G-NAS: Generalizable Neural Architecture Search for Single Domain Generalization Object Detection
Computing Research Repository (CoRR)(2024)
Shanghai Jiao Tong Univ | Huawei Noahs Ark Lab
Abstract
In this paper, we focus on a realistic yet challenging task, Single DomainGeneralization Object Detection (S-DGOD), where only one source domain's datacan be used for training object detectors, but have to generalize multipledistinct target domains. In S-DGOD, both high-capacity fitting andgeneralization abilities are needed due to the task's complexity.Differentiable Neural Architecture Search (NAS) is known for its high capacityfor complex data fitting and we propose to leverage Differentiable NAS to solveS-DGOD. However, it may confront severe over-fitting issues due to the featureimbalance phenomenon, where parameters optimized by gradient descent are biasedto learn from the easy-to-learn features, which are usually non-causal andspuriously correlated to ground truth labels, such as the features ofbackground in object detection data. Consequently, this leads to seriousperformance degradation, especially in generalizing to unseen target domainswith huge domain gaps between the source domain and target domains. To addressthis issue, we propose the Generalizable loss (G-loss), which is an OoD-awareobjective, preventing NAS from over-fitting by using gradient descent tooptimize parameters not only on a subset of easy-to-learn features but also theremaining predictive features for generalization, and the overall framework isnamed G-NAS. Experimental results on the S-DGOD urban-scene datasetsdemonstrate that the proposed G-NAS achieves SOTA performance compared tobaseline methods. Codes are available at https://github.com/wufan-cse/G-NAS.
MoreTranslated text
Key words
Object Detection
PDF
View via Publisher
AI Read Science
Must-Reading Tree
Example
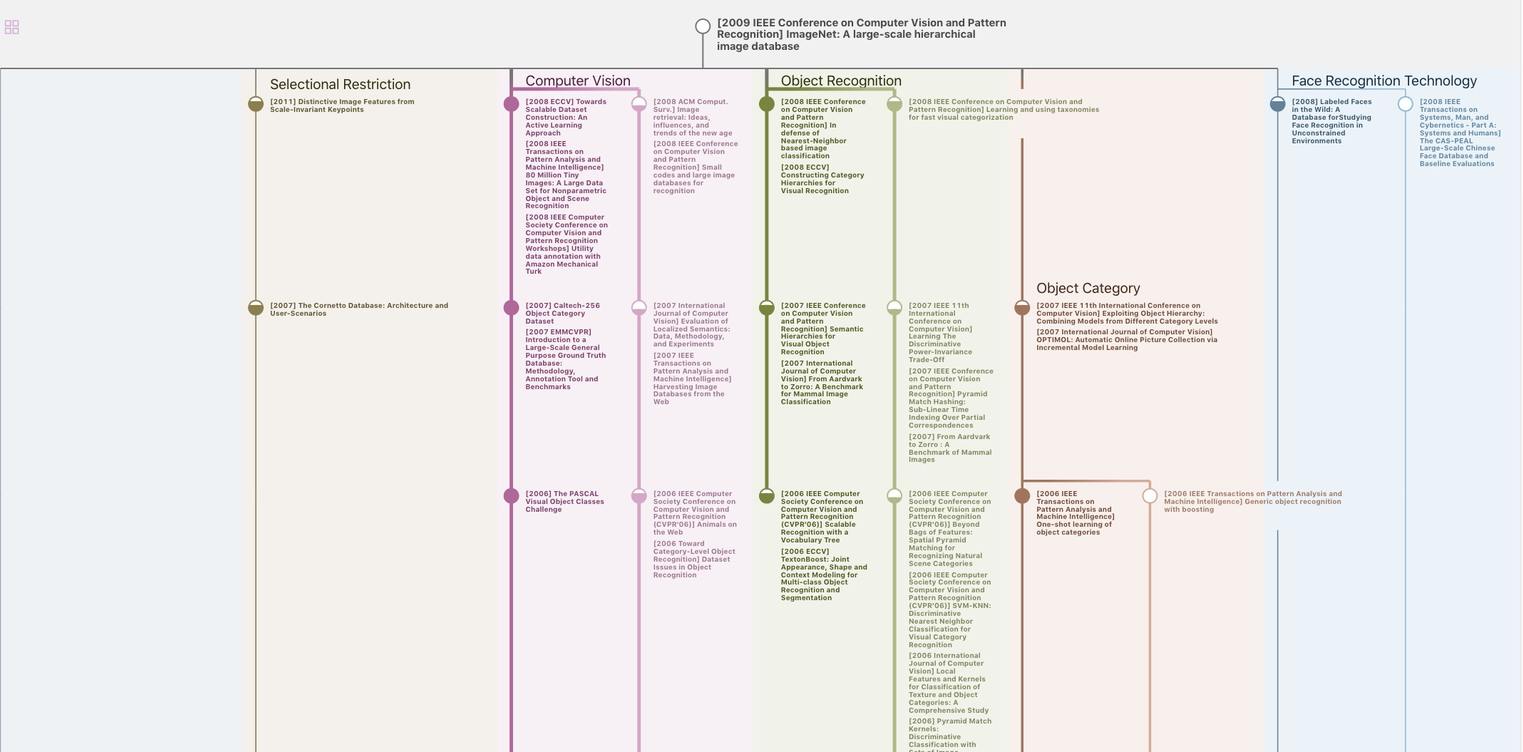
Generate MRT to find the research sequence of this paper
Related Papers
2019
被引用378 | 浏览
2019
被引用253 | 浏览
2021
被引用332 | 浏览
2022
被引用33 | 浏览
2024
被引用5 | 浏览
2024
被引用8 | 浏览
Data Disclaimer
The page data are from open Internet sources, cooperative publishers and automatic analysis results through AI technology. We do not make any commitments and guarantees for the validity, accuracy, correctness, reliability, completeness and timeliness of the page data. If you have any questions, please contact us by email: report@aminer.cn
Chat Paper
去 AI 文献库 对话