Individual mapping and asymmetric dual supervision for discrete cross-modal hashing
EXPERT SYSTEMS WITH APPLICATIONS(2024)
摘要
Cross -modal hashing has gained popularity in similarity search due to its excellent query efficiency and economical storage costs. However, current models frequently overlook the distinctive property of each modality, resulting in reduced accuracy due to inadequate utilization of these attributes. Moreover, there is a weak semantic relevance between modality attributes and multiple supervision knowledge (the labels and similarity constraints constructed by labels), accompanied by a cumulative quantization of the models. To address these issues, we propose an Individual Mapping and Asymmetric Dual Supervision method (IMADS). It merges specific and shared information to effectively learn a cross -modal representation space. Furthermore, we present an asymmetric dual supervision learning framework to produce discriminative hash codes. This framework achieves two primary goals: (1) Combing cross -modal representation and multiple supervision information to enhance the consistent relation of distinct modalities, and (2) developing a discrete optimization algorithm to mitigate the information loss caused by the hash code. Comprehensive experimental results illustrate that the introduced IMADS outperforms other stat-of-the-art hashing methods.
更多查看译文
关键词
Asymmetric dual supervision,Cross-modal hashing,Individual mapping,Optimization algorithm
AI 理解论文
溯源树
样例
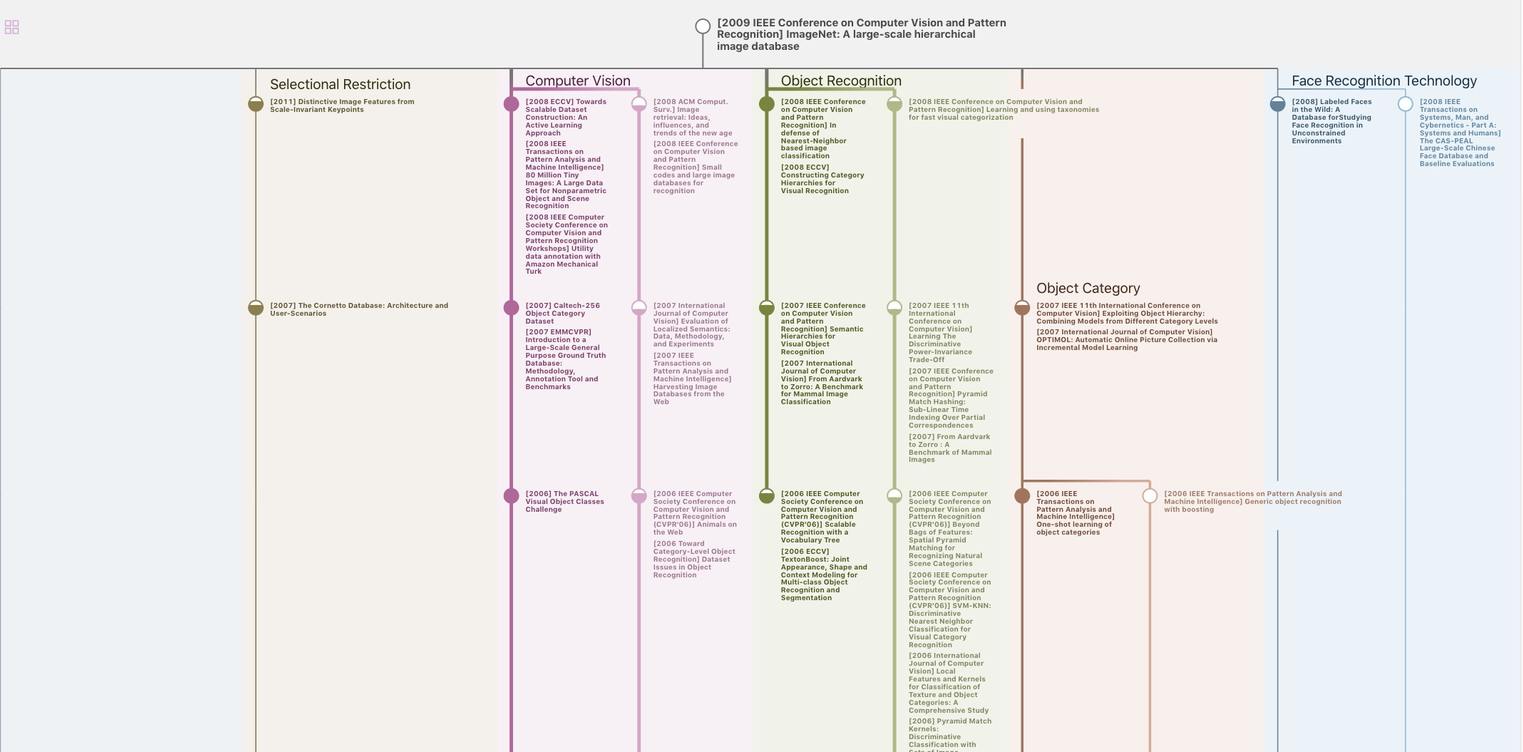
生成溯源树,研究论文发展脉络
Chat Paper
正在生成论文摘要