Control-Oriented Modeling of a Soft Manipulator Using the Learning-Based Koopman Operator.
2023 29th International Conference on Mechatronics and Machine Vision in Practice (M2VIP)(2023)
摘要
Modeling the dynamics of soft manipulators remains challenging owing to their intrinsic compliance and nonlinear deformation. The Koopman operator theory provides a way to construct control-oriented linear models for soft robots. However, the modeling accuracy of the Koopman operator heavily depends on the choices of the eigenfunctions. In this paper, we propose a data-driven modeling framework for the soft manipulator using the learning-based Koopman operator (LKO). Distinct from the classical Koopman operator, the LKO leverages a neural network to discover the representations of the Koopman operator and the eigenfunctions. In this way, the LKO avoids the manual selection of eigenfunctions, enabling the identification of linear models with higher accuracy than that of the classical Koopman operator. The LKO was examined to predict the tip position and the configuration of a customized soft manipulator. Moreover, the LKO was compared with three conventional data-driven approaches, including the classical Koopman operator, feedforward neural network, and recurrent neural network. The results indicate that the modeling accuracy of the LKO was improved by over 60% compared with that of the other three conventional data-driven approaches. With the linear representation and high accuracy, the LKO has great potential to improve the model-based control performance of soft robots.
更多查看译文
关键词
Soft manipulator,data-driven modeling,learning-based Koopman operator
AI 理解论文
溯源树
样例
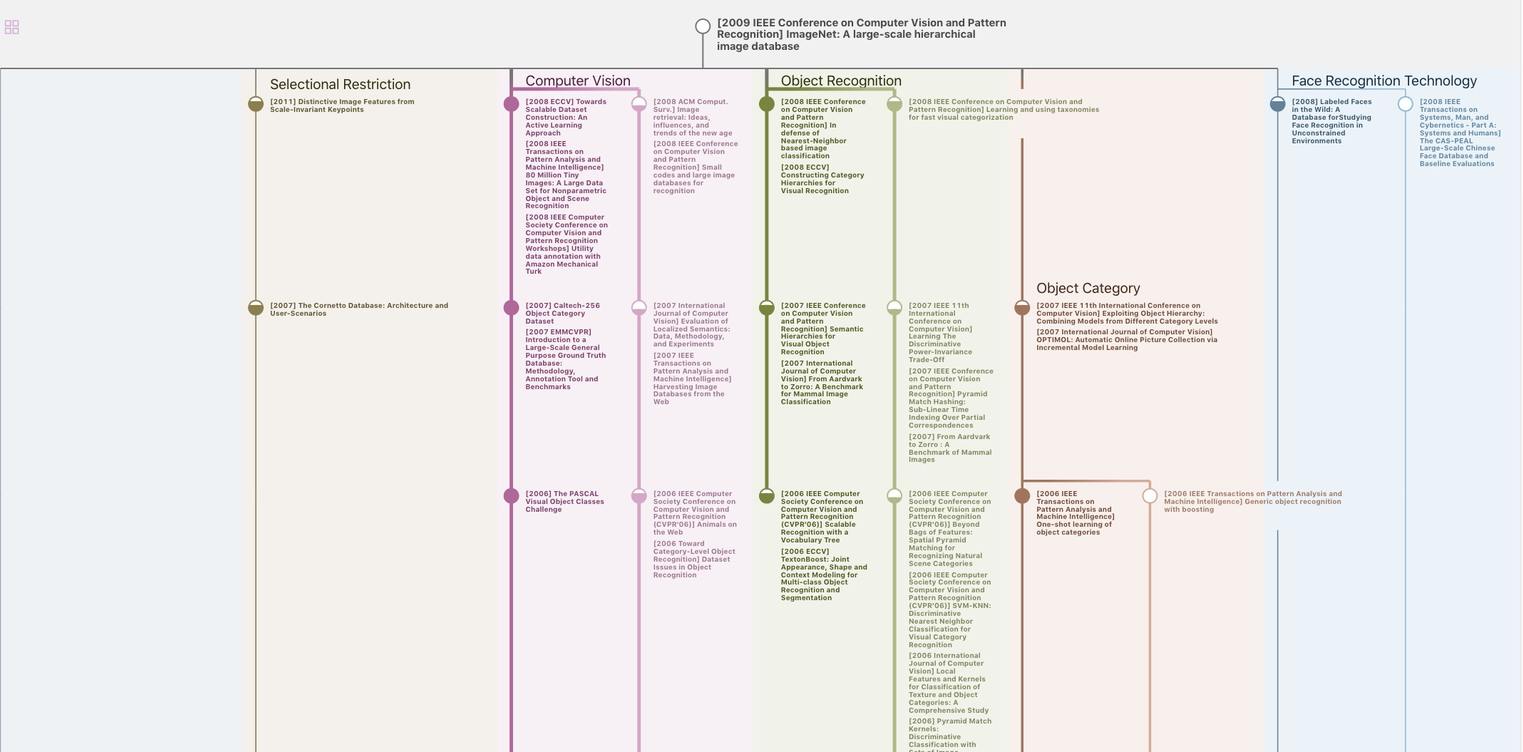
生成溯源树,研究论文发展脉络
Chat Paper
正在生成论文摘要