CascadedGaze: Efficiency in Global Context Extraction for Image Restoration
TMLR 2024(2024)
Researcher | Principal Researcher | Full Professor
Abstract
Image restoration tasks traditionally rely on convolutional neural networks. However, given the local nature of the convolutional operator, they struggle to capture global information. The promise of attention mechanisms in Transformers is to circumvent this problem, but it comes at the cost of intensive computational overhead. Many recent studies in image restoration have focused on solving the challenge of balancing performance and computational cost via Transformer variants. In this paper, we present CascadedGaze Network (CGNet), an encoder-decoder architecture that employs Global Context Extractor (GCE), a novel and efficient way to capture global information for image restoration. The GCE module leverages small kernels across convolutional layers to learn global dependencies, without requiring self-attention. Extensive experimental results show that our approach outperforms a range of state-of-the-art methods on denoising benchmark datasets including both real image denoising and synthetic image denoising, as well as on image deblurring task, while being more computationally efficient.
MoreTranslated text
Key words
Image Retrieval,Image Segmentation,Image Annotation,Object Recognition
PDF
View via Publisher
AI Read Science
Must-Reading Tree
Example
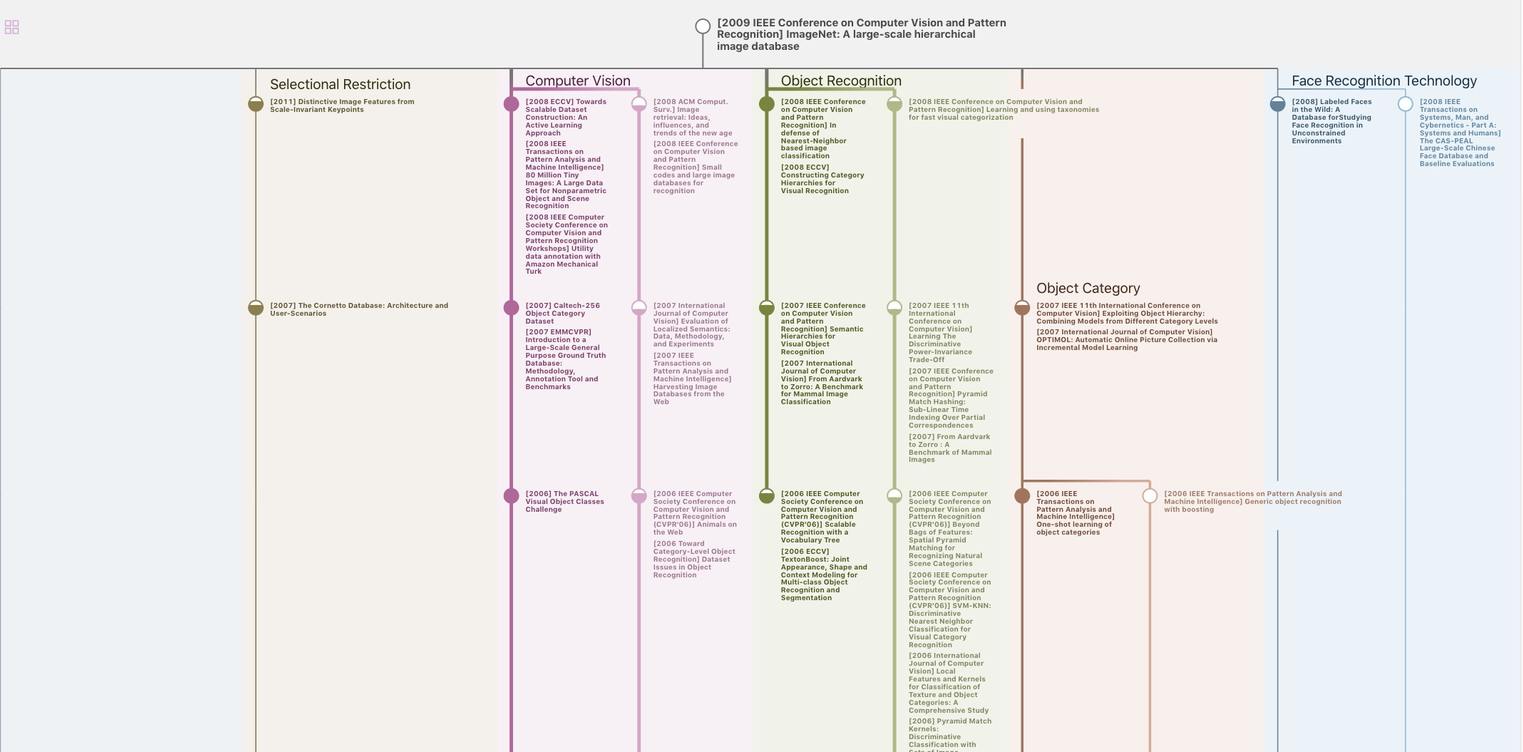
Generate MRT to find the research sequence of this paper
Related Papers
2011
被引用377 | 浏览
Data Disclaimer
The page data are from open Internet sources, cooperative publishers and automatic analysis results through AI technology. We do not make any commitments and guarantees for the validity, accuracy, correctness, reliability, completeness and timeliness of the page data. If you have any questions, please contact us by email: report@aminer.cn
Chat Paper
去 AI 文献库 对话