Segmented Curved-Voxel Occupancy Descriptor for Dynamic-Aware LiDAR Odometry and Mapping
IEEE TRANSACTIONS ON GEOSCIENCE AND REMOTE SENSING(2024)
摘要
Simultaneous localization and mapping (SLAM) employing 3-D light detection and ranging (LiDAR) data constitutes an indispensable perception technology for geospatial sensing of the surrounding environments. Nevertheless, the existence of dynamic objects can substantially impair sensing performance. This article proposes a novel egocentric descriptor named segmented curved-voxel occupancy descriptor (SCV-OD), which serves as the backbone for constructing a dynamic-aware and LiDAR-only SLAM in a tight-coupled and consistent manner. Assisted with LiDAR intensity and geometric features, the object segmentation module clusters curved voxels into objects and recognizes potential dynamic (PD) objects as prior knowledge. Then, in the object tracking module, PD objects are tracked through curved-voxel overlay, and high dynamic (HD) objects are removed according to the object overlap ratio. The aforementioned two modules are closely coupled to mutually compensate for accuracy loss by sharing the same SCV-OD. Finally, the voxelized generalized iterative closest point (VGICP)-based LiDAR mapping module optimizes LiDAR poses by considering dynamic objects and results in a global static instance map. We validated the proposed method on the public dataset (KITTI) and our custom dataset. The evaluation results illustrate that our method outperforms the state-of-the-art (SOTA) LiDAR-only methods in pose estimation and dynamic removal.
更多查看译文
关键词
Location awareness,Laser radar,Simultaneous localization and mapping,Object segmentation,Distance measurement,Sensors,Object tracking,Dynamic removal,geospatial sensing,light detection and ranging (LiDAR) data,pose estimation,simultaneous localization and mapping (SLAM)
AI 理解论文
溯源树
样例
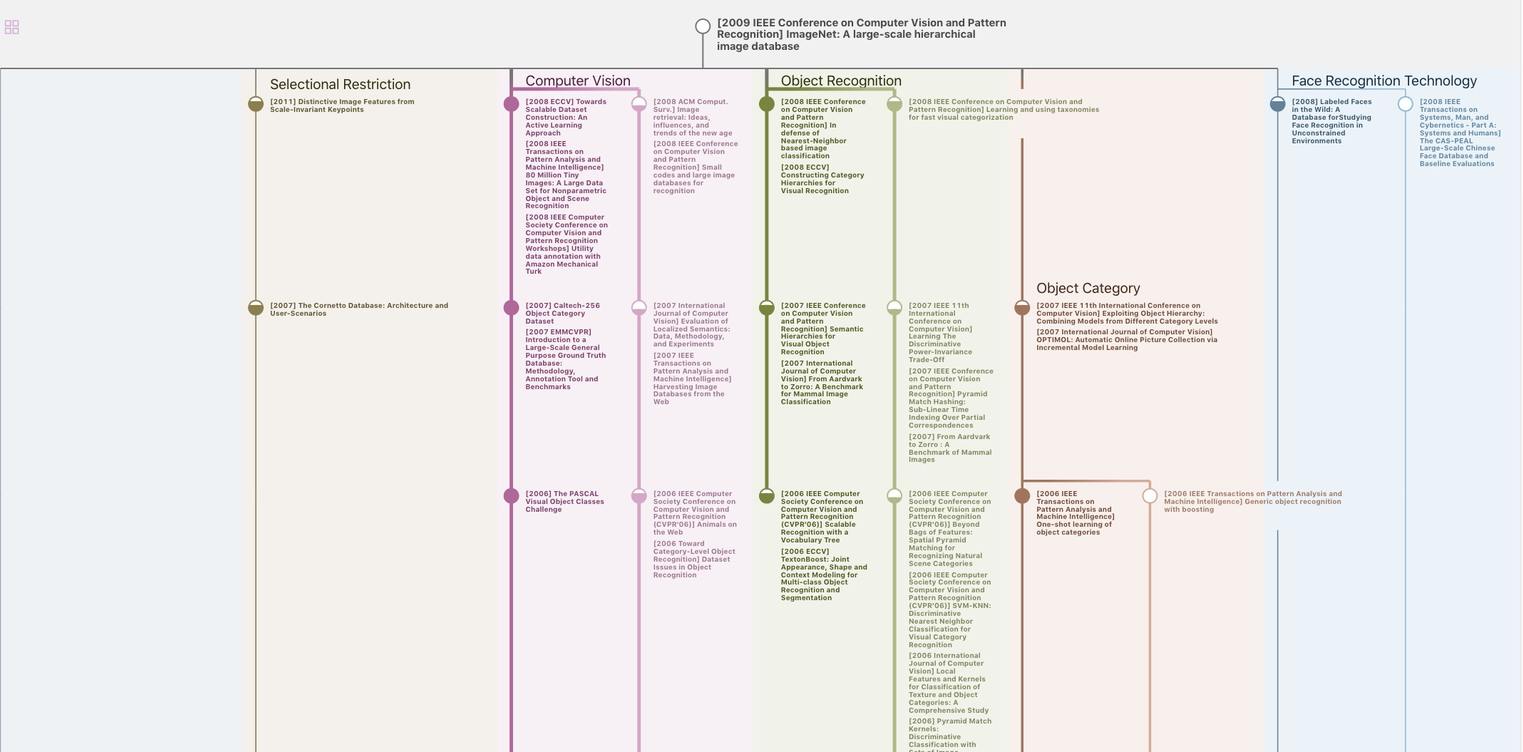
生成溯源树,研究论文发展脉络
Chat Paper
正在生成论文摘要