DBD Variance), which is a bounded version of KL-Divergence between models. Not only is the proposed DBD mechanism itself training-free, but it can be combined with existing adversarial defense mechanisms to boost the balance between clean data accuracy and adversarial robustness. Comprehensive experimental results across over 10 different state-of-the-art adversarial defense mechanisms using both CIFAR-10 and ImageNet benchmark datasets across different adversarial attacks (e.g., APGD, AutoAttack) demonstrates that the integration of DBD can lead to as much as a 6% improvement on clean data accuracy without compromising much on adversarial robustness.
更多查看译文
关键词
Adversarial attack,computer vision,deep learning,neural networks
AI 理解论文
溯源树
样例
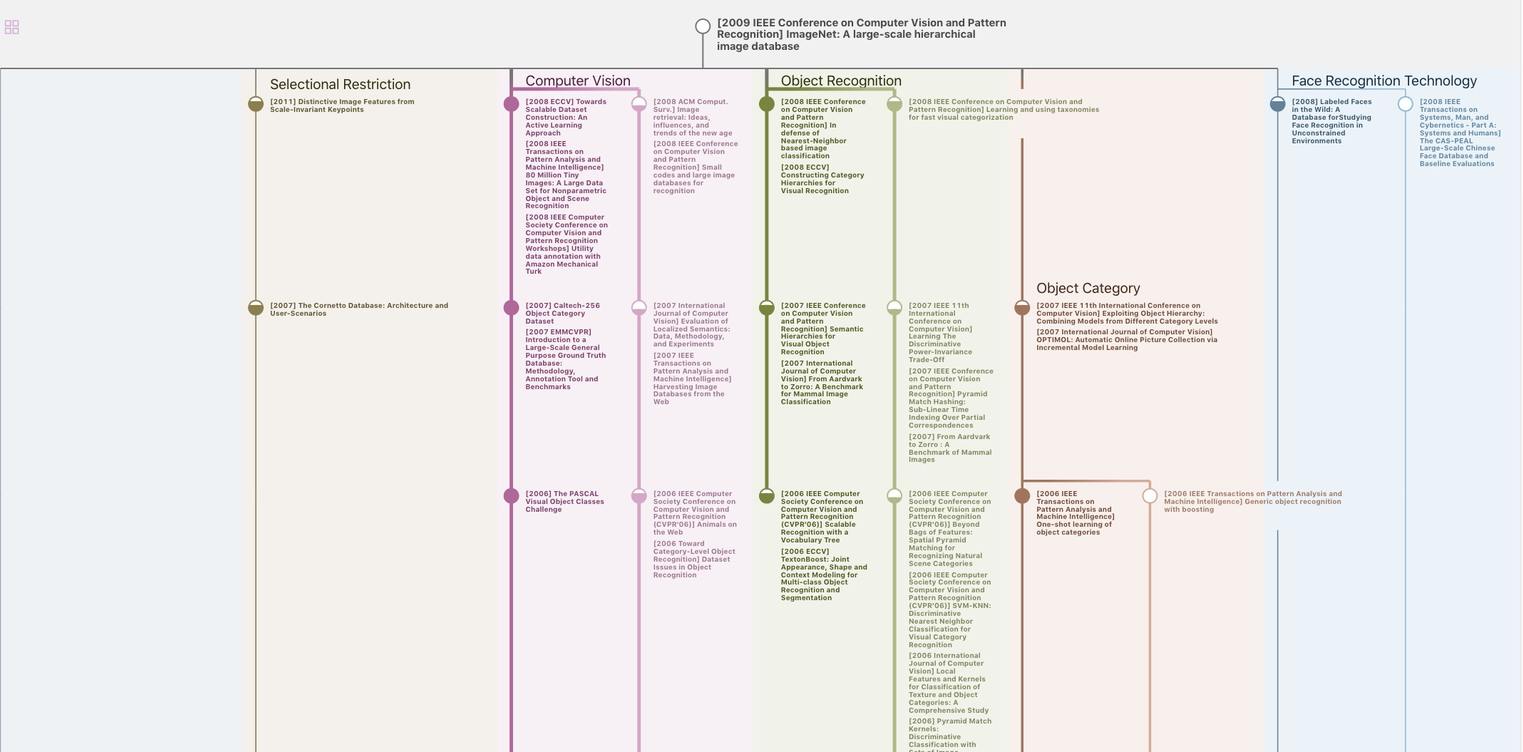
生成溯源树,研究论文发展脉络
Chat Paper
正在生成论文摘要