Semi-supervised Bayesian integration of multiple spatial proteomics datasets
biorxiv(2024)
摘要
The subcellular localisation of proteins is a key determinant of their function. High-throughput analyses of these localisations can be performed using mass spectrometry-based spatial proteomics, which enables us to examine the localisation and relocalisation of proteins. Furthermore, complementary data sources can provide additional sources of functional or localisation information. Examples include protein annotations and other high-throughput ‘omic assays. Integrating these modalities can provide new insights as well as additional confidence in results, but existing approaches for integrative analyses of spatial proteomics datasets are limited in the types of data they can integrate and do not quantify uncertainty. Here we propose a semi-supervised Bayesian approach to integrate spatial proteomics datasets with other data sources, to improve the inference of protein sub-cellular localisation. We demonstrate our approach outperforms other transfer-learning methods and has greater flexibility in the data it can model. To demonstrate the flexibility of our approach, we apply our method to integrate spatial proteomics data generated for the parasite Toxoplasma gondii with time-course gene expression data generated over its cell cycle. Our findings suggest that proteins linked to invasion organelles are associated with expression programs that peak at the end of the first cell-cycle. Furthermore, this integrative analysis divides the dense granule proteins into heterogeneous populations suggestive of potentially different functions. Our method is disseminated via the mdir R package available on the lead author’s Github.
Author summary Proteins are located in subcellular environments to ensure that they are near their interaction partners and occur in the correct biochemical environment to function. Where a protein is located can be determined from a number of data sources. To integrate diverse datasets together we develop an integrative Bayesian model to combine the information from several datasets in a principled manner. We learn how similar the dataset are as part of the modelling process and demonstrate the benefits of integrating mass-spectrometry based spatial proteomics data with timecourse gene-expression datasets.
### Competing Interest Statement
CW receives funding from MSD and GSK and is a part-time employee of GSK. These companies had no involvement in the work presented here.
更多查看译文
AI 理解论文
溯源树
样例
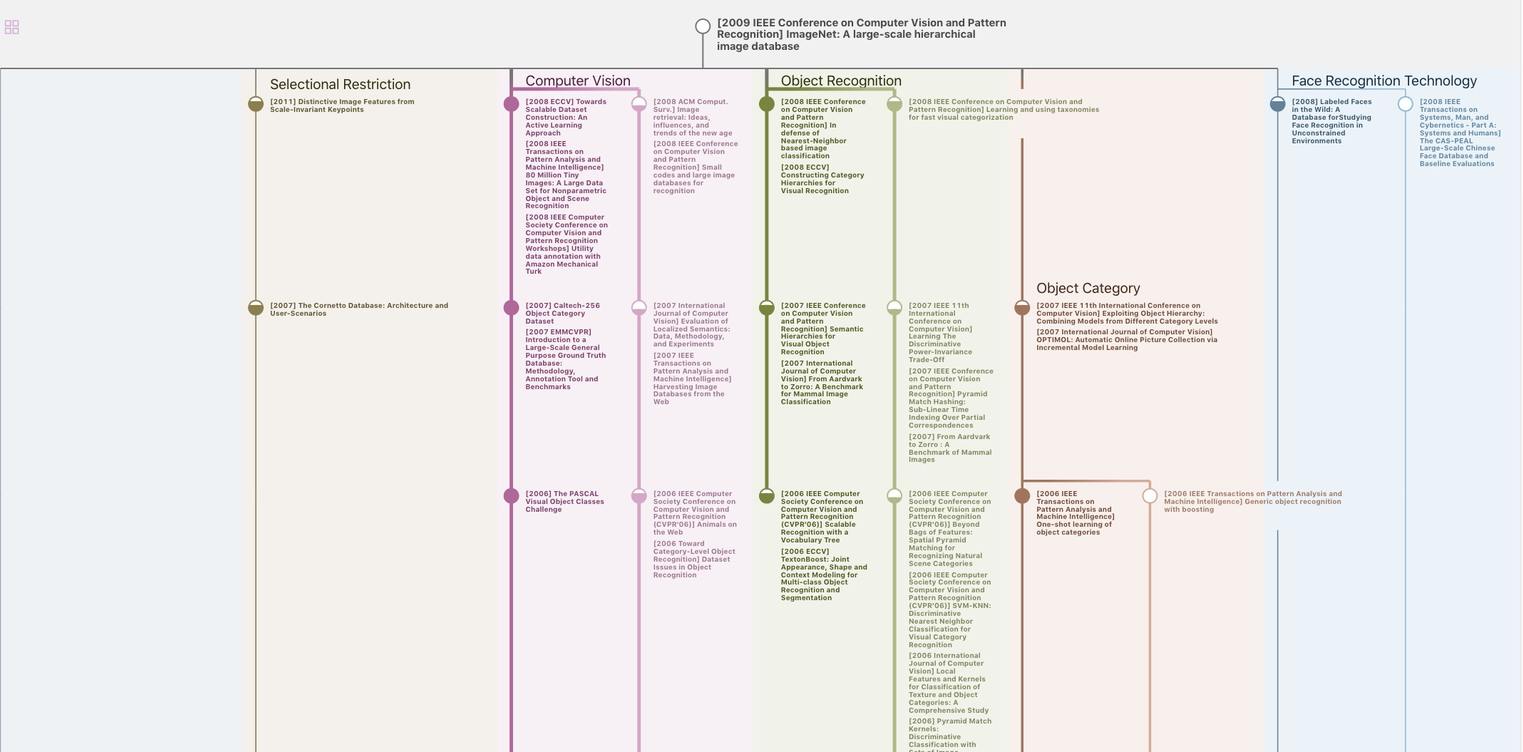
生成溯源树,研究论文发展脉络
Chat Paper
正在生成论文摘要