Sample Complexity for Distributionally Robust Learning under 2-divergence
JOURNAL OF MACHINE LEARNING RESEARCH(2023)
摘要
This paper investigates the sample complexity of learning a distributionally robust predictor under a particular distributional shift based on chi(2)-divergence, which is well known for its computational feasibility and statistical properties. We demonstrate that any hypothesis class H with finite VC dimension is distributionally robustly learnable. Moreover, we show that when the perturbation size is smaller than a constant, finite VC dimension is also necessary for distributionally robust learning by deriving a lower bound of sample complexity in terms of VC dimension.
更多查看译文
关键词
distributionally robustness,PAC learning,sample complexity,chi(2)-divergence
AI 理解论文
溯源树
样例
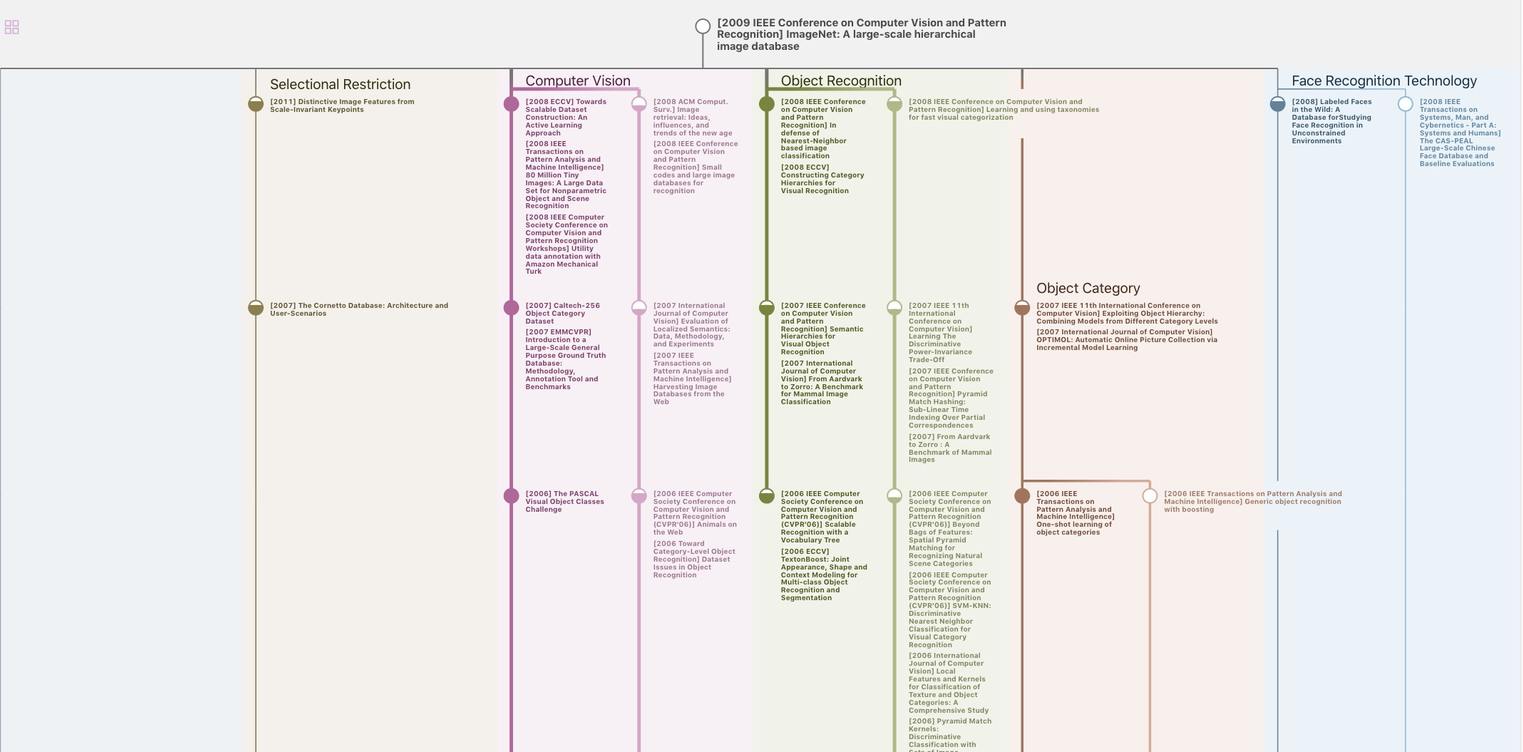
生成溯源树,研究论文发展脉络
Chat Paper
正在生成论文摘要