Particle Denoising Diffusion Sampler
ICML 2024(2024)
University of Oxford | Department of Statistics | Oxford | Oxford University
Abstract
Denoising diffusion models have become ubiquitous for generative modeling.The core idea is to transport the data distribution to a Gaussian by using adiffusion. Approximate samples from the data distribution are then obtained byestimating the time-reversal of this diffusion using score matching ideas. Wefollow here a similar strategy to sample from unnormalized probabilitydensities and compute their normalizing constants. However, the time-reverseddiffusion is here simulated by using an original iterative particle schemerelying on a novel score matching loss. Contrary to standard denoisingdiffusion models, the resulting Particle Denoising Diffusion Sampler (PDDS)provides asymptotically consistent estimates under mild assumptions. Wedemonstrate PDDS on multimodal and high dimensional sampling tasks.
MoreTranslated text
Key words
Immersed Boundary Method
PDF
View via Publisher
AI Read Science
Must-Reading Tree
Example
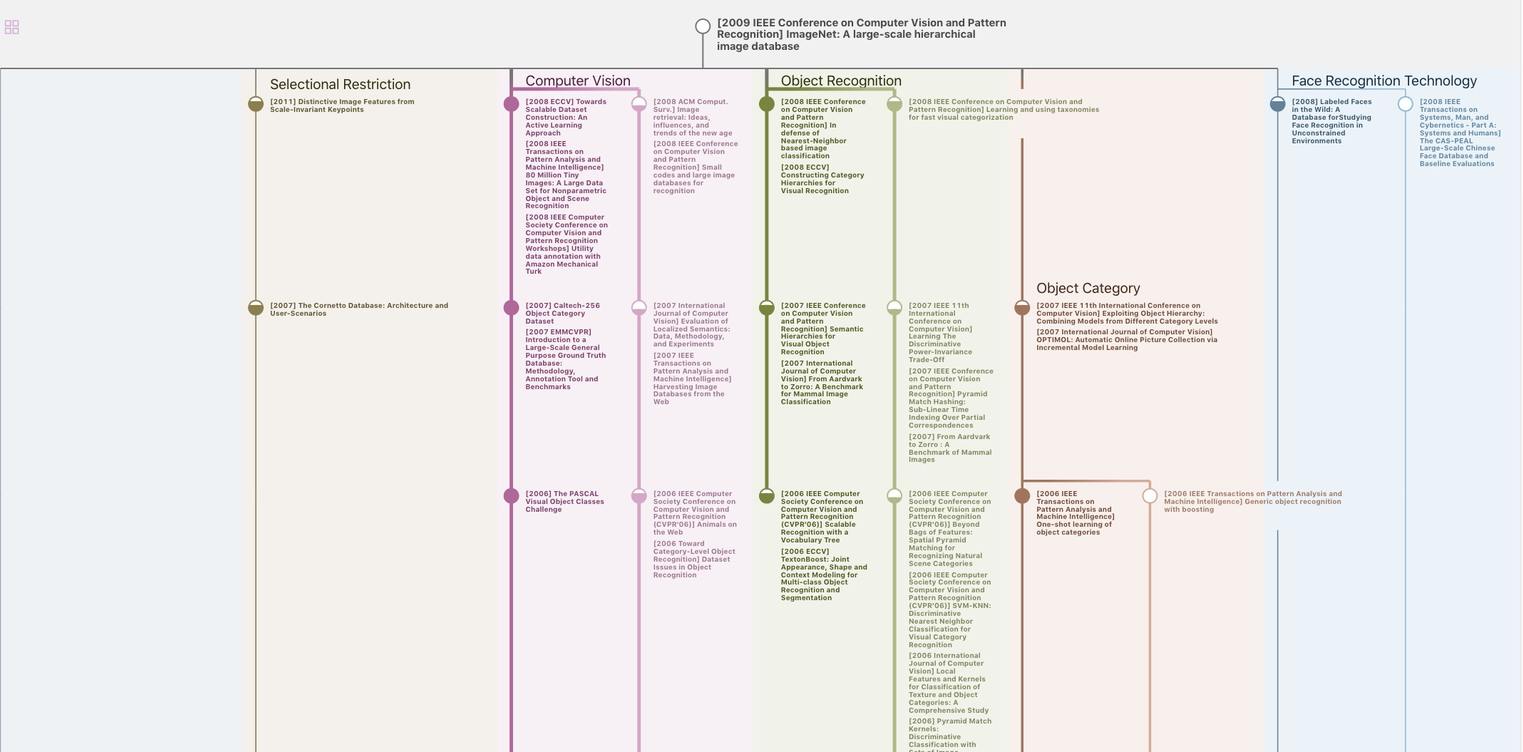
Generate MRT to find the research sequence of this paper
Related Papers
Data Disclaimer
The page data are from open Internet sources, cooperative publishers and automatic analysis results through AI technology. We do not make any commitments and guarantees for the validity, accuracy, correctness, reliability, completeness and timeliness of the page data. If you have any questions, please contact us by email: report@aminer.cn
Chat Paper
Summary is being generated by the instructions you defined